MTEX-CNN: Multivariate Time Series EXplanations for Predictions with Convolutional Neural Networks
2019 19TH IEEE INTERNATIONAL CONFERENCE ON DATA MINING (ICDM 2019)(2019)
摘要
In this work we present MTEX-CNN, a novel explainable convolutional neural network architecture which can not only be used for making predictions based on multivariate time series data, but also for explaining these predictions. The network architecture consists of two stages and utilizes particular kernel sizes. This allows us to apply gradient based methods for generating saliency maps for both the time dimension and the features. The first stage of the architecture explains which features are most significant to the predictions, while the second stage explains which time segments are the most significant. We validate our approach on two use cases, namely to predict rare server outages in the wild, as well as the average energy production of photovoltaic power plants based on a benchmark data set. We show that our explanations shed light over what the model has learned. We validate this by retraining the network using the most significant features extracted from the explanations and retaining similar performance to training with the full set of features.
更多查看译文
关键词
Convolutional Neural Network, Explainable Machine Learning, Multivariate Time Series, Deep Learning
AI 理解论文
溯源树
样例
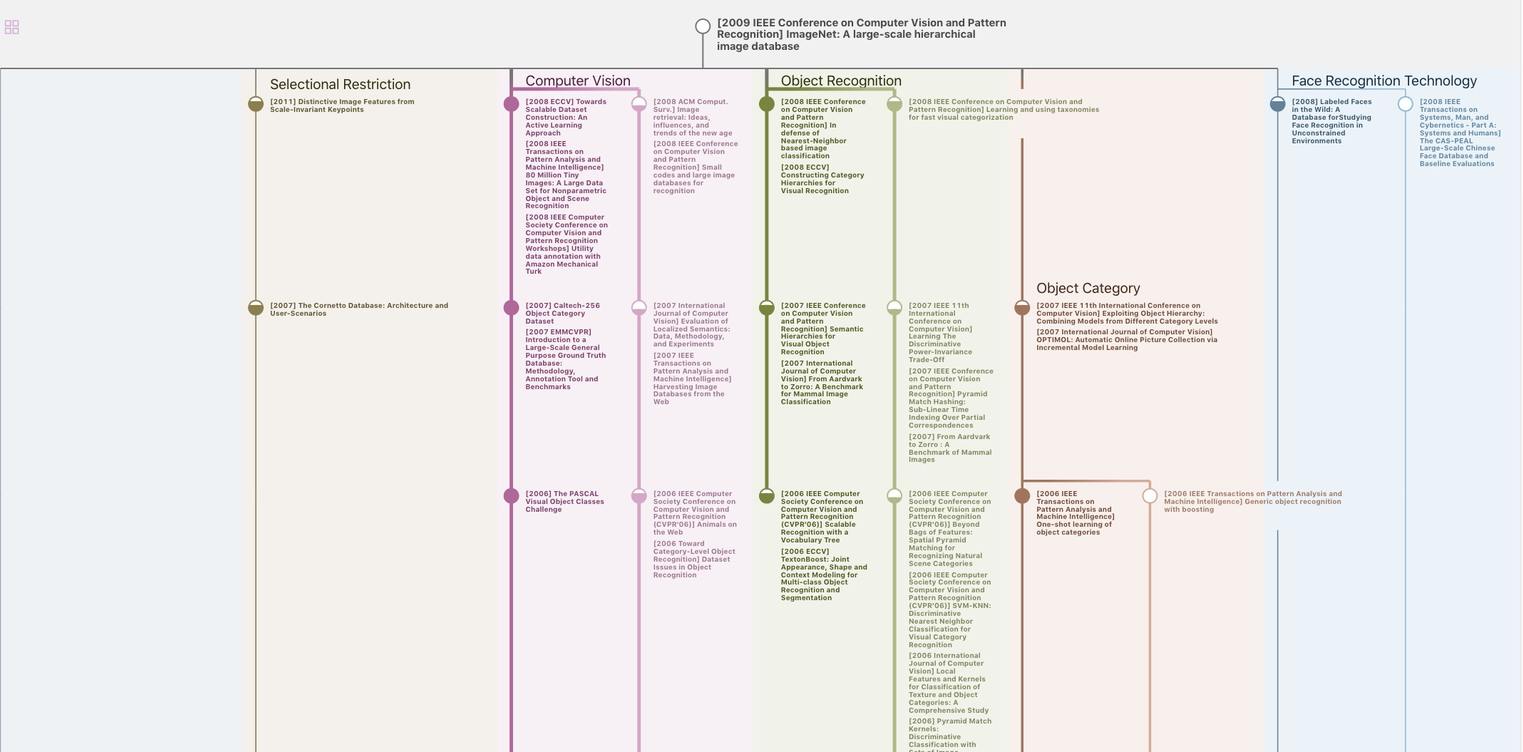
生成溯源树,研究论文发展脉络
Chat Paper
正在生成论文摘要