An enhanced multi-objective grey wolf optimizer for service composition in cloud manufacturing.
Applied Soft Computing(2020)
摘要
This paper presents an enhanced multi-objective grey wolf optimizer (EMOGWO) for multi-objective service composition and optimal selection (MO-SCOS) problem in cloud manufacturing, wherein both the quality of service and the energy consumption are considered from the perspectives of sustainable manufacturing. Given that there are still deficiencies in local optimum and diversity for the original multi-objective grey wolf optimizer (MOGWO). in response, the backward learning strategy is employed to heighten the exploration of initial population, so as to increase the search efficiency. Then, in order to improve the diversity, a nonlinear adjustment strategy for control parameter is proposed to enhance the global exploration of the algorithm. Besides, an enhanced search strategy, which strengthens the exploration of leaders, is designed to avoid the local optimum. Finally, the comparative study with typical multi-objective algorithms for MO-SCOS problems and benchmark problems are carried out to verify the proposed approach. simulation results suggest that the improvements for MOGWO are effective, and the proposed EMOGWO obtains better performance over other compared algorithms.
更多查看译文
关键词
Cloud manufacturing,Service composition,Multi-objective optimization,Energy consumption,Grey wolf optimizer
AI 理解论文
溯源树
样例
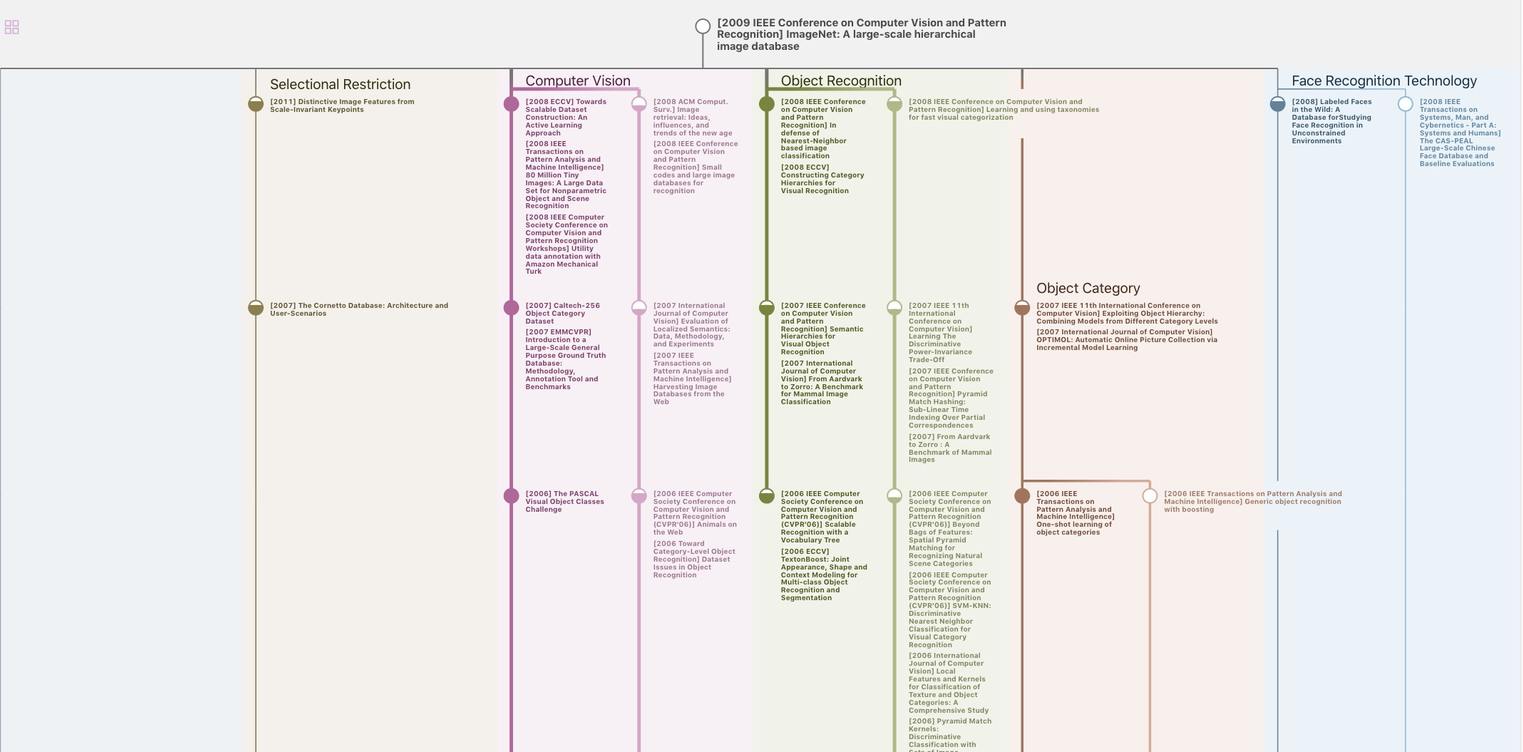
生成溯源树,研究论文发展脉络
Chat Paper
正在生成论文摘要