Gene Regulatory Network Inference with Evolution Strategies and Sparse Matrix Representation.
IEEE International Conference on Bioinformatics and Biomedicine(2019)
摘要
Gene Regulatory Networks (GRNs) represent the functional interactions between genes, such as the expression of one gene enhancing the subsequent expression of another gene. GRNs can be inferred from observations of the expressions of various genes over time. This task is difficult, due to the complex interactions among collections of genes, as well as the inherent noise in the observable data. In realistic genomes with thousands of genes, millions of gene-pairs exist, although the actual number of possible interactions may be considerably smaller, since each gene may affect only a small number of other genes. This motivates the application of sparse learning algorithms for GRN inference. In particular, we explore evolutionary algorithms, and their applications with sparse matrix representations. Our approach can speed up the optimization process and find good solutions, uncovering the underlying GRNs. We study evolution strategies, particle swarm optimization, and a greedy algorithm, and compare their performance in terms of solution quality and computational effort required for GRN inference.
更多查看译文
关键词
gene regulatory network,evolution strategies,evolutionary algorithms,sparse learning
AI 理解论文
溯源树
样例
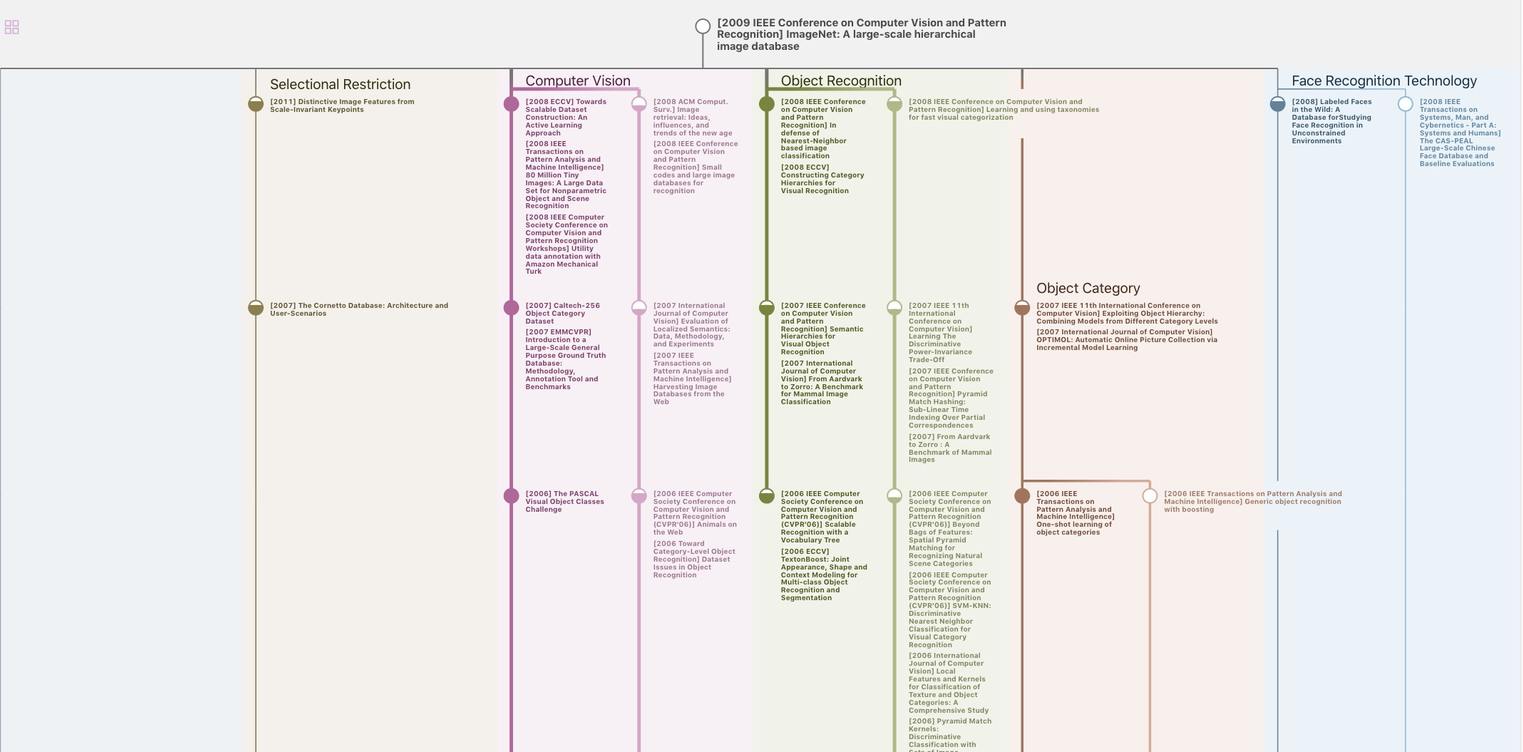
生成溯源树,研究论文发展脉络
Chat Paper
正在生成论文摘要