Graph Neural Distance Metric Learning with Graph-Bert
arxiv(2020)
摘要
Graph distance metric learning serves as the foundation for many graph learning problems, e.g., graph clustering, graph classification and graph matching. Existing research works on graph distance metric (or graph kernels) learning fail to maintain the basic properties of such metrics, e.g., non-negative, identity of indiscernibles, symmetry and triangle inequality, respectively. In this paper, we will introduce a new graph neural network based distance metric learning approaches, namely GB-DISTANCE (GRAPH-BERT based Neural Distance). Solely based on the attention mechanism, GB-DISTANCE can learn graph instance representations effectively based on a pre-trained GRAPH-BERT model. Different from the existing supervised/unsupervised metrics, GB-DISTANCE can be learned effectively in a semi-supervised manner. In addition, GB-DISTANCE can also maintain the distance metric basic properties mentioned above. Extensive experiments have been done on several benchmark graph datasets, and the results demonstrate that GB-DISTANCE can out-perform the existing baseline methods, especially the recent graph neural network model based graph metrics, with a significant gap in computing the graph distance.
更多查看译文
关键词
distance,learning,graph-bert
AI 理解论文
溯源树
样例
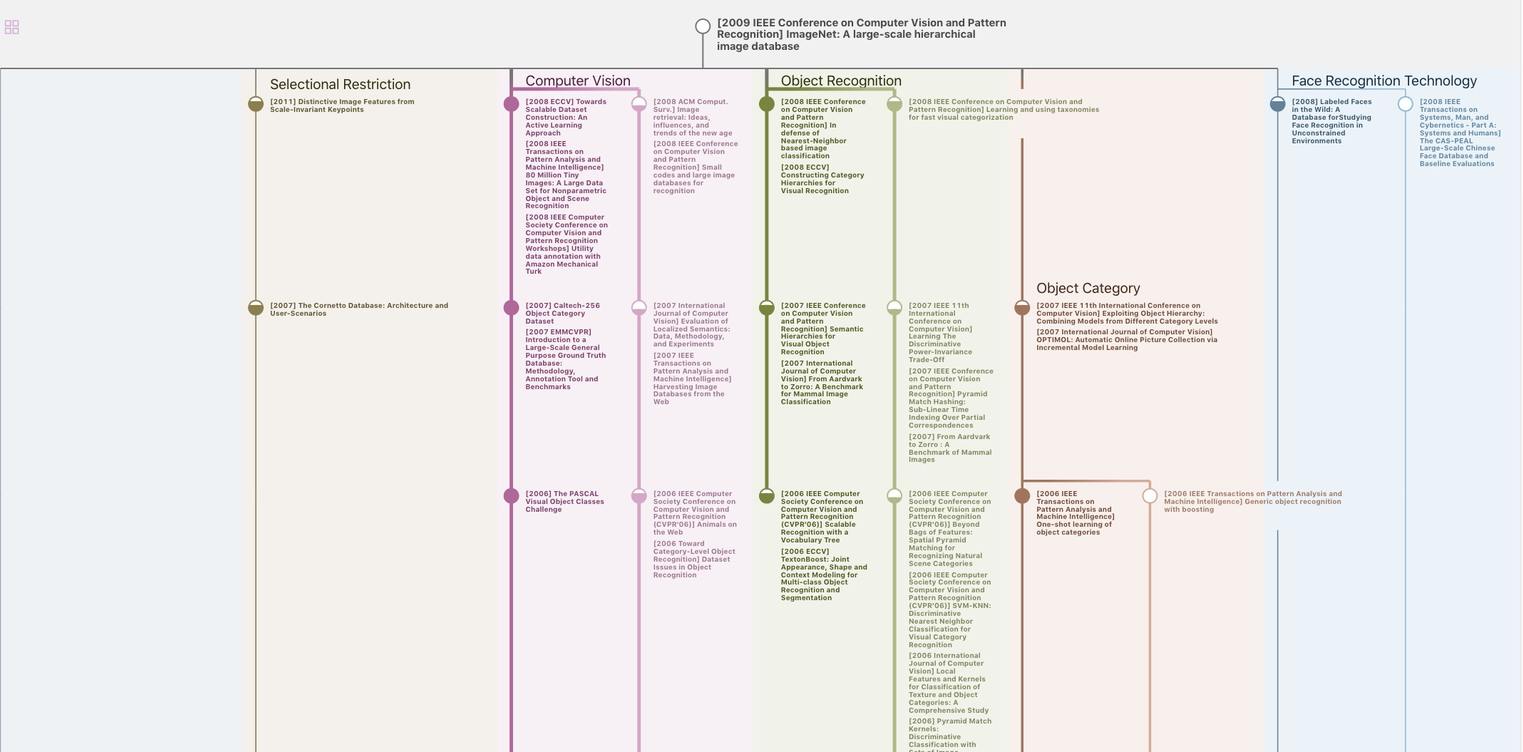
生成溯源树,研究论文发展脉络
Chat Paper
正在生成论文摘要