Feature Learning for Enhanced Security in the Internet of Things
2019 7TH IEEE GLOBAL CONFERENCE ON SIGNAL AND INFORMATION PROCESSING (IEEE GLOBALSIP)(2019)
关键词
Internet of Things,RF fingerprinting,Variational Inference,Deep Learning
AI 理解论文
溯源树
样例
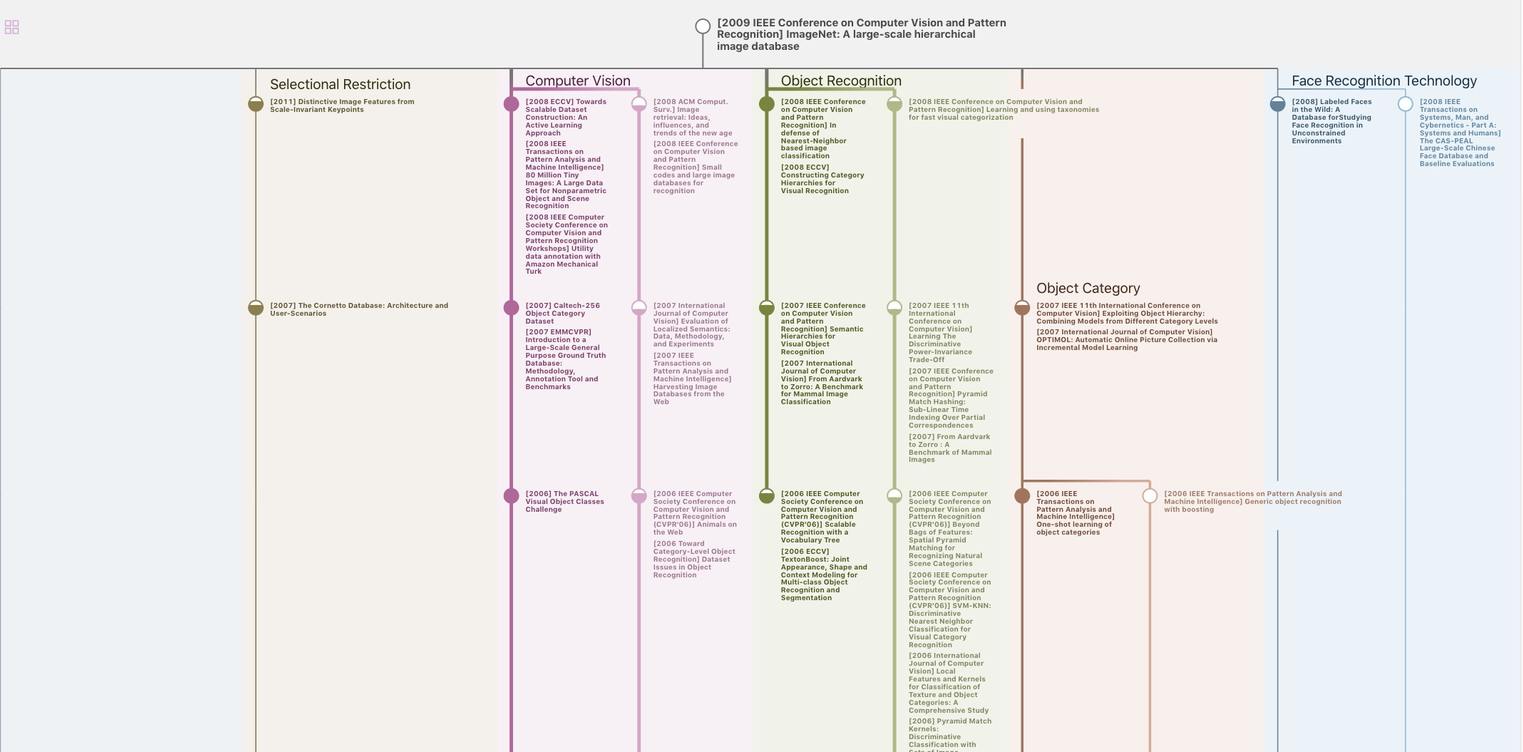
生成溯源树,研究论文发展脉络
Chat Paper
正在生成论文摘要