Modeling Citation Trajectories of Scientific Papers.
PAKDD (2)(2020)
摘要
Several network growth models have been proposed in the literature that attempt to incorporate properties of citation networks. Generally, these models aim at retaining the degree distribution observed in real-world networks. In this work, we explore whether existing network growth models can realize the diversity in citation growth exhibited by individual papers – a new node-centric property observed recently in citation networks across multiple domains of research. We theoretically and empirically show that the network growth models which are solely based on degree and/or intrinsic fitness cannot realize certain temporal growth behaviors that are observed in real-world citation networks. To this end, we propose two new growth models that localize the influence of papers through an appropriate attachment mechanism. Experimental results on the real-world citation networks of Computer Science and Physics domains show that our proposed models can better explain the temporal behavior of citation networks than existing models.
更多查看译文
关键词
citation trajectories,papers,scientific
AI 理解论文
溯源树
样例
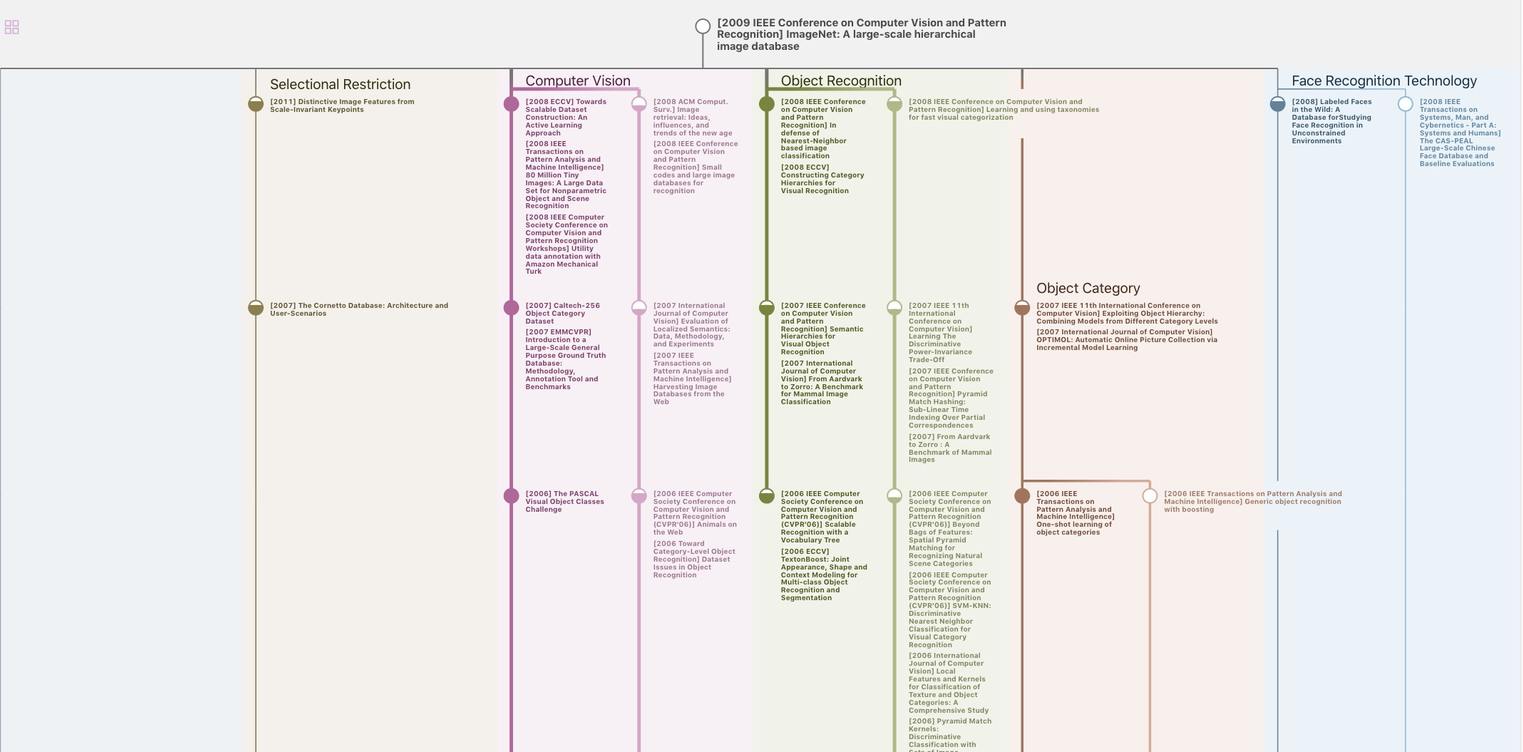
生成溯源树,研究论文发展脉络
Chat Paper
正在生成论文摘要