Design and Comprehensive Analysis of a Noise-Tolerant ZNN Model With Limited-Time Convergence for Time-Dependent Nonlinear Minimization
IEEE Transactions on Neural Networks and Learning Systems(2020)
摘要
Zeroing neural network (ZNN) is a powerful tool to address the mathematical and optimization problems broadly arisen in the science and engineering areas. The convergence and robustness are always co-pursued in ZNN. However, there exists no related work on the ZNN for time-dependent nonlinear minimization that achieves simultaneously limited-time convergence and inherently noise suppression. In this article, for the purpose of satisfying such two requirements, a limited-time robust neural network (LTRNN) is devised and presented to solve time-dependent nonlinear minimization under various external disturbances. Different from the previous ZNN model for this problem either with limited-time convergence or with noise suppression, the proposed LTRNN model simultaneously possesses such two characteristics. Besides, rigorous theoretical analyses are given to prove the superior performance of the LTRNN model when adopted to solve time-dependent nonlinear minimization under external disturbances. Comparative results also substantiate the effectiveness and advantages of LTRNN via solving a time-dependent nonlinear minimization problem.
更多查看译文
关键词
Limited-time convergence,nonlinear minimization,robustness,time varying,zeroing neural networks (ZNNs),Zhang neural networks
AI 理解论文
溯源树
样例
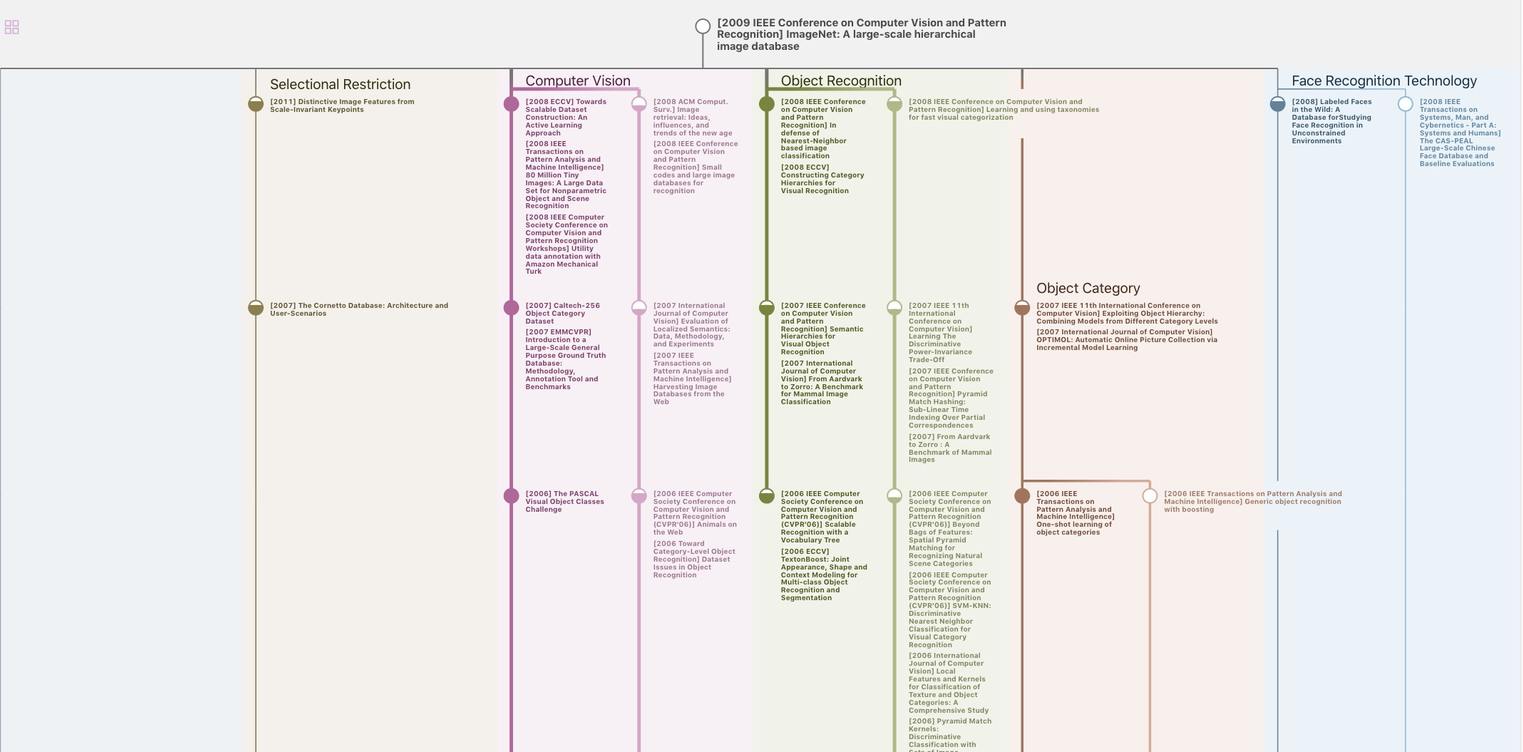
生成溯源树,研究论文发展脉络
Chat Paper
正在生成论文摘要