Evaluating Variational Autoencoder as a Private Data Release Mechanism for Tabular Data
2019 IEEE 24th Pacific Rim International Symposium on Dependable Computing (PRDC)(2019)
摘要
Multi-market businesses can collect data from different business entities and aggregate data from various sources to create value. However, due to the restriction of privacy regulation, it could be illegal to exchange data between business entities of the same parent company, unless the users have opted-in to allow it. Regulations such as the EU's GDPR allows data exchange if data is anonymized appropriately. In this study, we use variational autoencoder as a mechanism to generate synthetic data. The privacy and utility of the generated data sets are measured. And its performance is compared with the performance of the plain autoencoder. The primary findings of this study are 1) variational autoencoder can be an option for data exchange with good accuracy even when the number of latent dimensions is low 2) plain autoencoder still provides better accuracy when the number of hidden nodes is high 3) variational autoencoder, as a generative model, can be given to a data user to generate his version of data that closely mimic the original data set.
更多查看译文
关键词
variational autoencoder, private data release, k anonymity, k Level
AI 理解论文
溯源树
样例
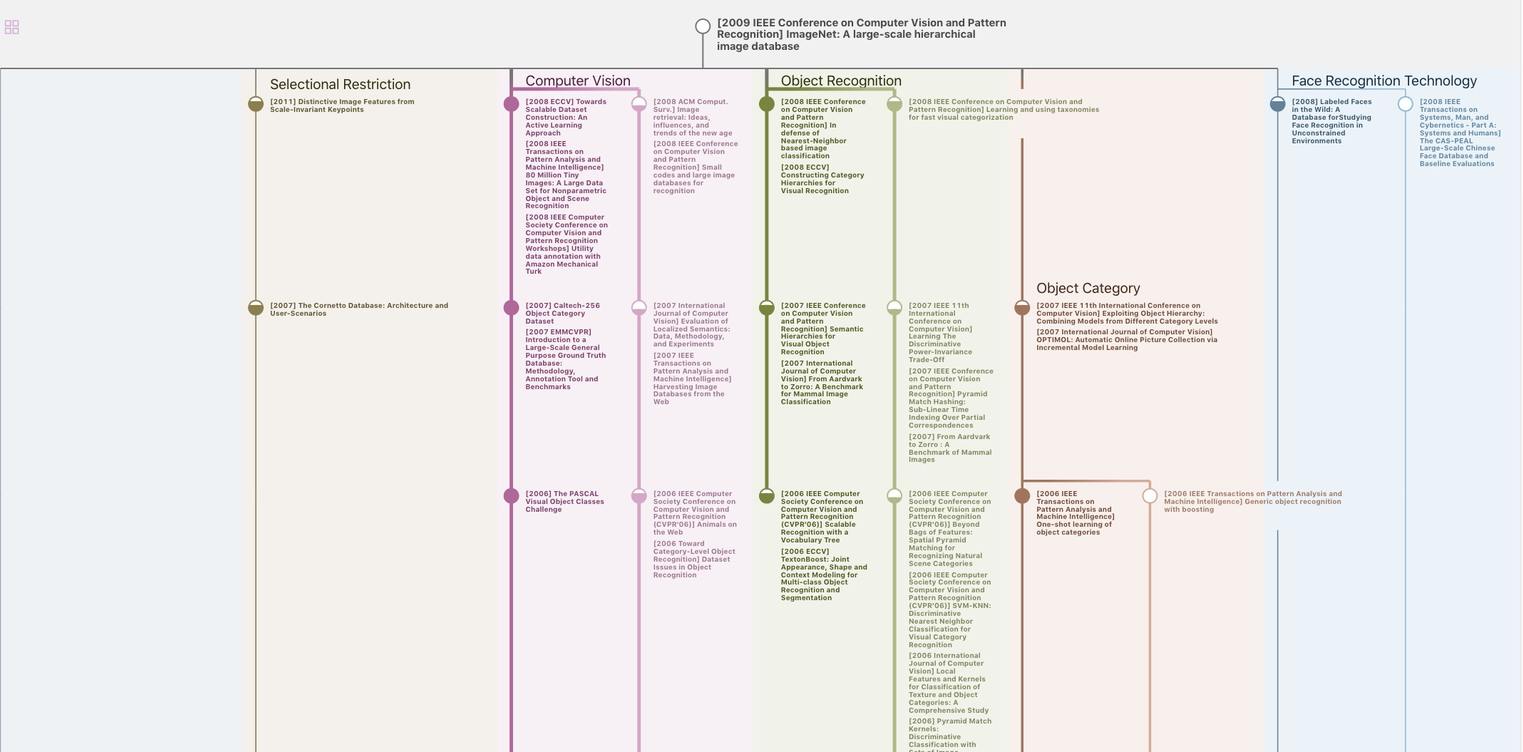
生成溯源树,研究论文发展脉络
Chat Paper
正在生成论文摘要