Beyond Geo-First Law: Learning Spatial Representations via Integrated Autocorrelations and Complementarity
2019 IEEE International Conference on Data Mining (ICDM)(2019)
摘要
Spatial representation learning (SRL) is to automatically learn feature representations that characterize spatial entities. In this paper, we study the problem of improving spatial representation learning using spatial structure knowledge. We consider two types of structure knowledge: (1) spatial autocorrelations refer to the pattern that similar spatial entities are more likely to share similar roles and configurations. (2) spatial complementarity refers to the effect that the role of a spatial entity can be complemented and augmented by other different yet compatible spatial entities. Along this line, we develop a step-by-step SRL framework to integrate spatial autocorrelations and complementarity. This framework includes four testable steps. First, we construct multi-view POI-POI(Point of Interest) graphs to characterize the static and dynamic patterns of each spatial region. We then use the graphs as inputs to train an adversarial autoencoder (AAE) that can preserve the spatial autocorrelation property and learn representations of spatial entities. Later, with the learned representations extracted from AAE inputs, a Graph Convolutional Network (GCN) is trained in an unsupervised fashion in order to overcome label sparsity and capture the spatial complementarity effect. In this way, we significantly improve the quality of spatial representations. In addition, we apply the proposed method to characterize residential communities for predicting real estate prices. Finally, we present intensive experimental results with real-world real estate data to demonstrate the proposed method effectiveness.
更多查看译文
关键词
Spatial Representation,Autocorrelation,Complementarity,AAE,GCN
AI 理解论文
溯源树
样例
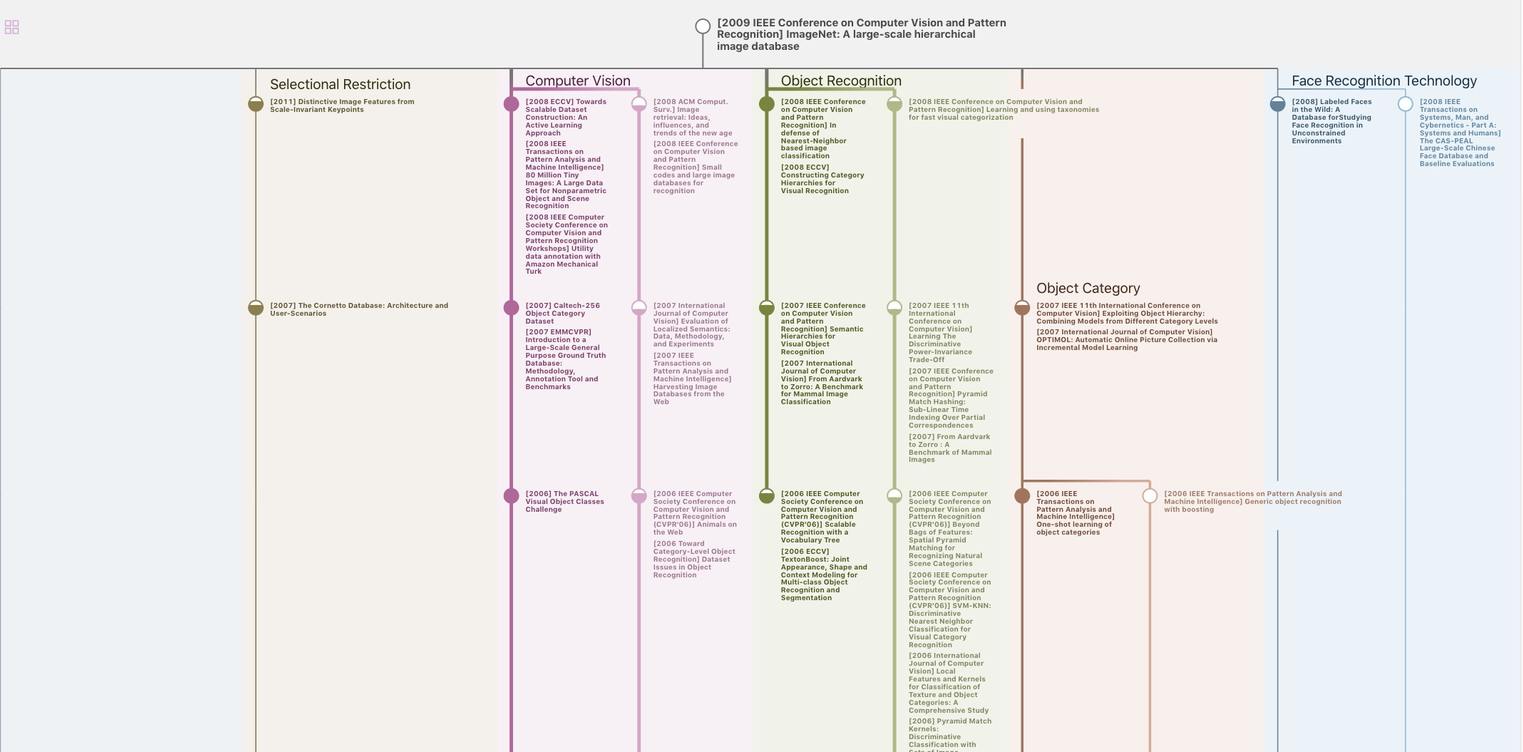
生成溯源树,研究论文发展脉络
Chat Paper
正在生成论文摘要