Detecting Sequential Human Mental Workload Using U-Net With Continuity-Aware Loss Applied To Streamed Physiological Signals
2019 IEEE 17TH INTERNATIONAL CONFERENCE ON INDUSTRIAL INFORMATICS (INDIN)(2019)
摘要
Human mental workload perception is an important problem with multiple applications in the areas of industry, transportation, military and medical contexts. In this paper, we present a deep learning model developed for detecting sequential driver mental workload using physiological signals. We modify a fully convolutional U-Net in order to learn from multiple temporal physiological signals as inputs. We also demonstrate that the element-wise cross-entropy loss function, which has been adopted by many other deep learning architectures for classification or segmentation purposes, is not sufficient for the sequential workload segmentation because it treats each data point as an independent classification object while ignoring the neighboring relation between the points. We introduce a new loss function that is sensitive to local continuity property in workload sequence. The proposed method is evaluated on two sets of data collected by wearable sensors to record multiple physiological signals. The effectiveness of an introduced scalar used to control the influence of the continuity-aware loss is investigated. Our experiments show that the proposed U-Net is effective in detecting sequential human mental workload. Furthermore, when the proposed continuity-aware loss is combined with the element-wise binary cross-entropy loss with the properly selected controlling scalar value, the quality of the predicted mental workload segments is significantly improved.
更多查看译文
关键词
continuity-aware loss,sequential human mental workload,human mental workload perception,military contexts,medical contexts,deep learning model,sequential driver mental workload,multiple temporal physiological signals,element-wise cross-entropy loss function,deep learning architectures,classification,sequential workload segmentation,local continuity property,workload sequence,multiple physiological signals,fully convolutional U-net,mental workload segments,streamed physiological signals,wearable sensors
AI 理解论文
溯源树
样例
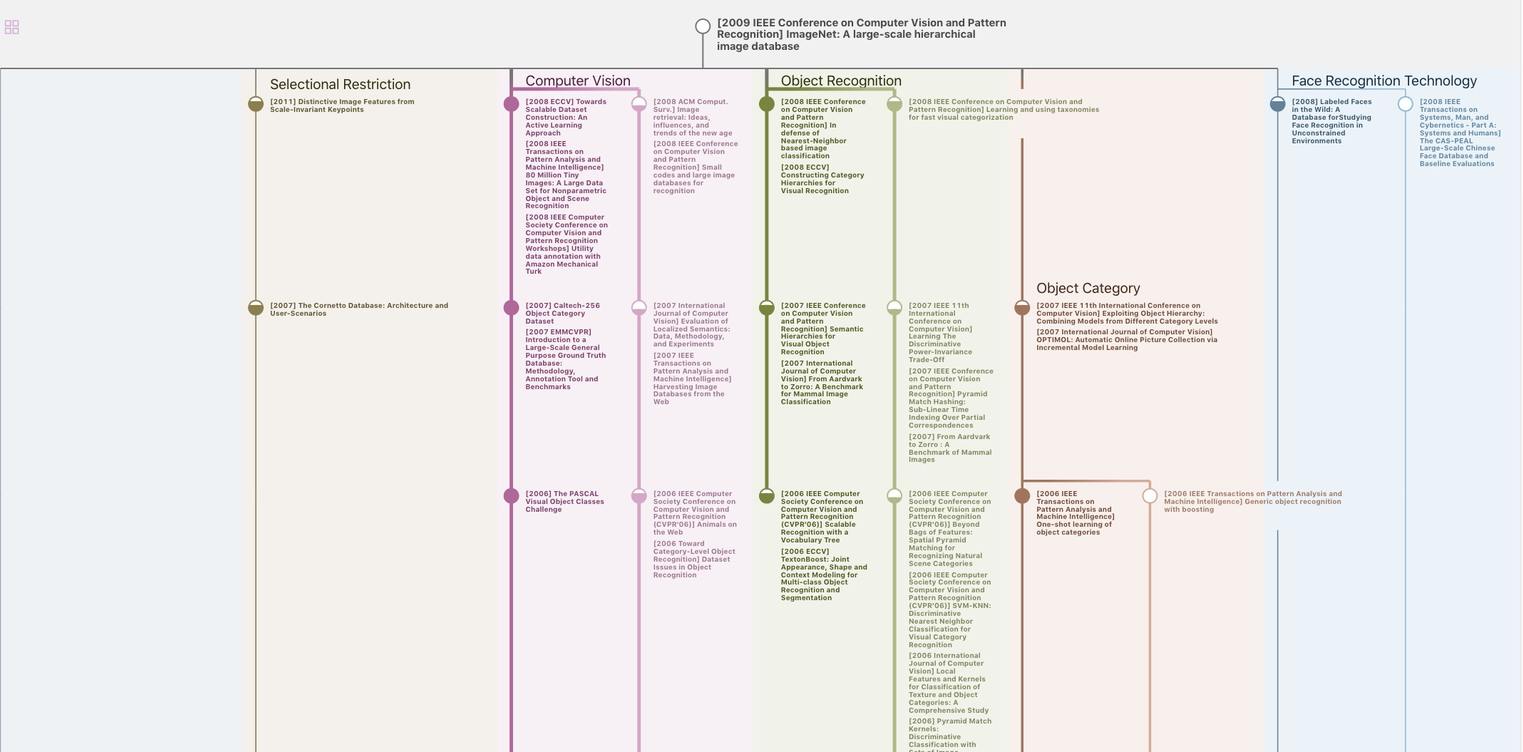
生成溯源树,研究论文发展脉络
Chat Paper
正在生成论文摘要