Collaborative Label Correction Via Entropy Thresholding
2019 19TH IEEE INTERNATIONAL CONFERENCE ON DATA MINING (ICDM 2019)(2019)
摘要
Deep neural networks (DNNs) have the capacity to fit extremely noisy labels nonetheless they tend to learn data with clean labels first and then memorize those with noisy labels. We examine this behavior in light of the Shannon entropy of the predictions and demonstrate the low entropy predictions determined by a given threshold are much more reliable as the supervision than the original noisy labels. It also shows the advantage in maintaining more training samples than previous methods. Then, we power this entropy criterion with the Collaborative Label Correction (CLC) framework to further avoid undesired local minimums of the single network. A range of experiments have been conducted on multiple benchmarks with both synthetic and real-world settings. Extensive results indicate that our CLC outperforms several state-of-the-art methods.
更多查看译文
关键词
deep learning, noisy supervision, entropy thresholding, label correction, sample selection
AI 理解论文
溯源树
样例
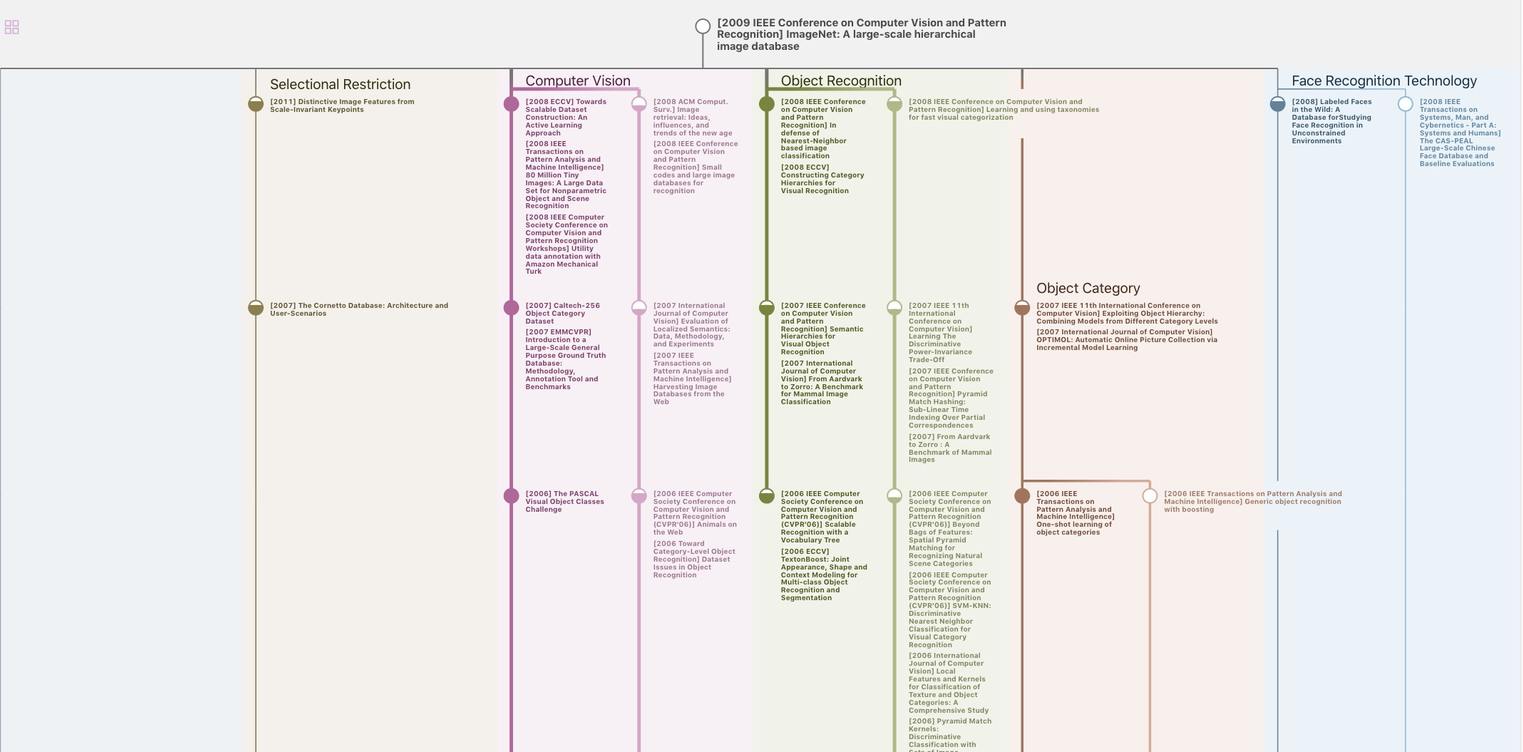
生成溯源树,研究论文发展脉络
Chat Paper
正在生成论文摘要