An Adaptive X-Vector Model for Text-Independent Speaker Verification.
arxiv(2020)
摘要
In this paper, adaptive mechanisms are applied in deep neural network (DNN) training for x-vector-based text-independent speaker verification. First, adaptive convolutional neural networks (ACNNs) are employed in frame-level embedding layers, where the parameters of the convolution filters are adjusted based on the input features. Compared with conventional CNNs, ACNNs have more flexibility in capturing speaker information. Moreover, we replace conventional batch normalization (BN) with adaptive batch normalization (ABN). By dynamically generating the scaling and shifting parameters in BN, ABN adapts models to the acoustic variability arising from various factors such as channel and environmental noises. Finally, we incorporate these two methods to further improve performance. Experiments are carried out on the speaker in the wild (SITW) and VOiCES databases. The results demonstrate that the proposed methods significantly outperform the original x-vector approach.
更多查看译文
关键词
Speaker verification, Adaptive convolution, Adaptive batch normalization, Attention mechanism
AI 理解论文
溯源树
样例
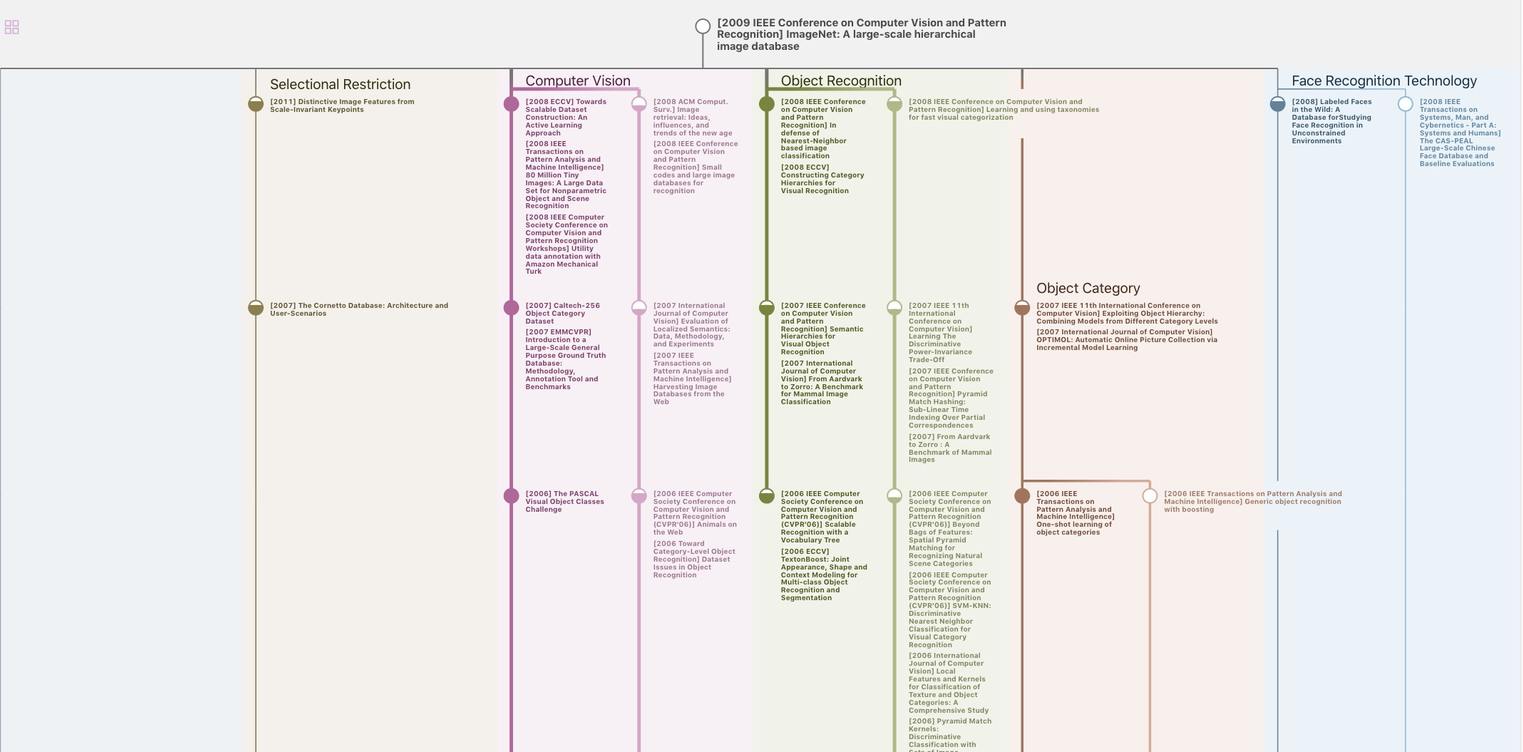
生成溯源树,研究论文发展脉络
Chat Paper
正在生成论文摘要