Smak-Net: Self-Supervised Multi-Level Spatial Attention Network For Knowledge Representation Towards Imitation Learning
2019 28TH IEEE INTERNATIONAL CONFERENCE ON ROBOT AND HUMAN INTERACTIVE COMMUNICATION (RO-MAN)(2019)
摘要
In this paper, we propose an end-to-end self-supervised feature representation network for imitation learning. The proposed network incorporates a novel multi-level spatial attention module to amplify the relevant and suppress the irrelevant information while learning task-specific feature embeddings. The multi-level attention module takes multiple intermediate feature maps of the input image at different stages of the CNN pipeline and results a 2D matrix of compatibility scores for each feature map with respect to the given task. The weighted combination of the feature vectors with the scores estimated from attention modules leads to a more task specific feature representation of the input images. We thus name the proposed network as SMAK-Net, abbreviated from Self-supervised Multi-level spatial Attention Knowledge representation Network. We have trained this network using a metric learning loss which aims to decrease the distance between the feature representations of simultaneous frames from multiple view points and increases the distance between the neighboring frames of the same view point. The experiments are performed on the publicly available Multi-View pouring dataset [1]. The outputs of the attention module are demonstrated to highlight the task specific objects while suppressing the rest of the background in the input image. The proposed method is validated by qualitative and quantitative comparisons with the state-of-the art technique TCN [1] along with intensive ablation studies. This method is shown to significantly outperform TCN by 6 :5% in the temporal alignment error metric while reducing the total number of training steps by 155K.
更多查看译文
关键词
SMAK-Net,imitation learning,end-to-end self-supervised feature representation network,task-specific feature embeddings,multiple intermediate feature maps,feature vectors,metric learning loss,multiple view points,self-supervised multilevel spatial attention network,multilevel spatial attention module,CNN pipeline,2D matrix,self-supervised multilevel spatial attention knowledge representation network
AI 理解论文
溯源树
样例
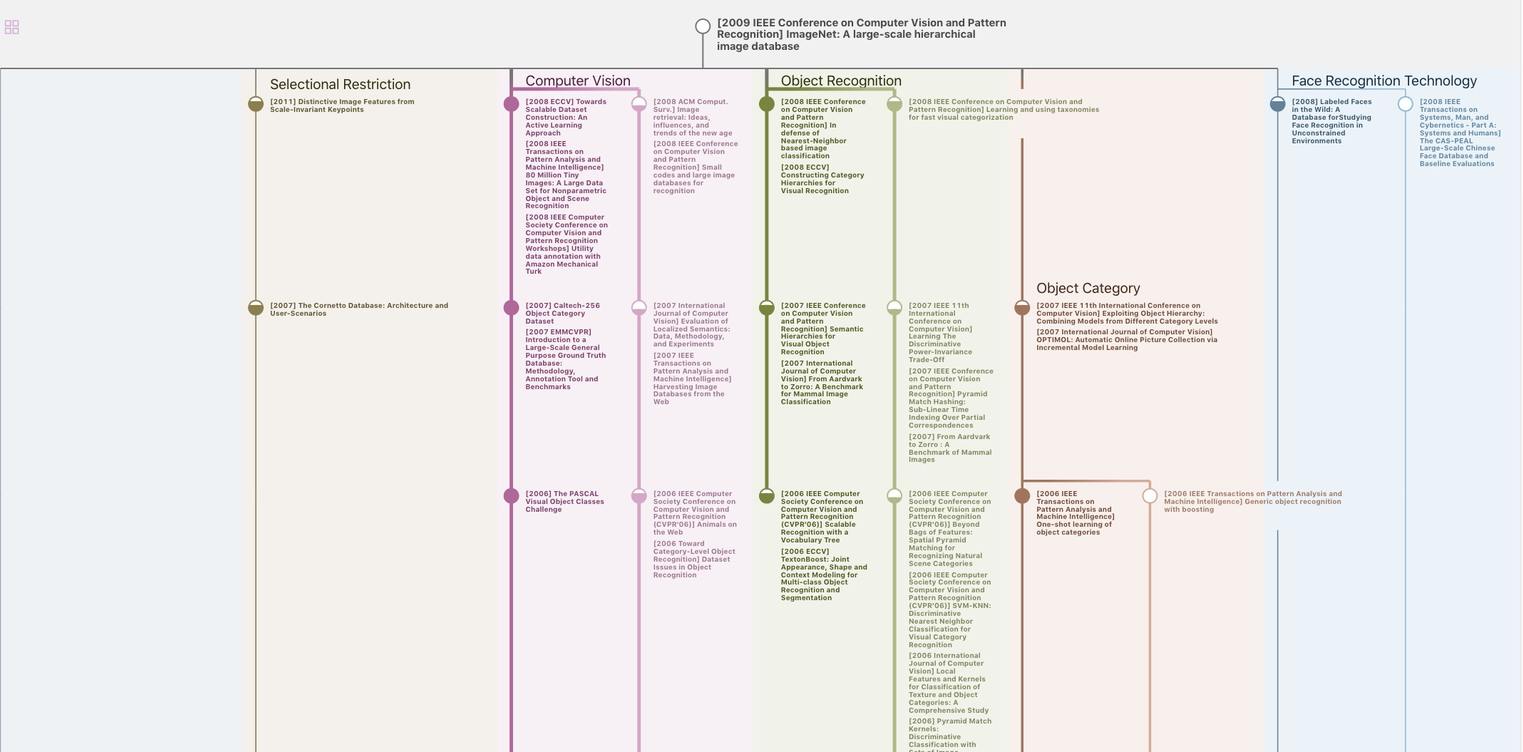
生成溯源树,研究论文发展脉络
Chat Paper
正在生成论文摘要