Adaptive Low-Rank Tensor Approximation for SRAM Yield Analysis using Bootstrap Resampling
2019 IEEE 13th International Conference on ASIC (ASICON)(2019)
摘要
With increasing design complexity and reliability requirement, performance failure has become a main threat for a robust circuit design. The major challenge to develop a high-sigma yield analysis method is “Curse of Dimensionality”. In this paper, we propose a novel Adaptive Low-Rank Tensor Approximation (Adaptive LRTA) method. It starts with a Latin Hypercube Sampling set to build the initial LRTA meta-model. Our adaptive framework has multiple iterations of meta-model refinement. In each iteration, we evaluate the confidence interval by constructing a set of bootstrap resampled LRTA meta-models, and develop a learning function which determines our experimental design enrichment criteria. The experiment results on high-dimensional SRAM column validate that our method has better efficiency and higher accuracy. Our adaptive LRTA uses 2-6X fewer samples than state-of-the-art methods without compromising accuracy.
更多查看译文
关键词
Process Variation,Failure Probability,Low-Rank Tensor Approximation,Bootstrap Resampling
AI 理解论文
溯源树
样例
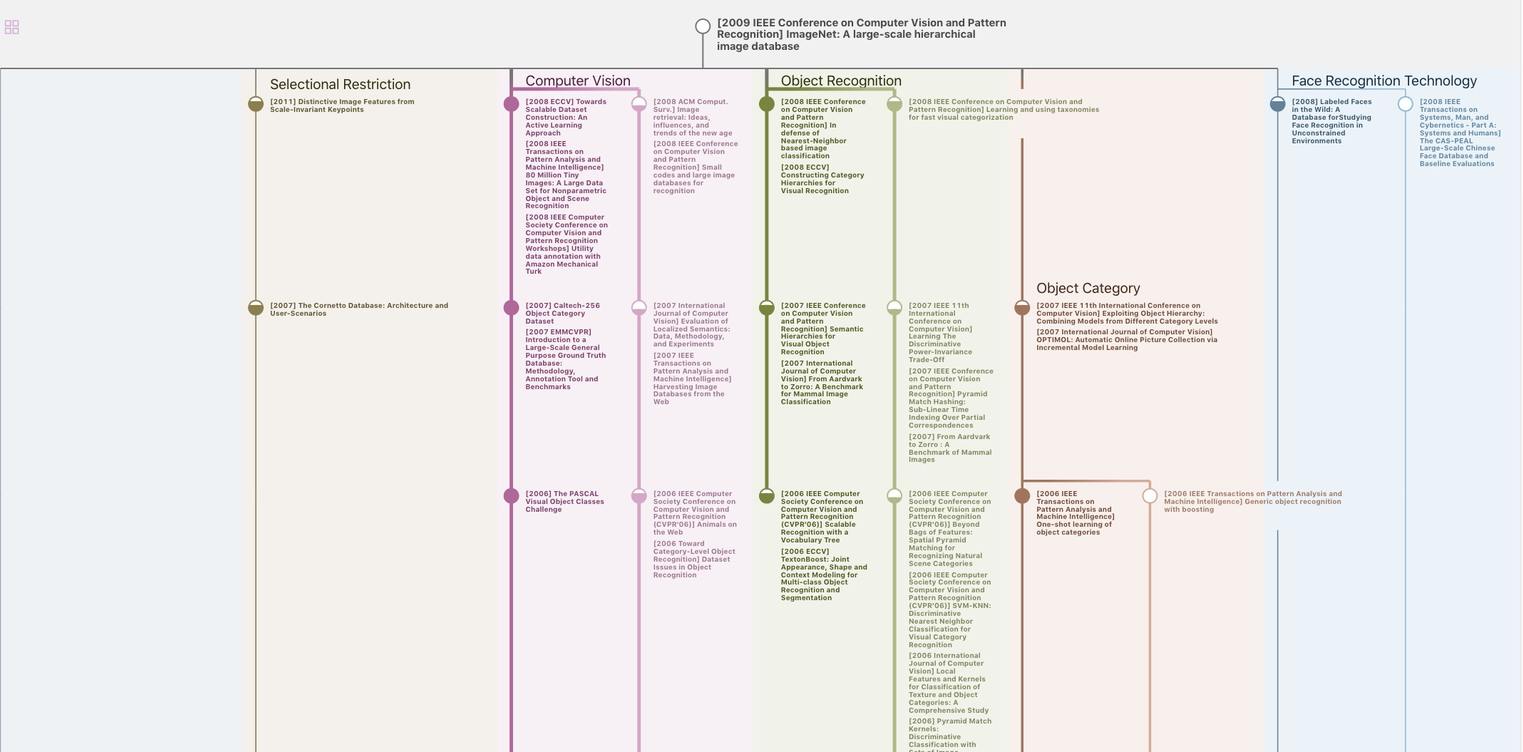
生成溯源树,研究论文发展脉络
Chat Paper
正在生成论文摘要