Finding structure in multi-armed bandits.
Cognitive Psychology(2020)
摘要
How do humans search for rewards? This question is commonly studied using multi-armed bandit tasks, which require participants to trade off exploration and exploitation. Standard multi-armed bandits assume that each option has an independent reward distribution. However, learning about options independently is unrealistic, since in the real world options often share an underlying structure. We study a class of structured bandit tasks, which we use to probe how generalization guides exploration. In a structured multi-armed bandit, options have a correlation structure dictated by a latent function. We focus on bandits in which rewards are linear functions of an option’s spatial position. Across 5 experiments, we find evidence that participants utilize functional structure to guide their exploration, and also exhibit a learning-to-learn effect across rounds, becoming progressively faster at identifying the latent function. Our experiments rule out several heuristic explanations and show that the same findings obtain with non-linear functions. Comparing several models of learning and decision making, we find that the best model of human behavior in our tasks combines three computational mechanisms: (1) function learning, (2) clustering of reward distributions across rounds, and (3) uncertainty-guided exploration. Our results suggest that human reinforcement learning can utilize latent structure in sophisticated ways to improve efficiency.
更多查看译文
关键词
Learning,Decision making,Reinforcement learning,Function learning,Exploration-exploitation,Learning-to-learn,Generalization,Gaussian process,Structure learning,Latent structure
AI 理解论文
溯源树
样例
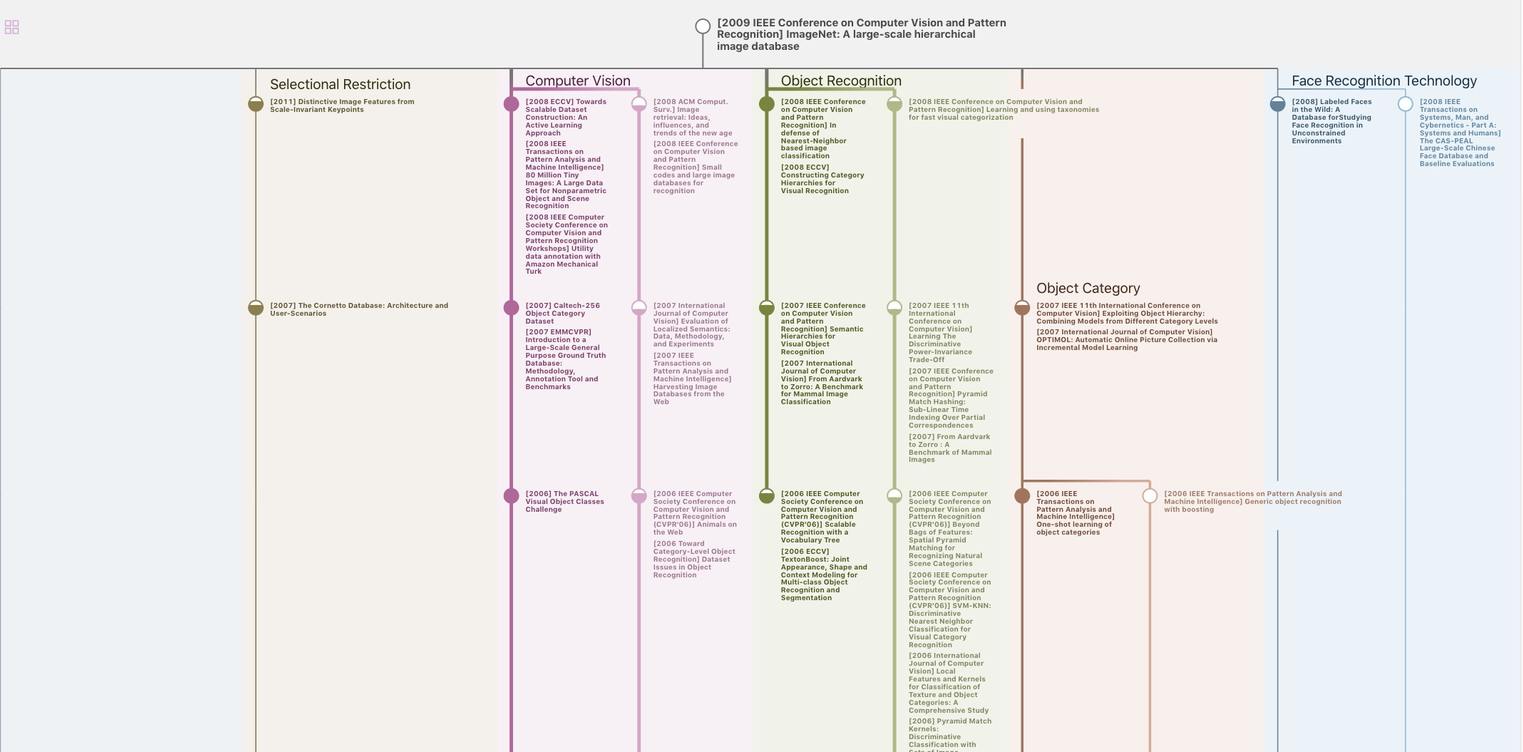
生成溯源树,研究论文发展脉络
Chat Paper
正在生成论文摘要