Truncated Hawkes point process modeling: System theory and system identification.
Automatica(2020)
摘要
Point processes have a growing list of applications, including, neuroscience, high frequency finance, genomics and more recently, streaming data. Perhaps the most used parameterized dynamic (aka self-exciting i.e. history dependent) model is the (linear) Hawkes model. But the Hawkes model assumes the infinite past is available and has to be truncated for empirical use. Here we develop, for the first time, the system theory for the truncated Hawkes process. This includes: necessary and sufficient conditions for stability; a Wold decomposition; and an asymptotic spectrum. We then discuss various parameterizations (Exponential, Laguerre, Binomial-autoregression) and their properties. Using the Laguerre parameterization, we next develop a new continuous time multiplicative EM algorithm for maximum likelihood estimation and develop some of its properties. It turns out that the multiplicative EM algorithm does not guarantee stability and so we develop a modified multiplicative EM that does. We also derive, for the first time for self-exciting processes, the Cramer–Rao bound as well as a BIC model selection criterion. We illustrate with simulations, comparing the Laguerre approach to the popular binomial-autoregression and then with an application to neural data recorded from a cat’s brain under visual stimuli.
更多查看译文
关键词
Point processes,System identification,Maximum likelihood,Hawkes processes
AI 理解论文
溯源树
样例
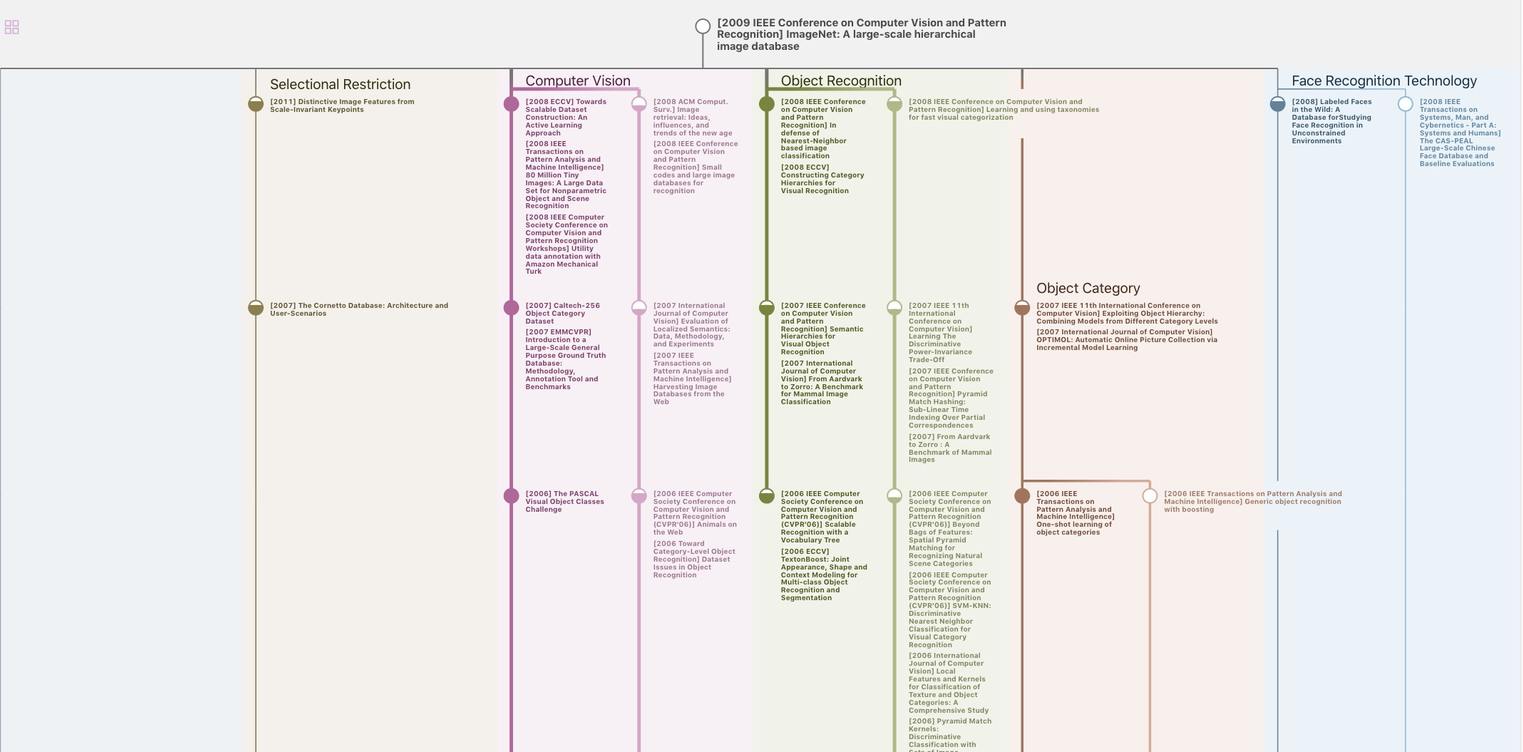
生成溯源树,研究论文发展脉络
Chat Paper
正在生成论文摘要