Estimating Gradients for Discrete Random Variables by Sampling without Replacement
ICLR(2020)
摘要
We derive an unbiased estimator for expectations over discrete random variables based on sampling without replacement, which reduces variance as it avoids duplicate samples. We show that our estimator can be derived as the Rao-Blackwellization of three different estimators. Combining our estimator with REINFORCE, we obtain a policy gradient estimator and we reduce its variance using a built-in control variate which is obtained without additional model evaluations. The resulting estimator is closely related to other gradient estimators. Experiments with a toy problem, a categorical Variational Auto-Encoder and a structured prediction problem show that our estimator is the only estimator that is consistently among the best estimators in both high and low entropy settings.
更多查看译文
关键词
gradient, estimator, discrete, categorical, sampling, without replacement, reinforce, baseline, variance, gumbel, vae, structured prediction
AI 理解论文
溯源树
样例
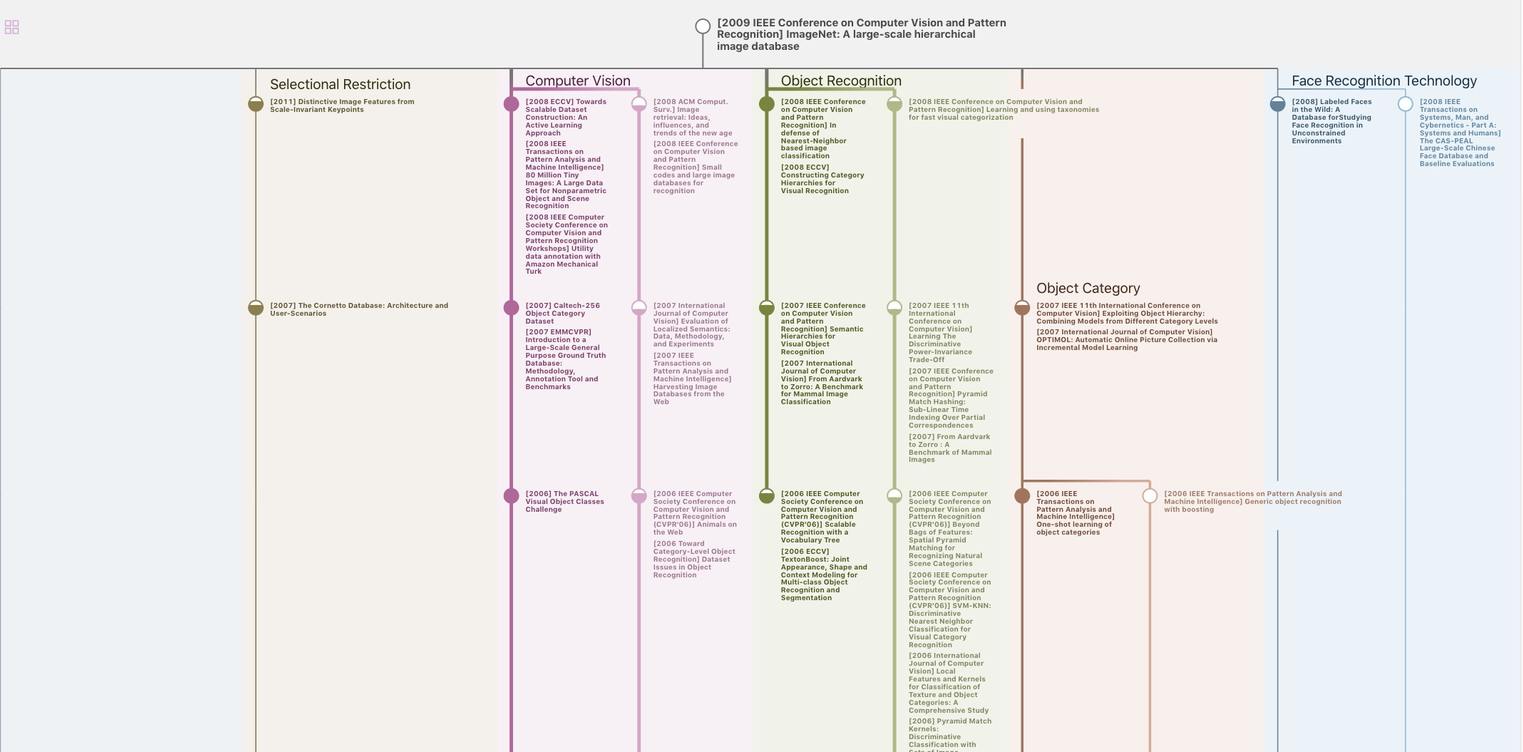
生成溯源树,研究论文发展脉络
Chat Paper
正在生成论文摘要