A New Approach Of Applying Deep Learning To Protein Model Quality Assessment
2019 IEEE INTERNATIONAL CONFERENCE ON BIOINFORMATICS AND BIOMEDICINE (BIBM)(2019)
摘要
Computational protein structure prediction is an important problem in bioinformatics. In recent years, several deep learning methods have been developed for protein 3D model quality assessment (QA) and achieved promising results. In this paper, we propose a new approach of applying deep learning to the QA problem, called DeepPTQA: training one customized deep learning network for each target protein whose models are to be evaluated, i.e. the per-target training approach. For each target protein, a deep neural network is first trained using a labeled training set created based on templates of the target protein, and then applied to evaluate the quality of models of the target protein. We implemented this approach using new deep inception networks based on templates of the target protein. To predict the quality of a protein model, the deep networks use an extensive set of 1-D and 2-D features of the target protein and the target model, including predicted and real secondary structures, solvent accessibility, distance matrix, co-evolutionary information, to predict the model's true GDT-TS score, a widely used quality score in the range of [0, 1]. Experimental results using CASP targets show that DeepPTQA outperformed the best existing QA methods on the CASP Stage 2 QA task.
更多查看译文
关键词
Inception Network, Deep learning, Protein Model Quality Assessment
AI 理解论文
溯源树
样例
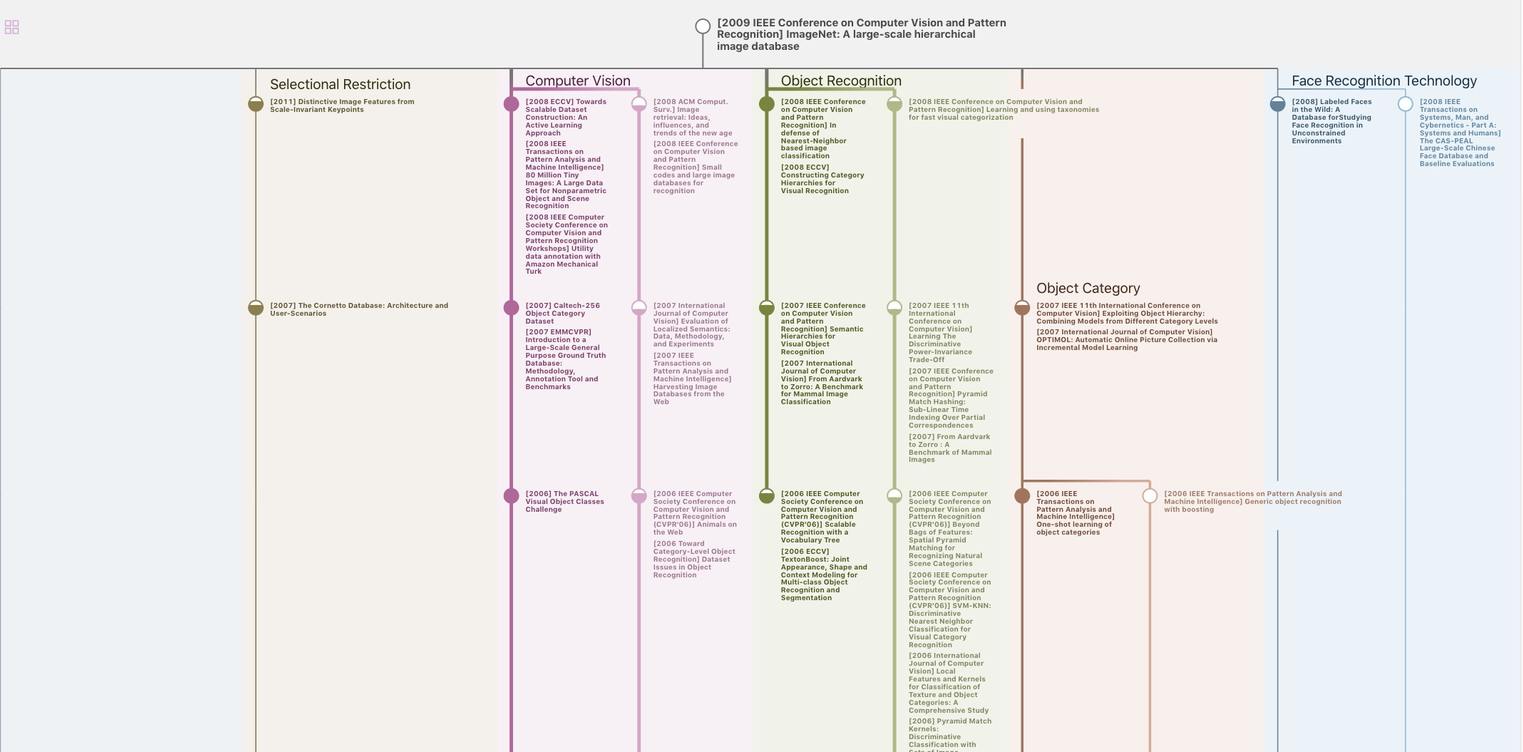
生成溯源树,研究论文发展脉络
Chat Paper
正在生成论文摘要