Multi-Task Learning By A Top-Down Control Network.
ICIP(2021)
摘要
A general problem that received considerable recent attention is how to perform multiple tasks in the same network, maximizing both prediction accuracy and efficiency of training. Recent approaches address this problem by branching networks, or by a channel-wise modulation of the feature-maps with task specific vectors. We propose a novel architecture that uses a top-down network to modify the main network according to the task in a channel-wise, as well as spatial-wise, image-dependent computation scheme. We show the effectiveness of our scheme by achieving better results than alternative state-of-the-art approaches to multi-task learning. We also demonstrate our advantages in terms of task selectivity, scaling the number of tasks, learning from fewer examples and interpretability.
更多查看译文
关键词
Multi-task learning,Deep learning
AI 理解论文
溯源树
样例
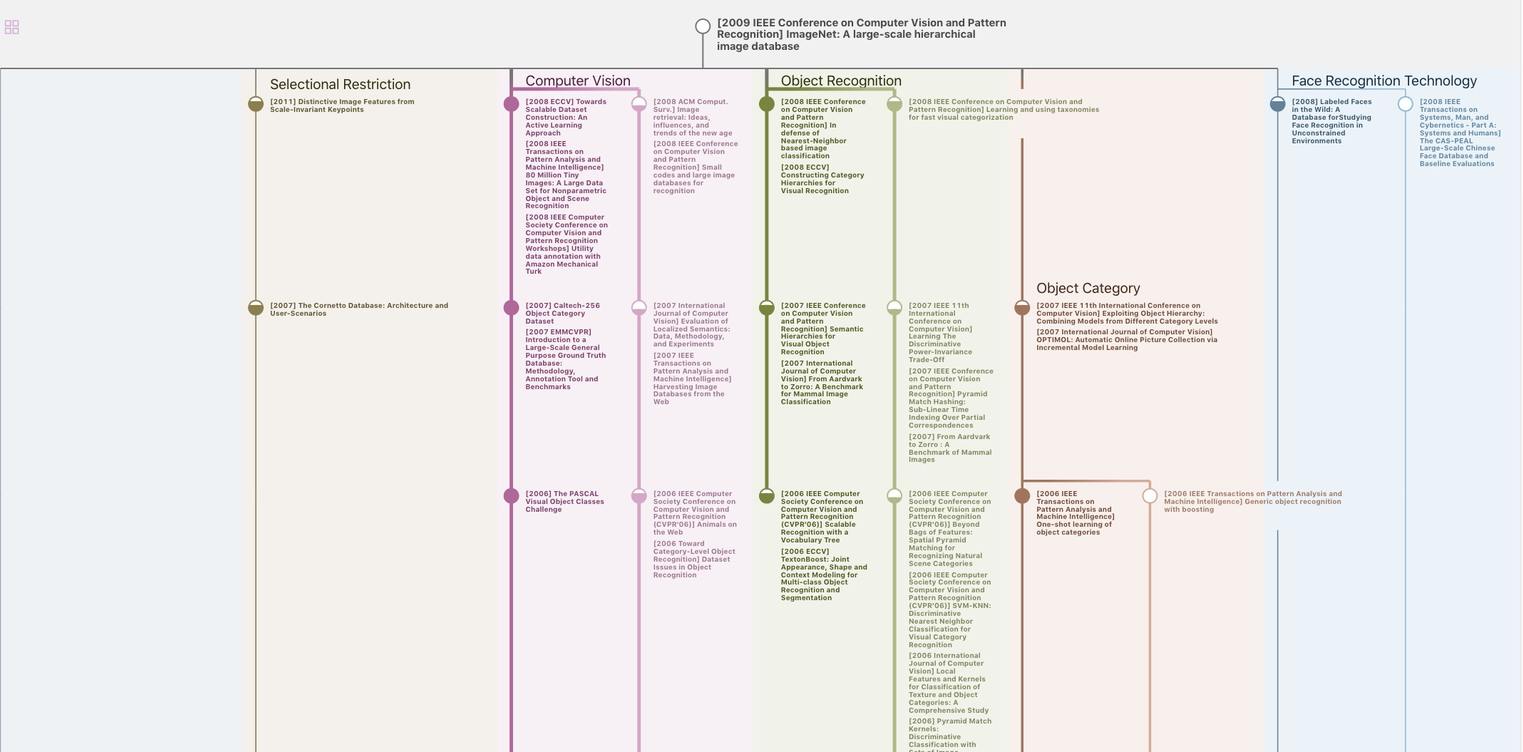
生成溯源树,研究论文发展脉络
Chat Paper
正在生成论文摘要