A Circularly Polarized Horn Antenna Based on an FSS Polarization Converter
IEEE Antennas and Wireless Propagation Letters(2020)SCI 2区
Southeast Univ | SARFT
Abstract
This letter designed a circularly polarized horn antenna by loading a frequency selective surface (FSS) polarization converter at the aperture of a standard horn. The proposed polarization converter is based on multilayer nonresonant FSS. It is composed of a two-layer dielectric slab and three printed metal layers, which are etched on the top, middle, and bottom layers of the slab. The three metal layers consist of a two-layer patch with a square truncated corner and a one-layer grid line with a slot. By introducing perturbation to both the patch and the grid line, the polarization converter shows a low profile and subwavelength unit size, whose dimension is 0.18λ0 × 0.18λ0 while the thickness is only 0.1λ0. The simulated results show that the axial ratio (AR) is less than 3 dB in the frequency range of 9.6–11.7 GHz, i.e., a fractional bandwidth of 19.7% within the targeted frequency band. The measurement results are in a good agreement with the simulation results, which proves the feasibility of the design.
MoreTranslated text
Key words
Axial ratio (AR),frequency selective surface (FSS),horn,polarization converter
PDF
View via Publisher
AI Read Science
AI Summary
AI Summary is the key point extracted automatically understanding the full text of the paper, including the background, methods, results, conclusions, icons and other key content, so that you can get the outline of the paper at a glance.
Example
Background
Key content
Introduction
Methods
Results
Related work
Fund
Key content
- Pretraining has recently greatly promoted the development of natural language processing (NLP)
- We show that M6 outperforms the baselines in multimodal downstream tasks, and the large M6 with 10 parameters can reach a better performance
- We propose a method called M6 that is able to process information of multiple modalities and perform both single-modal and cross-modal understanding and generation
- The model is scaled to large model with 10 billion parameters with sophisticated deployment, and the 10 -parameter M6-large is the largest pretrained model in Chinese
- Experimental results show that our proposed M6 outperforms the baseline in a number of downstream tasks concerning both single modality and multiple modalities We will continue the pretraining of extremely large models by increasing data to explore the limit of its performance
Try using models to generate summary,it takes about 60s
Must-Reading Tree
Example
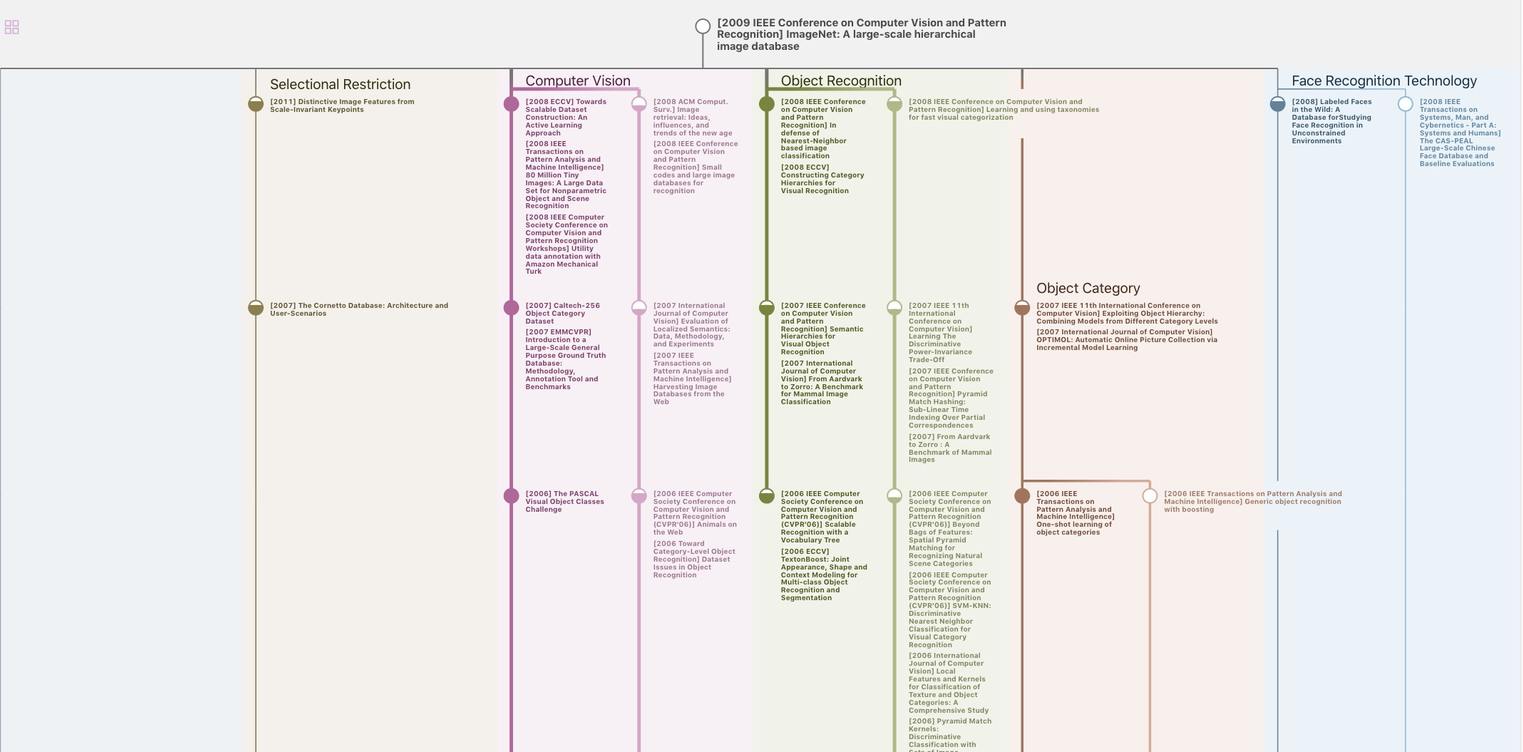
Generate MRT to find the research sequence of this paper
Related Papers
Metasurface Concept for Mm-Wave Wideband Circularly Polarized Horns Design
IEEE Transactions on Antennas and Propagation 2020
被引用9
Optics Communications 2021
被引用45
IEEE Sensors Journal 2021
被引用7
A Triple‐band Composite Base Station and Satellite Antenna Array for Maritime Application
International Journal of RF and Microwave Computer-Aided Engineering 2022
被引用4
Improved Co-substituted Zinc Vanadate Ceramics Based on LTCC for Enhanced Polarization Converters
JOURNAL OF ALLOYS AND COMPOUNDS 2022
被引用15
Sensors 2022
被引用2
Design of Polarization-Insensitive Polarization Converter Based on Frequency Selective Surface
Optics Communications 2022
被引用2
Design of Miniaturized Frequency-Selective Rasorber with Embedded Dual-Bow Resonators
IEEE ANTENNAS AND WIRELESS PROPAGATION LETTERS 2023
被引用2
Broadband Linear to Circular Polarizer Based on Multilayer Frequency-Selective Surface
INTERNATIONAL JOURNAL OF ANTENNAS AND PROPAGATION 2023
被引用1
A Flexible and Transparent Broadband Metasurface Polarization Converter
IEEE Antennas and Wireless Propagation Letters 2024
被引用1
Data Disclaimer
The page data are from open Internet sources, cooperative publishers and automatic analysis results through AI technology. We do not make any commitments and guarantees for the validity, accuracy, correctness, reliability, completeness and timeliness of the page data. If you have any questions, please contact us by email: report@aminer.cn
Chat Paper
去 AI 文献库 对话