A Fast Radio Map Construction Method Merging Self-Adaptive Local Linear Embedding (LLE) and Graph-Based Label Propagation in WLAN Fingerprint Localization Systems.
SENSORS(2020)
摘要
Indoor WLAN fingerprint localization systems have been widely applied due to the simplicity of implementation on various mobile devices, including smartphones. However, collecting received signal strength indication (RSSI) samples for the fingerprint database, named a radio map, is significantly labor-intensive and time-consuming. To solve the problem, this paper proposes a semi-supervised self-adaptive local linear embedding algorithm to build the radio map. First, this method uses the self-adaptive local linear embedding (SLLE) algorithm based on manifold learning to reduce the dimension of the high-dimensional RSSI samples and to extract a neighbor weight matrix. Secondly, a graph-based label propagation (GLP) algorithm is employed to build the radio map by semi-supervised learning from a large number of unlabeled RSSI samples to a few labeled RSSI samples. Finally, we propose a k self-adaptive neighbor weight (kSNW) algorithm, used for radio map construction in this paper, to realize online localization. The results of the experiments conducted in a real indoor environment show that the proposed method reduces the demand for large quantities of labeled samples and achieves good positioning accuracy. With only 25% labeled RSSI samples, our system can obtain positioning accuracy of more than 88%, within 3 m of localization errors.
更多查看译文
关键词
indoor positioning,radio map,LLE,manifold learning,graph-based label propagation
AI 理解论文
溯源树
样例
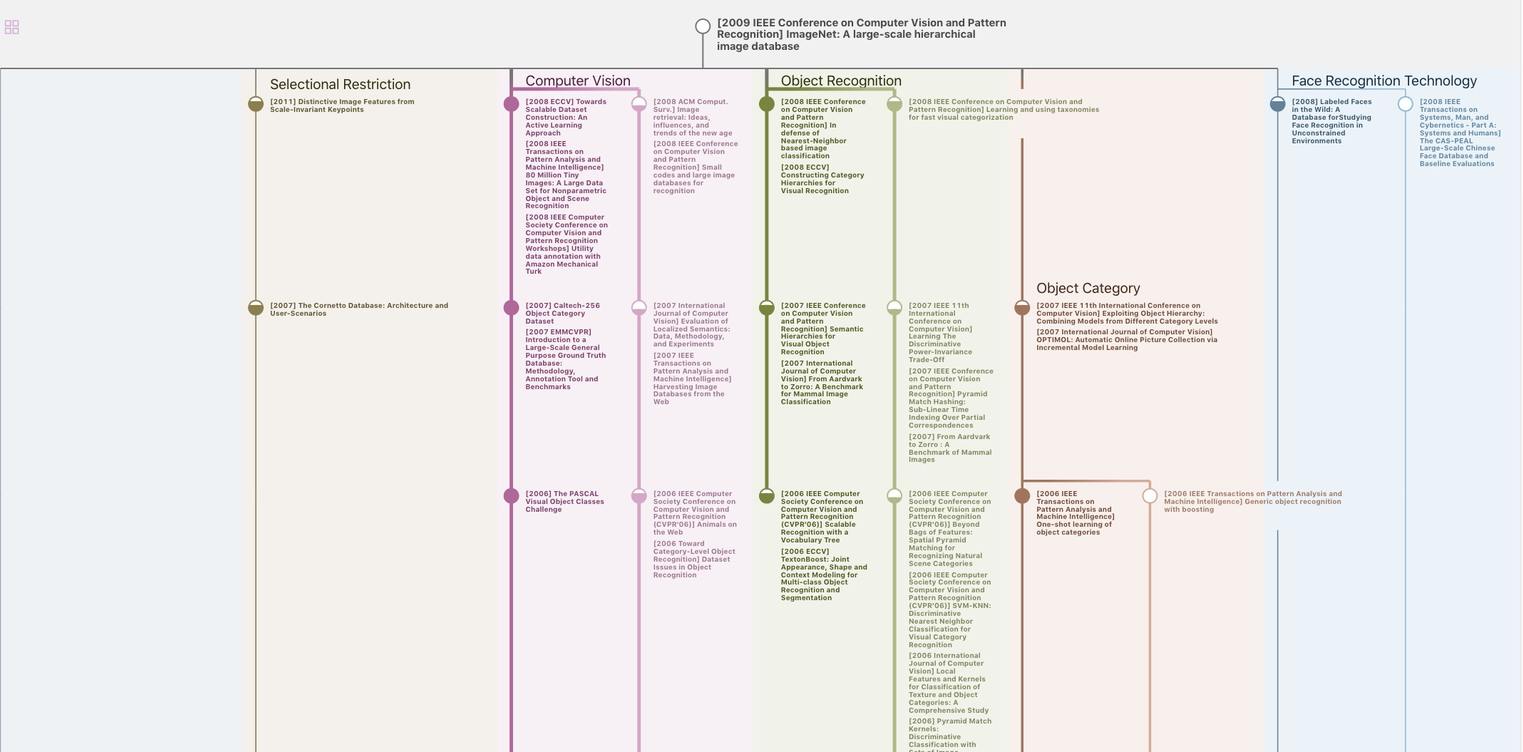
生成溯源树,研究论文发展脉络
Chat Paper
正在生成论文摘要