Privacy Preserving PCA for Multiparty Modeling
arXiv (Cornell University)(2020)
摘要
In this paper, we present a general multiparty modeling paradigm with PrivacyPreserving Principal Component Analysis (PPPCA) for horizontally partitioneddata. PPPCA can accomplish multiparty cooperative execution of PCA under thepremise of keeping plaintext data locally. We also propose implementationsusing two techniques, i.e., homomorphic encryption and secret sharing. Theoutput of PPPCA can be sent directly to data consumer to build any machinelearning models. We conduct experiments on three UCI benchmark datasets and areal-world fraud detection dataset. Results show that the accuracy of the modelbuilt upon PPPCA is the same as the model with PCA that is built based oncentralized plaintext data.
更多查看译文
关键词
Privacy-Preserving Computation,Secure Multi-party Computation,Differential Privacy,Privacy Preservation,Homomorphic Encryption
AI 理解论文
溯源树
样例
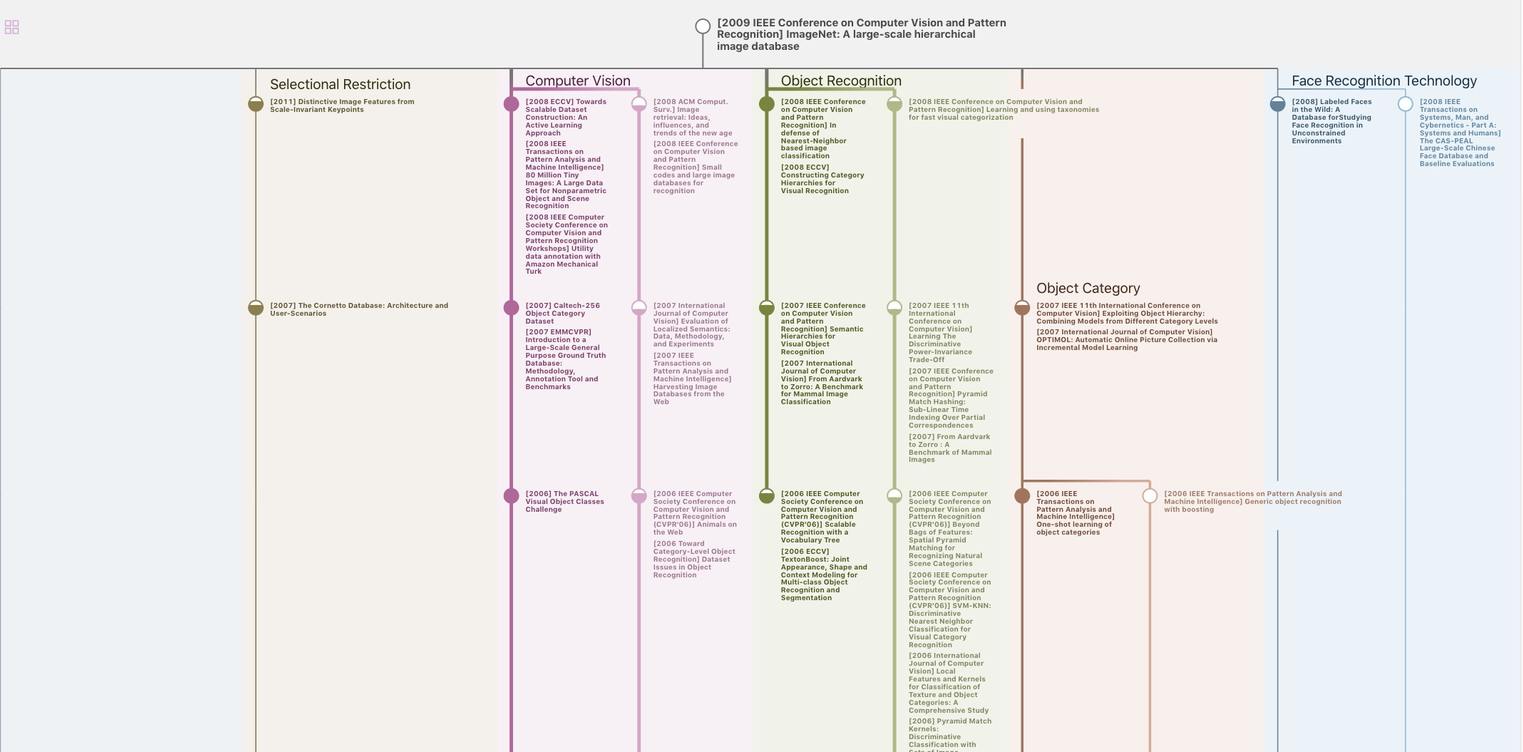
生成溯源树,研究论文发展脉络
Chat Paper
正在生成论文摘要