Real-time resource scaling platform for Big Data workloads on serverless environments.
Future Generation Computer Systems(2020)
摘要
The serverless execution paradigm is becoming an increasingly popular option when workloads are to be deployed in an abstracted way, more specifically, without specifying any infrastructure requirements. Currently, such workloads are typically comprised of small programs or even a series of single functions used as event triggers or to process a data stream. Other applications that may also fit on a serverless scenario are stateless services that may need to seamlessly scale in terms of resources, such as a web server. Although several commercial serverless services are available (e.g., Amazon Lambda), their use cases are mostly limited to the execution of functions or scripts that can be adapted to predefined templates or specifications. However, current research efforts point out that it is interesting for the serverless paradigm to evolve from single functions and support more flexible infrastructure units such as operating-system-level virtualization in the form of containers. In this paper we present a novel platform to automatically scale container resources in real time, while they are running, and without any need for reboots. This platform is evaluated using Big Data workloads, both batch and streaming, as representative examples of applications that could be initially regarded as unsuitable for the serverless paradigm considering the currently available services. The results show how our serverless platform can improve the CPU utilization by up to 77% with an execution time overhead of only 6%, while remaining scalable when using a 32-container cluster.
更多查看译文
关键词
Serverless computing,Big Data,Resource scaling,Operating-system-level virtualization,Container cluster
AI 理解论文
溯源树
样例
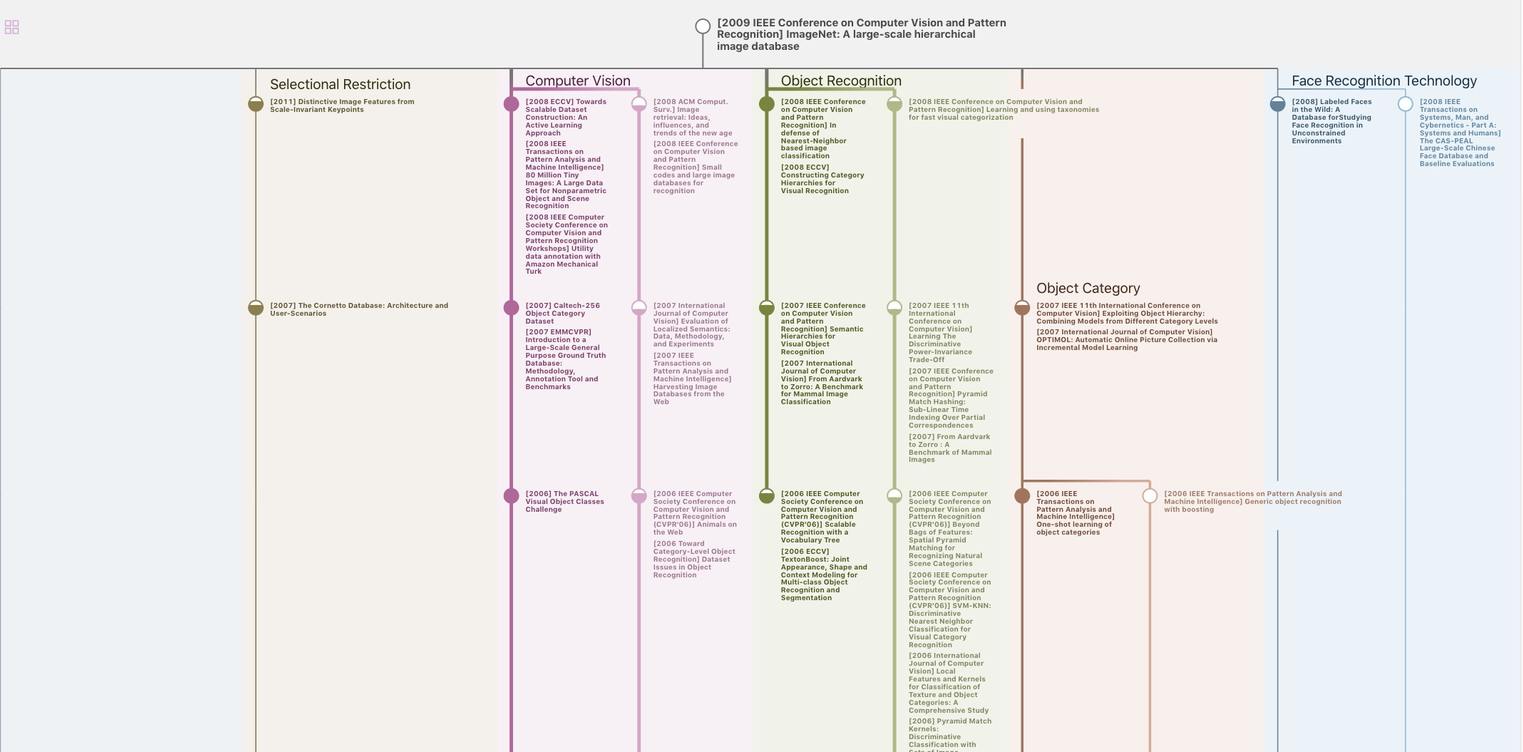
生成溯源树,研究论文发展脉络
Chat Paper
正在生成论文摘要