Detecting Emotion Primitives from Speech and their use in discerning Categorical Emotions
2020 IEEE INTERNATIONAL CONFERENCE ON ACOUSTICS, SPEECH, AND SIGNAL PROCESSING(2020)
摘要
Emotion plays an essential role in human-to-human communication, enabling us to convey feelings such as happiness, frustration, and sincerity. While modern speech technologies rely heavily on speech recognition and natural language understanding for speech content understanding, the investigation of vocal expression is increasingly gaining attention. Key considerations for building robust emotion models include characterizing and improving the extent to which a model, given its training data distribution, is able to generalize to unseen data conditions. This work investigated a long-shot-term memory (LSTM) network and a time convolution - LSTM (TC-LSTM) to detect primitive emotion attributes such as valence, arousal, and dominance, from speech. It was observed that training with multiple datasets and using robust features improved the concordance correlation coefficient (CCC) for valence, by 30\% with respect to the baseline system. Additionally, this work investigated how emotion primitives can be used to detect categorical emotions such as happiness, disgust, contempt, anger, and surprise from neutral speech, and results indicated that arousal, followed by dominance was a better detector of such emotions.
更多查看译文
关键词
Vocal expression, intent, paralinguistic features, long-short-term memory networks, emotion
AI 理解论文
溯源树
样例
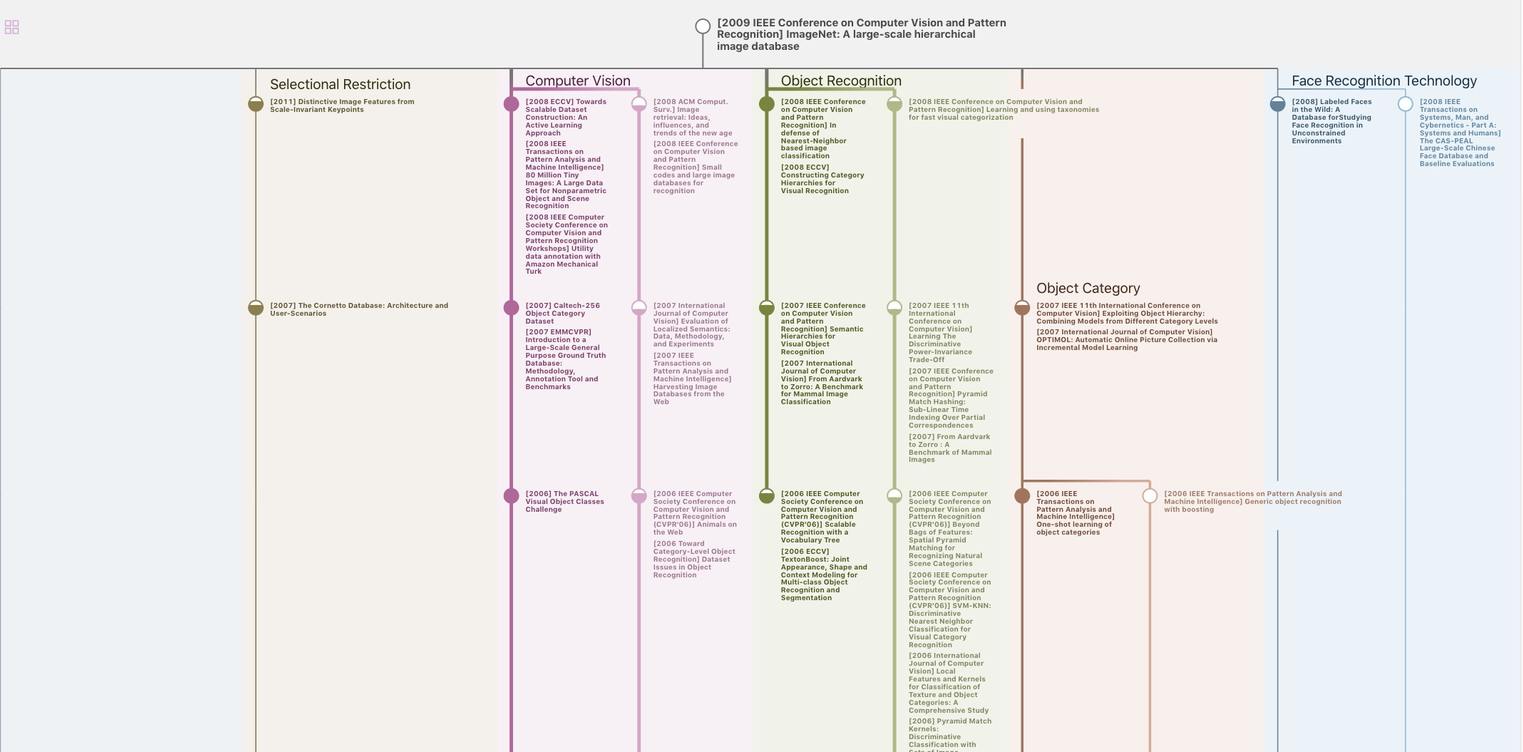
生成溯源树,研究论文发展脉络
Chat Paper
正在生成论文摘要