Fall Detection with Supervised Machine Learning using Wearable Sensors
2019 IEEE 17th International Conference on Industrial Informatics (INDIN)(2019)
摘要
Unintentional falls can cause severe injuries to a person, and even death, especially when no immediate assistance is provided. The aim of Fall Detection Systems (FDSs) is to detect the occurrence of a fall and to automatically and promptly request the necessary assistance. This work proposes a FDS based on wearable sensors - i.e., accelerometers and gyroscopes - and Machine Learning (ML), for sensor signal processing and detection. The process extracts a number of features on portions of the signal and classifies them as falls or regular daily activities. The classifier is a Support Vector Machine (SVM) that is trained using a manually labelled dataset, where human activities are distinguished between falls and regular activities. The method is assessed on the publicly available SisFall dataset, with extended annotation, and compared with the results obtained in the literature for the same dataset; the proposed method largely outperforms the original analysis technique proposed for the SisFall dataset, with an F1 score higher than 97% and a recall higher than 99.7%.
更多查看译文
关键词
Fall Detection,Machine Learning,Support Vector Machines,Dataset,Wearable Sensors,Healthcare
AI 理解论文
溯源树
样例
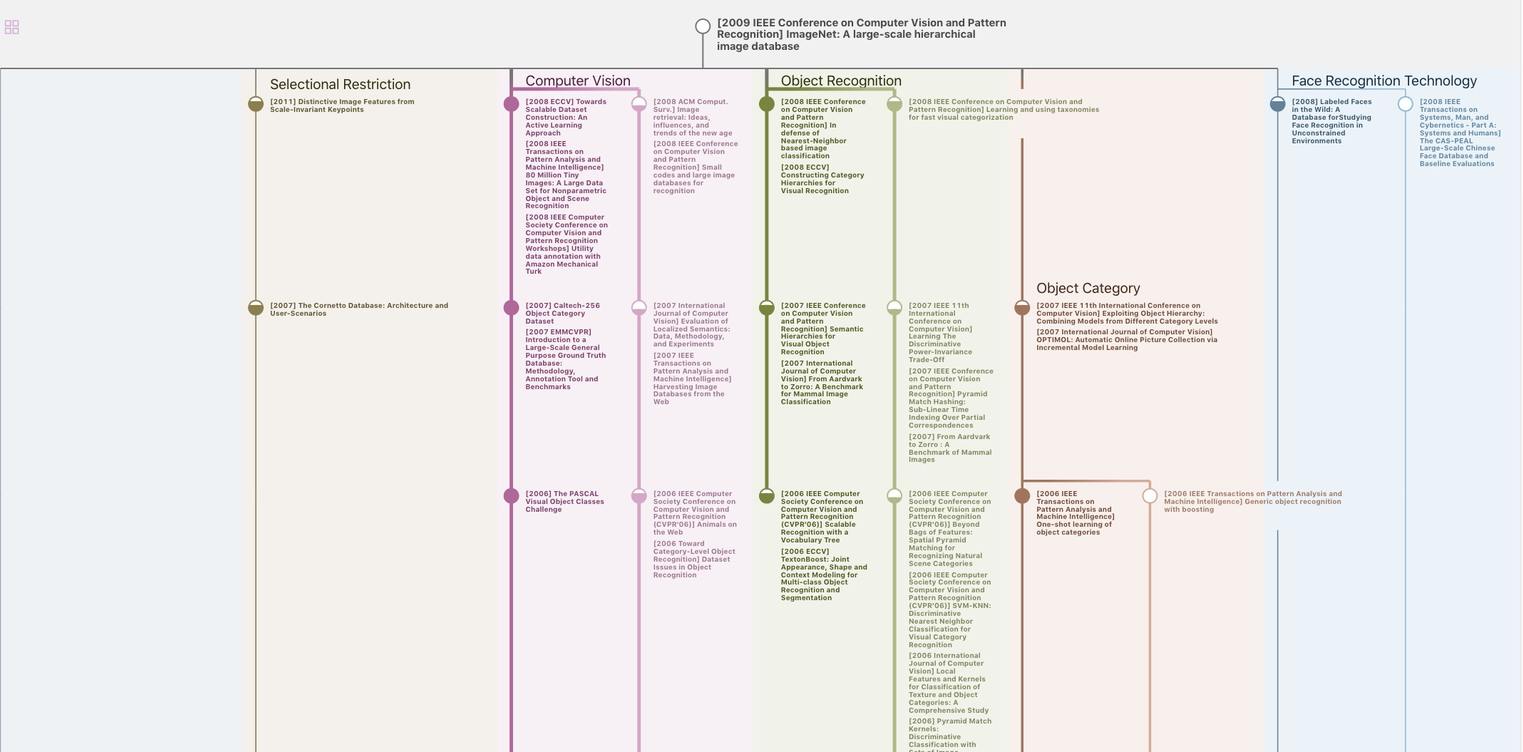
生成溯源树,研究论文发展脉络
Chat Paper
正在生成论文摘要