Conditional Training Based GM and GM-OPELM Data Fusion Schemes in Wireless Sensor Networks
2019 IEEE Pacific Rim Conference on Communications, Computers and Signal Processing (PACRIM)(2019)
摘要
As a key infrastructure of Internet of Things (loT), wireless sensor networks (WSN) can be utilized in a wide range of applications. The prediction based data fusion methods provide effective tools to reduce the amount of data transmissions while maintaining prediction accuracy. Recently a grey prediction model (GM) combining optimally-pruned extreme learning machine (OPELM) data fusion method has been proposed and shown to have good performance. However, the existing GM- OPELM method performs model training and broadcasting before each prediction, resulting in high complexity and energy consumption. In this paper the conditional training based GM (CT-GM) and GM-OPELM (CT-GM-OPELM) are proposed. By introducing an error threshold, the algorithms only perform model training when the prediction error is beyond the threshold. Compared with existing GM and GM-OPELM methods, the CT- GM and CT-GM-OPELM methods not only can achieve the higher rate of acceptable prediction and better time efficiency but also has significant reduction in the energy consumption on model training and transmissions.
更多查看译文
关键词
Wireless sensor networks,grey prediction model,extreme learning machine,data fusion
AI 理解论文
溯源树
样例
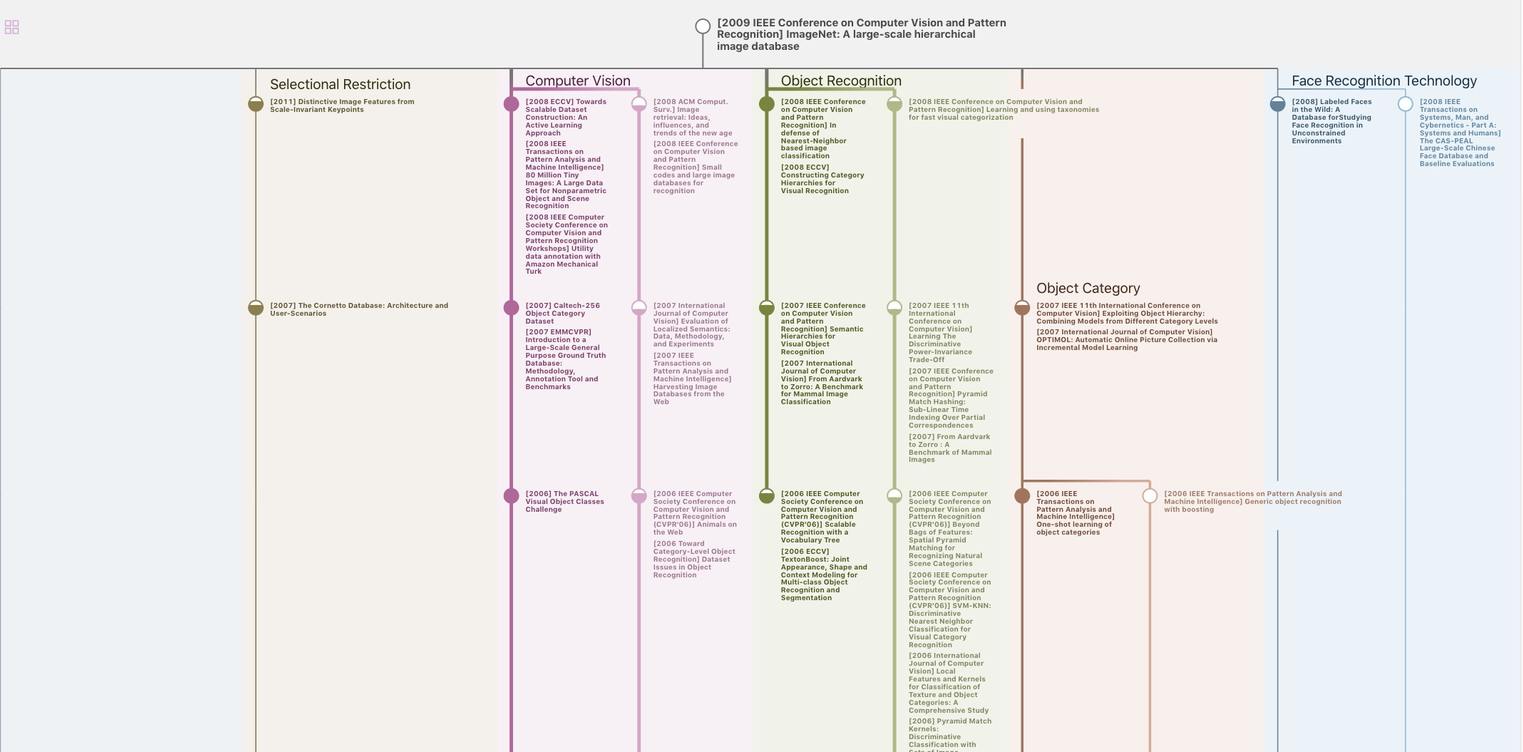
生成溯源树,研究论文发展脉络
Chat Paper
正在生成论文摘要