Triple-Shapelet Networks For Time Series Classification
2019 19TH IEEE INTERNATIONAL CONFERENCE ON DATA MINING (ICDM 2019)(2019)
摘要
Shapelets are discriminative subsequences for time series classification (TSC). Although shapelet-based methods have achieved good performance and interpretability, they still have two issues that can be improved. First, previous methods only assess a shapelet by how accurately it can classify all the samples. However, for multi-class imbalanced classification tasks, these methods will ignore the shapelets that can distinguish minority class from other classes and will tend to use the shapelets that are useful for discriminating the majority classes. Second, the shapelets are fixed after the training phase and cannot adapt to time series with deformations, which will lead to poor matches to the shapelets. In this paper, we propose a novel end-to-end shapelet learning model called Triple Shapelet Networks (TSNs) to extract multi-level feature representations. Specifically, TSN learns the most discriminative shapelets by gradient descent similar to previous methods. In addition, it learns category-specific shapelets for each class by using auxiliary binary classifiers. Finally, it uses a shapelet generator to produce sample-specific shapelets conditioned on subsequences of the input time series. The addition of category-level and sample-level shapelets to the standard model improves the performance. Experiments conducted on extensive time series data sets show that TSN is state-of-the-art compared to existing shapelet-based methods, and the visualization analysis also shows its effectiveness.
更多查看译文
关键词
Shapelet transformation, time series classification, temporal feature learning
AI 理解论文
溯源树
样例
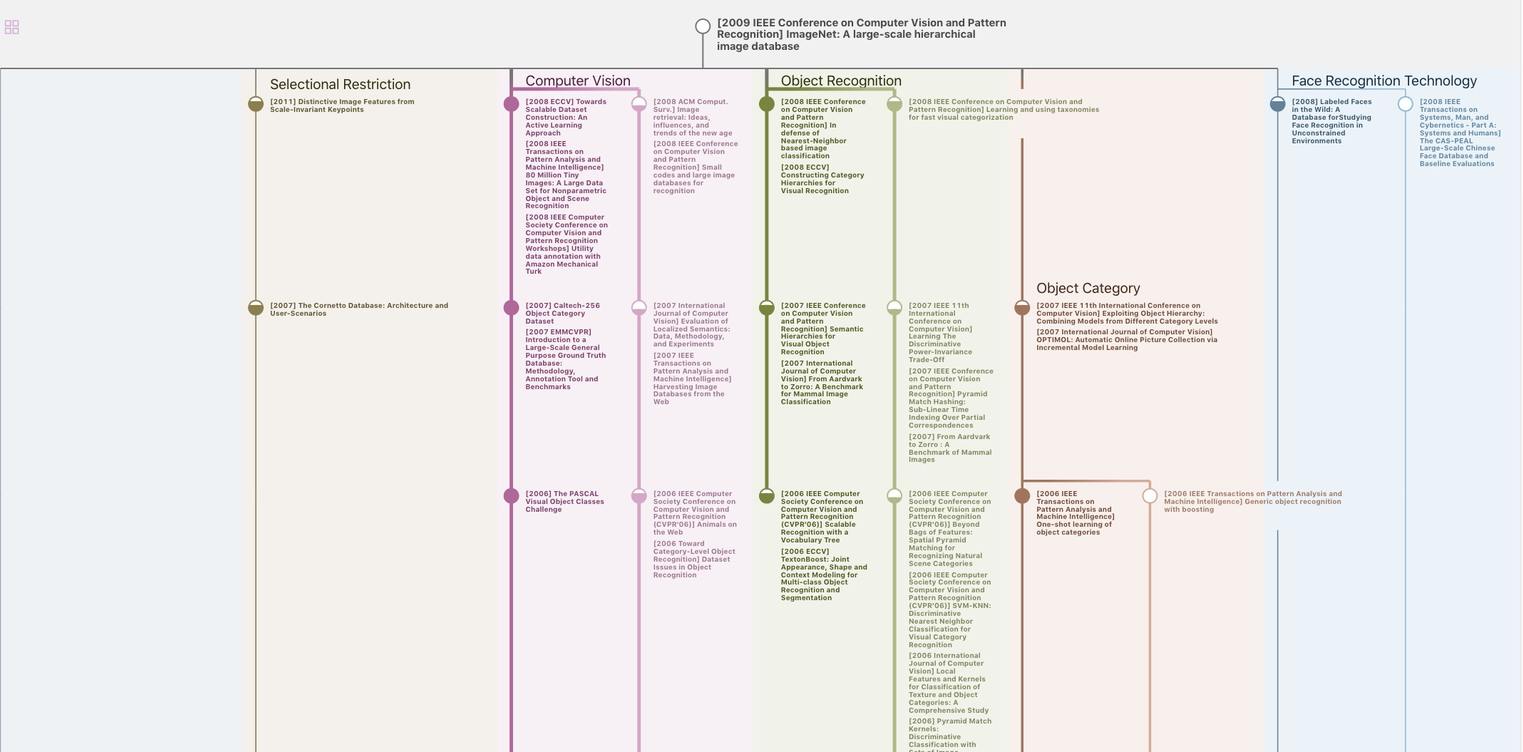
生成溯源树,研究论文发展脉络
Chat Paper
正在生成论文摘要