An adaptive sparse Bayesian model combined with probabilistic label fusion for multiple sclerosis lesion segmentation in brain MRI.
Future Generation Computer Systems(2020)
摘要
This paper presents a novel method for automatic segmentation of multiple sclerosis (MS) lesion in brain magnetic resonance (MR) image. The first research directly focuses on the development of an algorithm called the adaptive sparse Bayesian decision theorem (ASBDT), which integrates Markov random field theorem and Gibbs random field theorem to segment MS lesion in T1-w, T2-w and FLAIR images, respectively. The second research focus is based on a probabilistic label fusion (PLF) algorithm, which utilizes a set of prior known multi-atlas obtained from T1-w, T2-w and FLAIR images, respectively. The PLF algorithm is based on local weighted voting strategy, in which a specific coefficient obtained using the maximum likelihood function between the prior known atlas and the target image is assigned as the weight to individual atlas. The proposed segmentation approach is evaluated by 20 brain MR image data sets from the MICCAI MS Lesion Segmentation Challenge. The values of evaluation parameters TPR, PPV, DSC indicate that our approach is better than some other methods. According to the McDonald Criteria updated in 2017, the number and volume is a key point in defining the disease, we calculate the total MS lesion volume and number. Therefore, this novel approach has an added value for the clinical evaluation of MS lesion patients.
更多查看译文
关键词
Multiple sclerosis lesion segmentation,Sparse Bayesian decision theorem,Markov random field,Gibbs random field,Label fusion
AI 理解论文
溯源树
样例
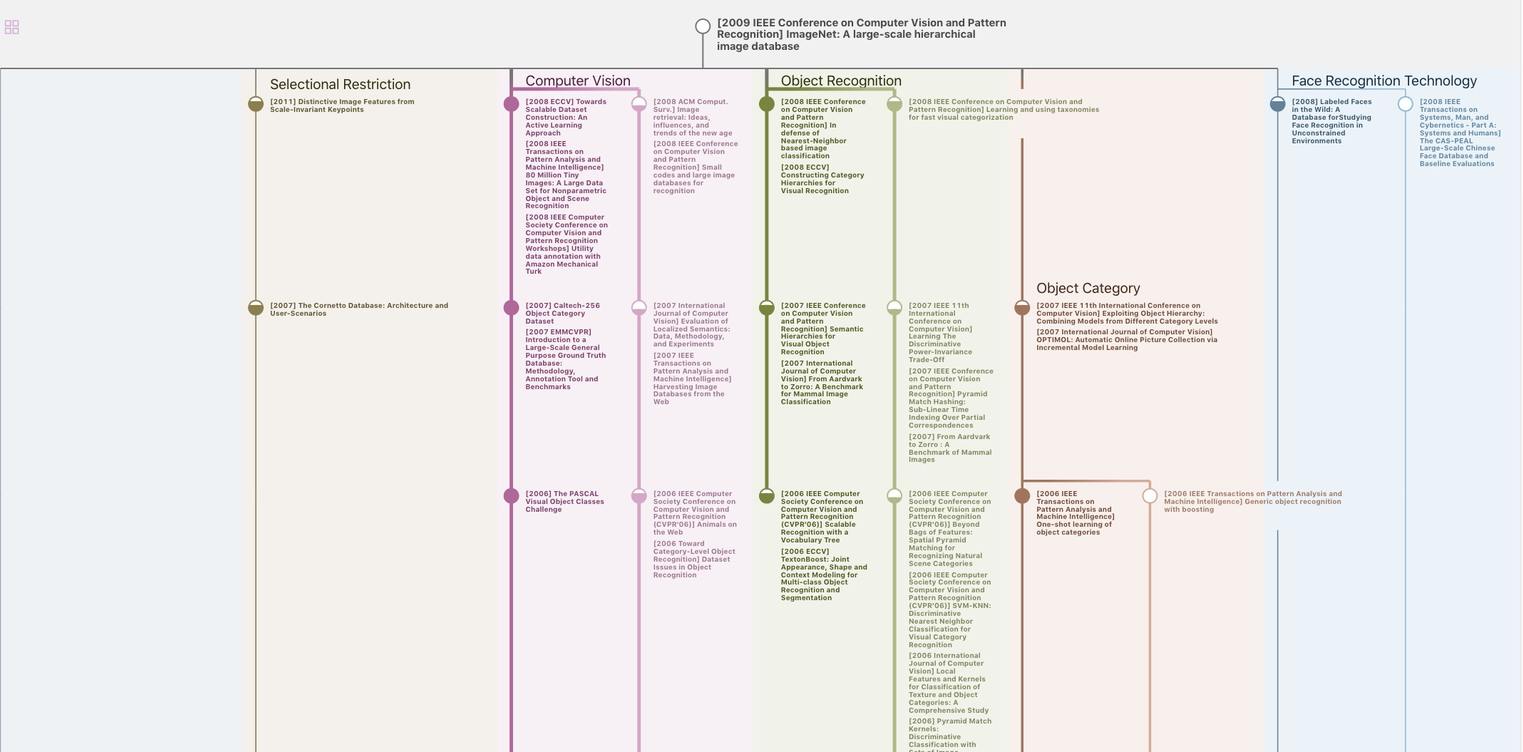
生成溯源树,研究论文发展脉络
Chat Paper
正在生成论文摘要