Importance-Aware Filter Selection for Convolutional Neural Network Acceleration
2019 IEEE Visual Communications and Image Processing (VCIP)(2019)
摘要
Convolutional Neural Networks(CNNs) are widely used in many fields, including artificial intelligence, computer vision and video coding. However, CNNs are typically over-parameterized and contain significant redundancy. Traditional model acceleration methods mainly rely on specific manual rules. This usually leads to sub-optimal results with relatively limited compression ratio. Recent works have deployed the self-learning agent on the layer-level acceleration but still combined with human-designed criterias. In this paper, we proposed a filter-based model acceleration method to directly and automatically decide which filters should be pruned with the reinforcement learning method DDPG. We designed a novel reward function with the reward shaping technique for the training process. Our method is utilized on the models trained on MNIST and CIFAR-10 datasets and achieves both higher acceleration ratio and less accuracy loss than the conventional methods simultaneously.
更多查看译文
关键词
Deep learning,Model acceleration,CNNs acceleration,Reinforcement learning
AI 理解论文
溯源树
样例
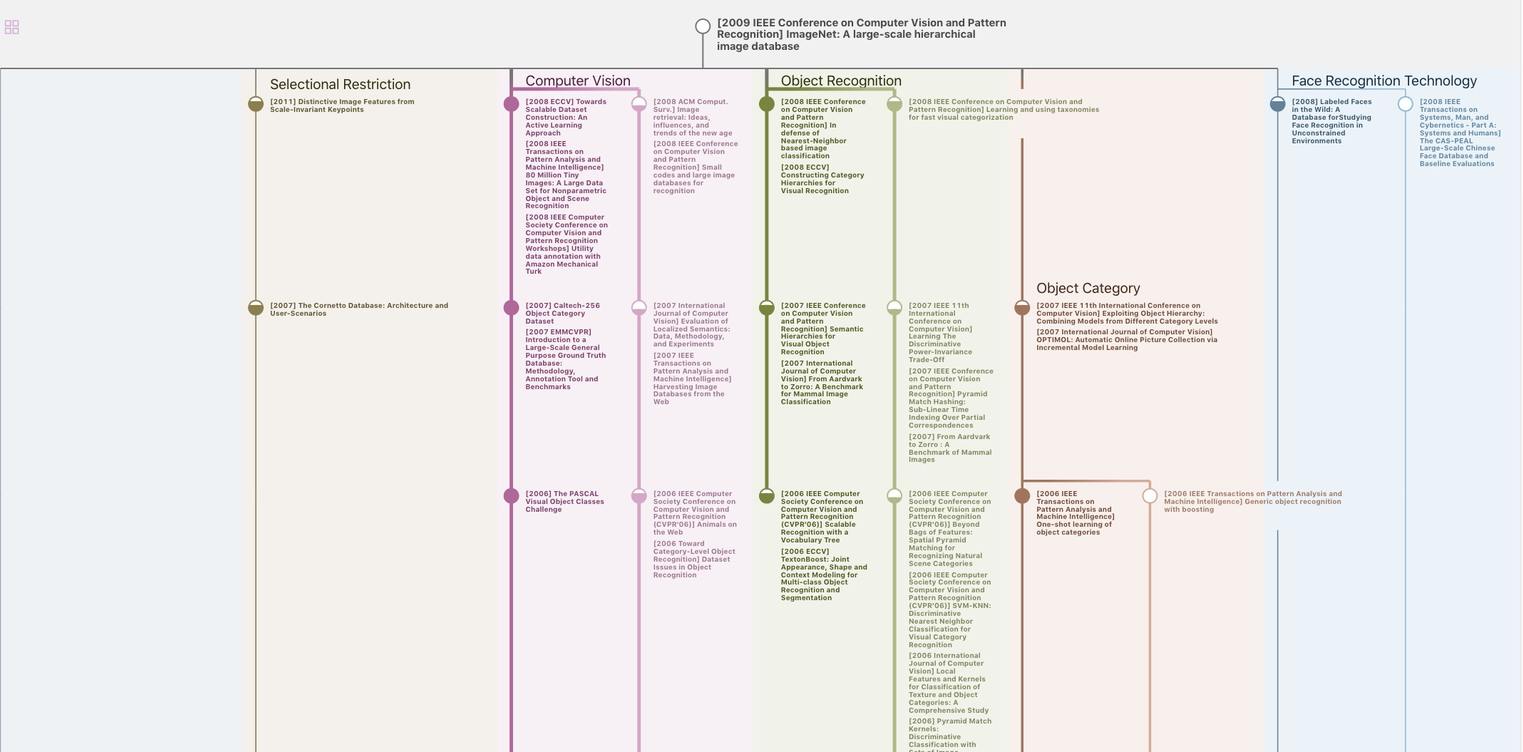
生成溯源树,研究论文发展脉络
Chat Paper
正在生成论文摘要