Mind the gap: an experimental evaluation of imputation of missing values techniques in time series
Hosted Content(2020)
摘要
AbstractRecording sensor data is seldom a perfect process. Failures in power, communication or storage can leave occasional blocks of data missing, affecting not only real-time monitoring but also compromising the quality of near- and off-line data analysis. Several recovery (imputation) algorithms have been proposed to replace missing blocks. Unfortunately, little is known about their relative performance, as existing comparisons are limited to either a small subset of relevant algorithms or to very few datasets or often both. Drawing general conclusions in this case remains a challenge.In this paper, we empirically compare twelve recovery algorithms using a novel benchmark. All but two of the algorithms were re-implemented in a uniform test environment. The benchmark gathers ten different datasets, which collectively represent a broad range of applications. Our benchmark allows us to fairly evaluate the strengths and weaknesses of each approach, and to recommend the best technique on a use-case basis. It also allows us to identify the limitations of the current body of algorithms and suggest future research directions.
更多查看译文
AI 理解论文
溯源树
样例
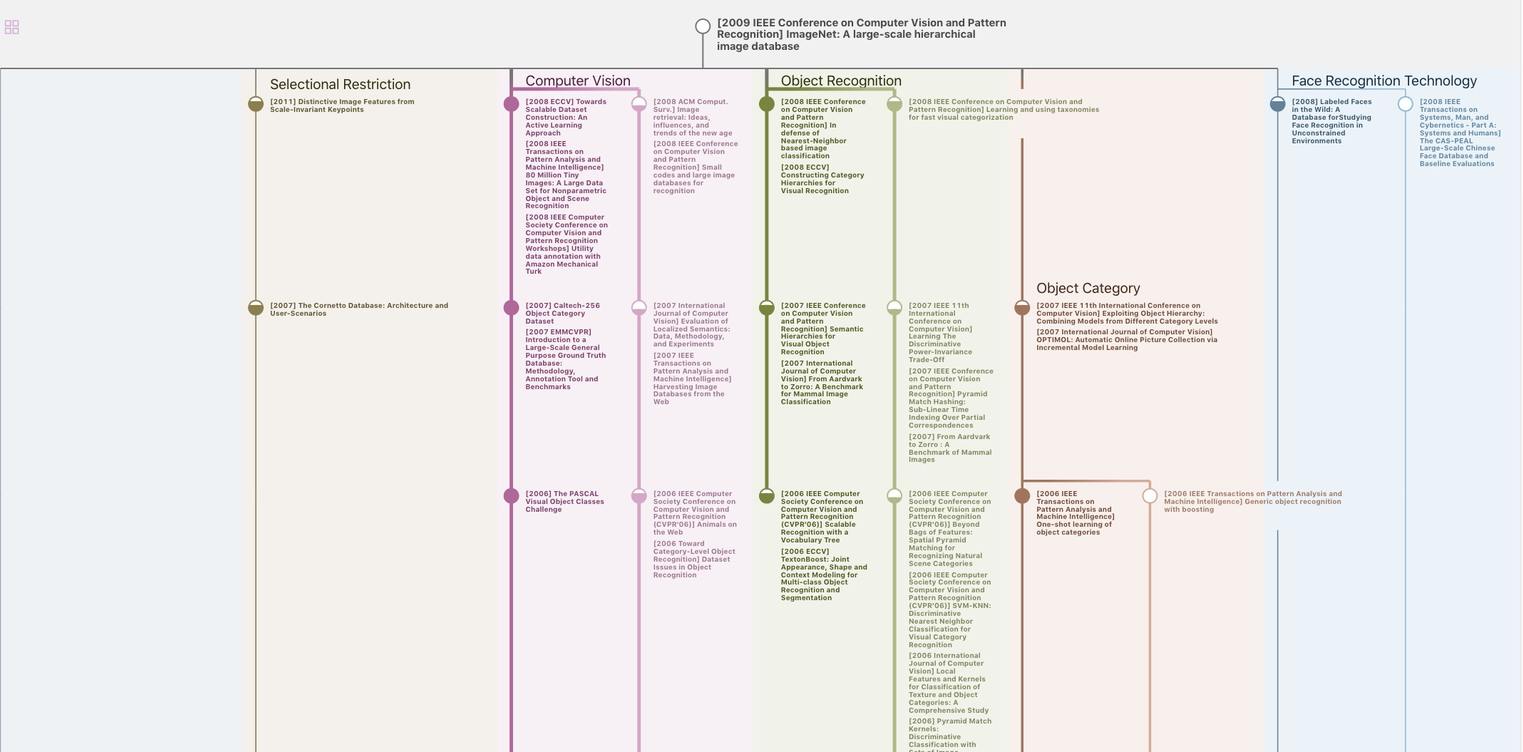
生成溯源树,研究论文发展脉络
Chat Paper
正在生成论文摘要