Pre-Training for Query Rewriting in A Spoken Language Understanding System
ICASSP(2020)
摘要
Query rewriting (QR) is an increasingly important technique to reduce customer friction caused by errors in a spoken language understanding pipeline, where the errors originate from various sources such as speech recognition errors, language understanding errors or entity resolution errors. In this work, we first propose a neural-retrieval based approach for query rewriting. Then, inspired by the wide success of pre-trained contextual language embeddings, and also as a way to compensate for insufficient QR training data, we propose a language-modeling (LM) based approach to pre-train query embeddings on historical user conversation data with a voice assistant. In addition, we propose to use the NLU hypotheses generated by the language understanding system to augment the pre-training. Our experiments show pre-training provides rich prior information and help the QR task achieve strong performance. We also show joint pre-training with NLU hypotheses has further benefit. Finally, after pre-training, we find a small set of rewrite pairs is enough to fine-tune the QR model to outperform a strong baseline by full training on all QR training data.
更多查看译文
关键词
query rewrite, neural retrieval, contextual embeddings, pre-training, user friction
AI 理解论文
溯源树
样例
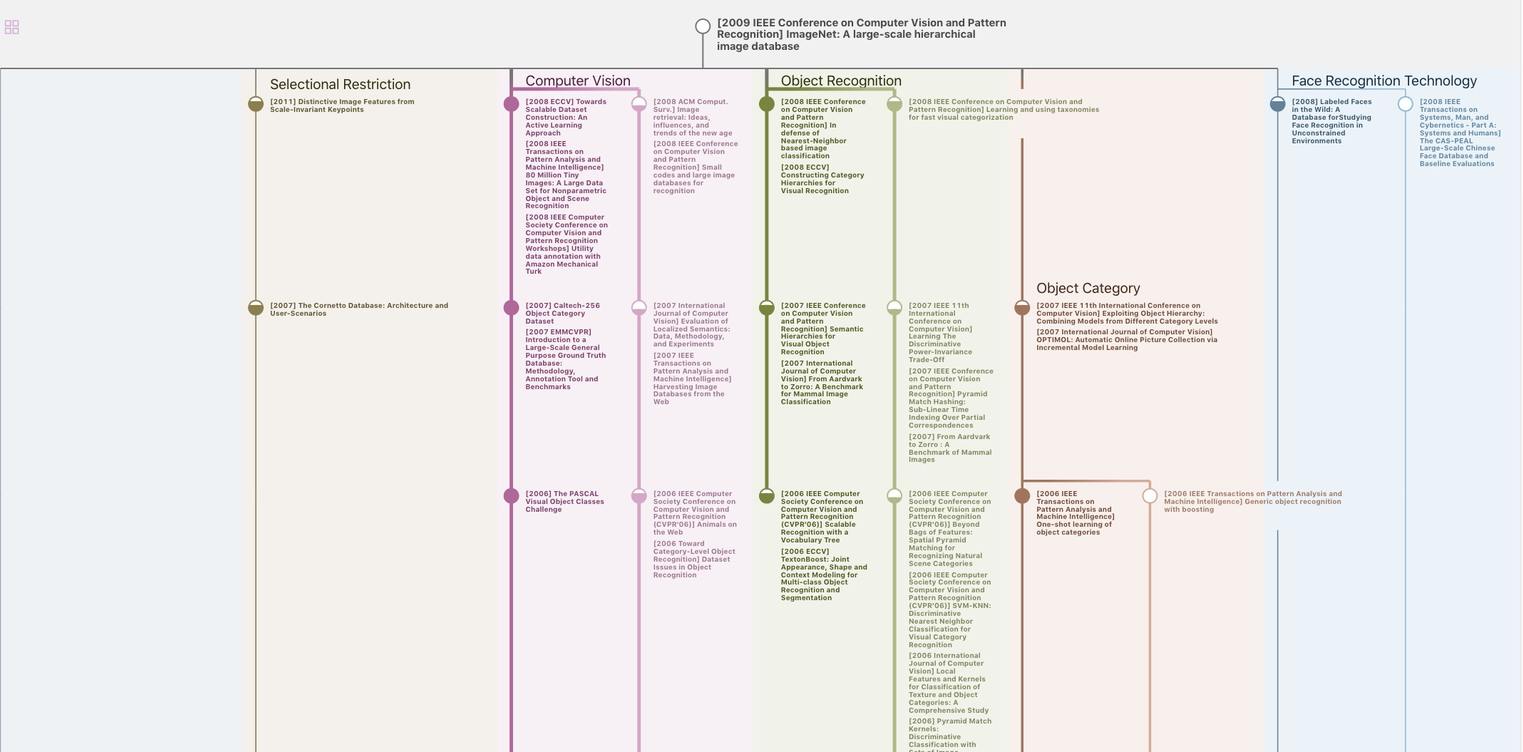
生成溯源树,研究论文发展脉络
Chat Paper
正在生成论文摘要