MAPPING BRAIN STRUCTURAL CONNECTIVITIES TO FUNCTIONAL NETWORKS VIA GRAPH ENCODER-DECODER WITH INTERPRETABLE LATENT EMBEDDINGS
IEEE Global Conference on Signal and Information Processing(2019)
摘要
In this paper, the relationship between functional and structural brain networks is investigated by training a graph encoder-decoder system to learn the mapping from brain structural connectivity (SC) to functional connectivity (FC). Our work leverages a graph convolutional network (GCN) model in the encoder which integrates both nodal attributes and the network topology information to generate new graph representations in lower dimensions. Using brain SC graphs as inputs, the novel GCN-based encoder-decoder system manages to account for both direct and indirect interactions between brain regions to reconstruct the empirical FC networks. In doing so, the latent variables within the system (i.e., the learnt low dimensional embeddings) capture important information regarding the relation between functional and structural networks. By decomposing the reconstructed functional networks in terms of the output of each graph convolution filter, we can extract those brain regions which contribute most to the generation of FC networks from their SC counterparts. Experiments on a large population of healthy subjects from the Human Connectome Project show our model can learn a generalizable and interpretable SC-FC relationship. Overall, results here support the promising prospect of using GCNs to discover more about the complex nature of human brain activity and function.
更多查看译文
关键词
Brain networks,graph convolutional network,encoder-decoder system,graph embedding,structural connectivity-functional connectivity relationship
AI 理解论文
溯源树
样例
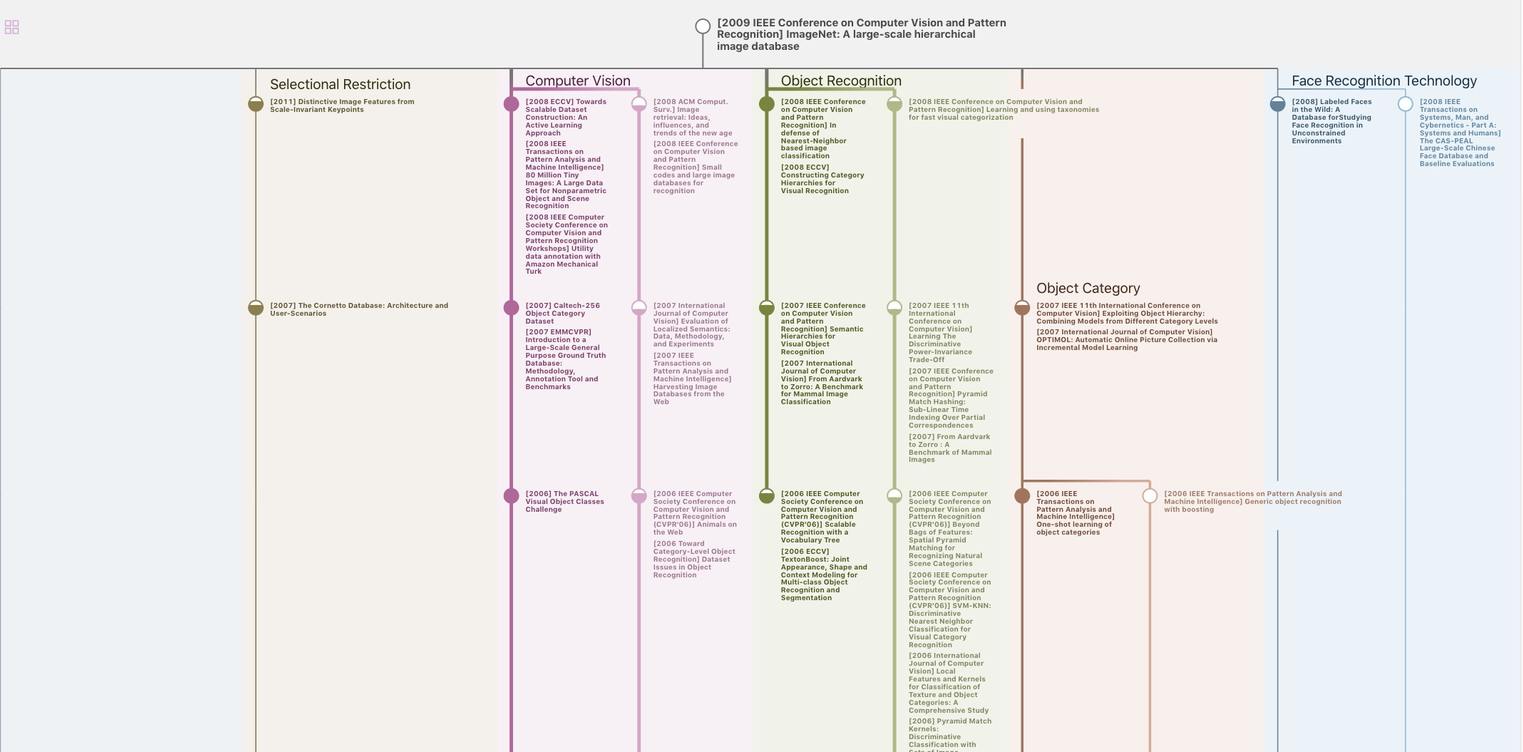
生成溯源树,研究论文发展脉络
Chat Paper
正在生成论文摘要