A Stein Goodness-of-fit Test for Directional Distributions
INTERNATIONAL CONFERENCE ON ARTIFICIAL INTELLIGENCE AND STATISTICS, VOL 108(2020)
摘要
In many fields, data appears in the form of direction (unit vector) and usual statistical procedures are not applicable to such directional data. In this study, we propose non-parametric goodness-of-fit testing procedures for general directional distributions based on kernel Stein discrepancy. Our method is based on Stein's operator on spheres, which is derived by using Stokes' theorem. Notably, the proposed method is applicable to distributions with an intractable normalization constant, which commonly appear in directional statistics. Experimental results demonstrate that the proposed methods control type-I error well and have larger power than existing tests, including the test based on the maximum mean discrepancy.
更多查看译文
关键词
goodness-of-fit
AI 理解论文
溯源树
样例
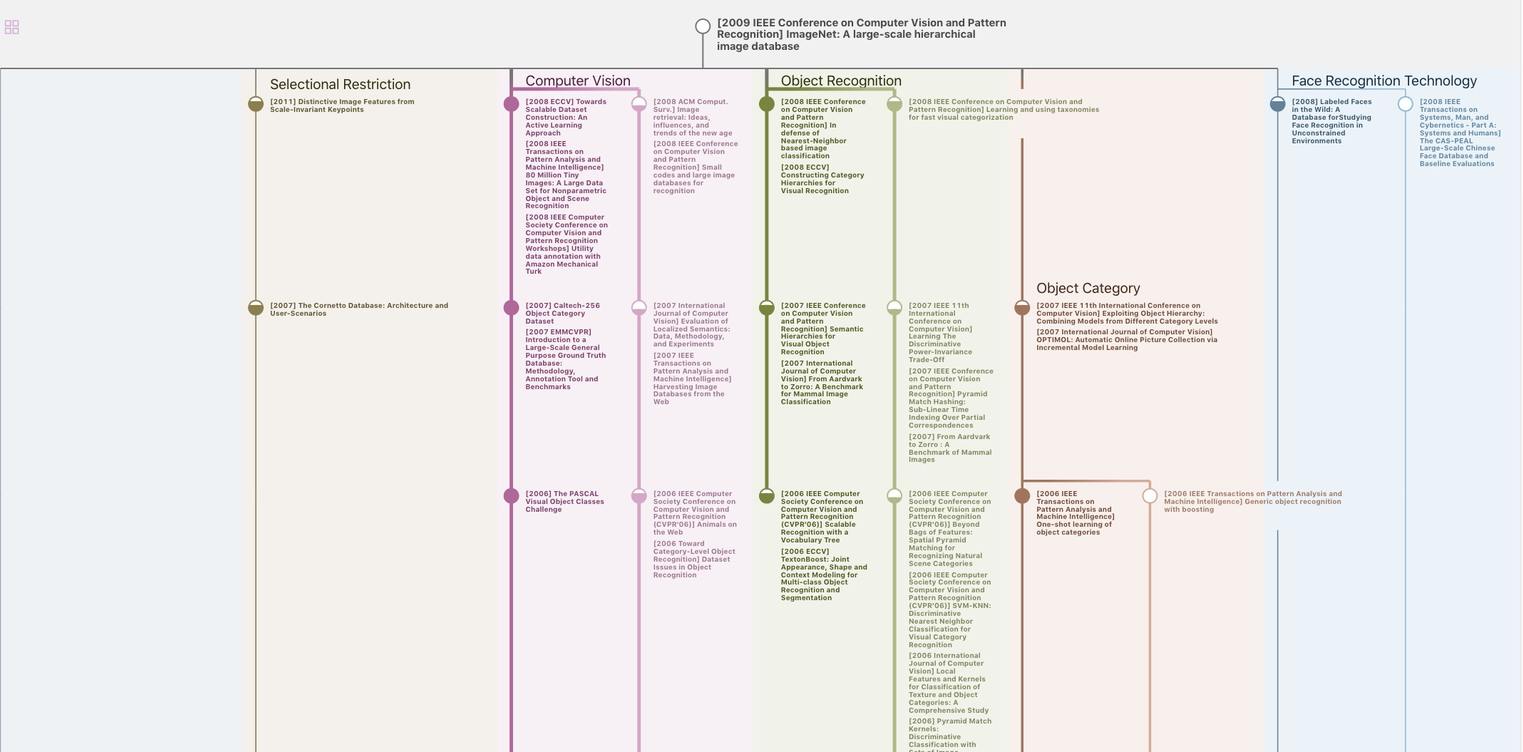
生成溯源树,研究论文发展脉络
Chat Paper
正在生成论文摘要