Transferring Dexterous Surgical Skill Knowledge Between Robots For Semi-Autonomous Teleoperation
2019 28TH IEEE INTERNATIONAL CONFERENCE ON ROBOT AND HUMAN INTERACTIVE COMMUNICATION (RO-MAN)(2019)
摘要
In the future, deployable, teleoperated surgical robots can save the lives of critically injured patients in battlefield environments. These robotic systems will need to have autonomous capabilities to take over during communication delays and unexpected environmental conditions during critical phases of the procedure. Understanding and predicting the next surgical actions (referred as "surgemes") is essential for autonomous surgery. Most approaches for surgeme recognition cannot cope with the high variability associated with austere environments and thereby cannot "transfer" well to field robotics. We propose a methodology that uses compact image representations with kinematic features for surgeme recognition in the DESK dataset. This dataset offers samples for surgical procedures over different robotic platforms with a high variability in the setup. We performed surgeme classification in two setups: 1) No transfer, 2) Transfer from a simulated scenario to two real deployable robots. Then, the results were compared with recognition accuracies using only kinematic data with the same experimental setup. The results show that our approach improves the recognition performance over kinematic data across different domains. The proposed approach produced a transfer accuracy gain up to 20% between the simulated and the real robot, and up to 31% between the simulated robot and a different robot. A transfer accuracy gain was observed for all cases, even those already above 90%.
更多查看译文
关键词
deployable robots,kinematic data,dexterous surgical skill knowledge,semiautonomous teleoperation,teleoperated surgical robots,critically injured patients,robotic systems,surgical actions,autonomous surgery,surgeme recognition,austere environments,compact image representations,kinematic features,DESK dataset,surgical procedures,surgeme classification
AI 理解论文
溯源树
样例
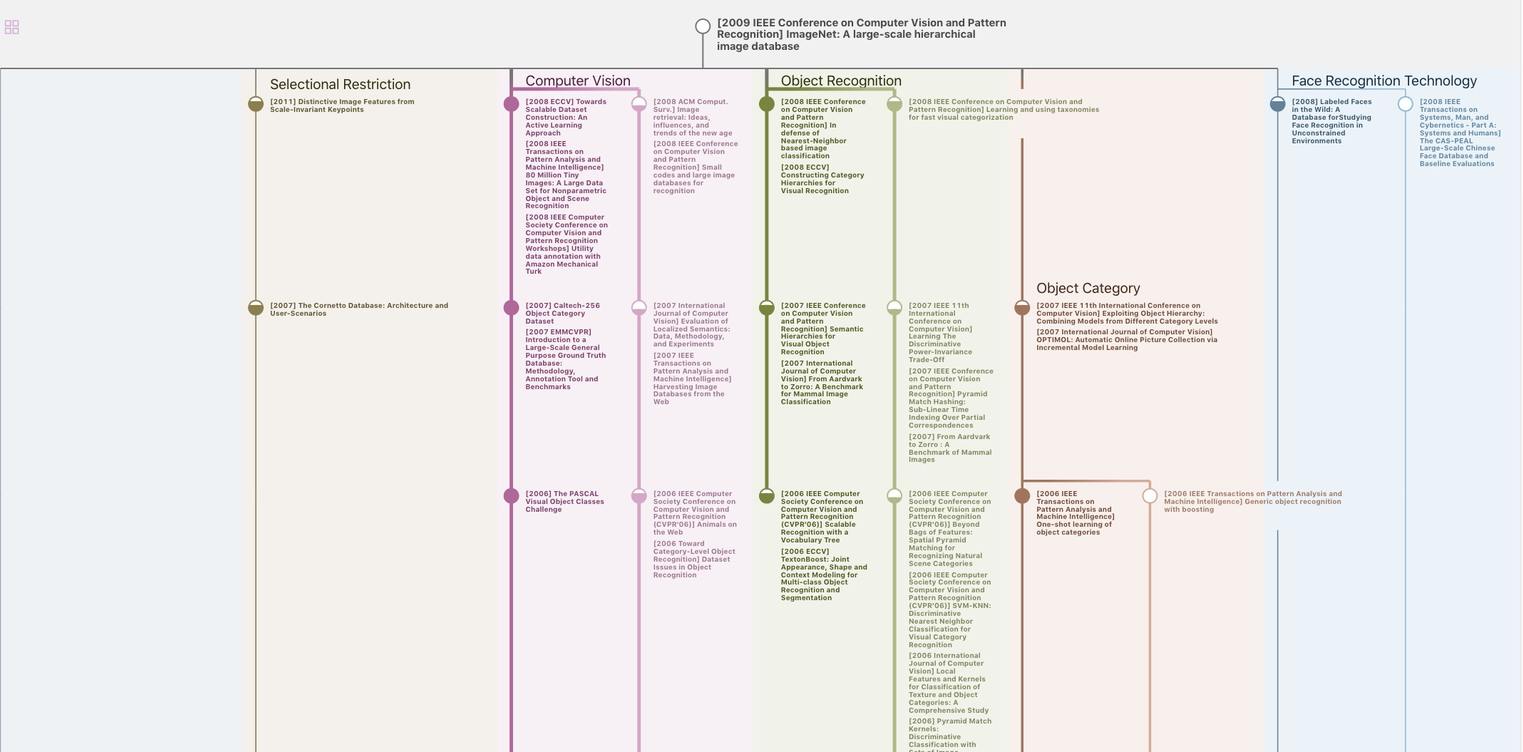
生成溯源树,研究论文发展脉络
Chat Paper
正在生成论文摘要