Learning Not to Learn in the Presence of Noisy Labels
arXiv (Cornell University)(2020)
摘要
Learning in the presence of label noise is a challenging yet important task: it is crucial to design models that are robust in the presence of mislabeled datasets. In this paper, we discover that a new class of loss functions called the gambler's loss provides strong robustness to label noise across various levels of corruption. We show that training with this loss function encourages the model to "abstain" from learning on the data points with noisy labels, resulting in a simple and effective method to improve robustness and generalization. In addition, we propose two practical extensions of the method: 1) an analytical early stopping criterion to approximately stop training before the memorization of noisy labels, as well as 2) a heuristic for setting hyperparameters which do not require knowledge of the noise corruption rate. We demonstrate the effectiveness of our method by achieving strong results across three image and text classification tasks as compared to existing baselines.
更多查看译文
关键词
noisy labels,learning
AI 理解论文
溯源树
样例
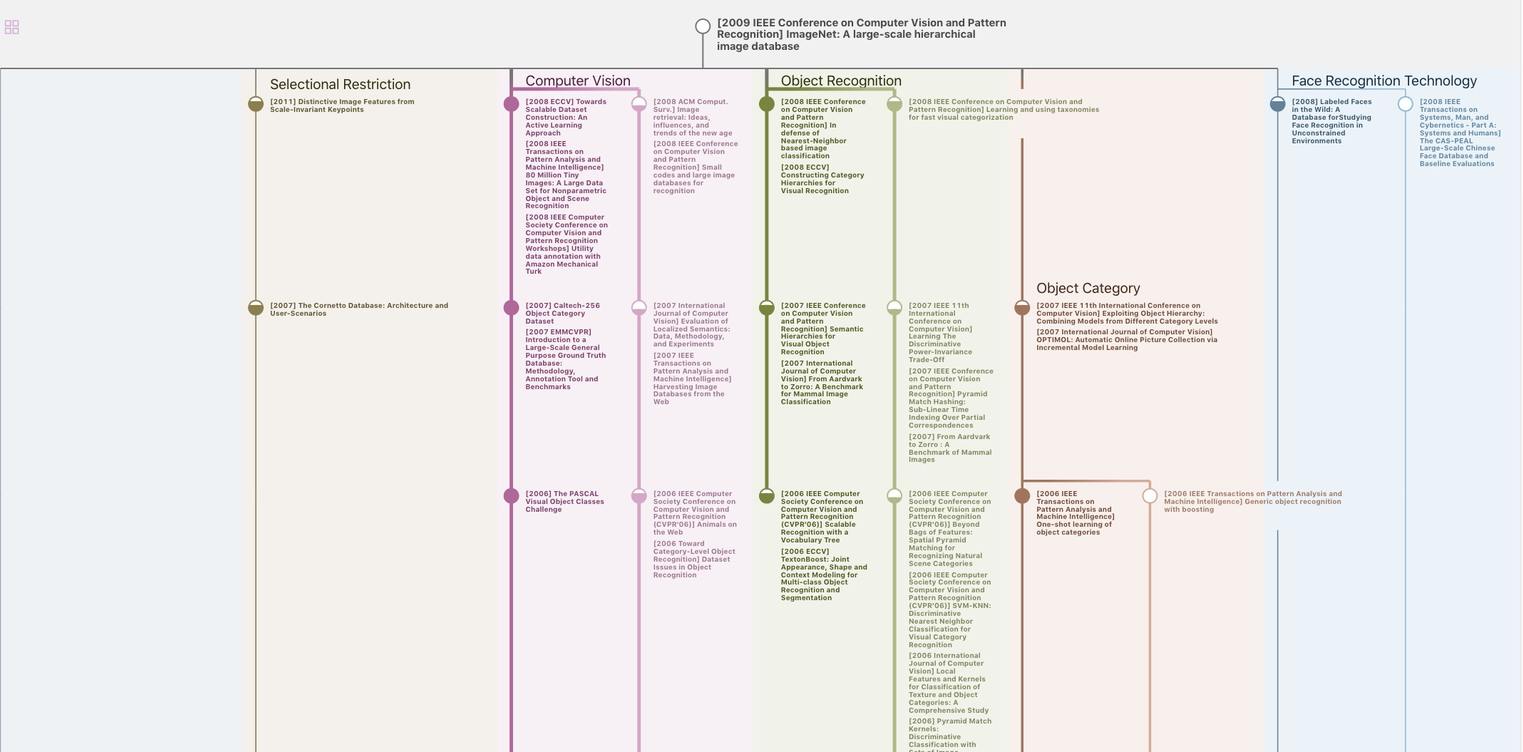
生成溯源树,研究论文发展脉络
Chat Paper
正在生成论文摘要