Block-Approximated Exponential Random Graphs
2020 IEEE 7th International Conference on Data Science and Advanced Analytics (DSAA)(2020)
摘要
An important challenge in the field of exponential random graphs (ERGs) is the fitting of non-trivial ERGs on large graphs. By utilizing fast matrix block-approximation techniques, we propose an approximative framework to such non-trivial ERGs that result in dyadic independence (i.e., edge independent) distributions, while being able to meaningfully model local information of the graph (e.g., degrees) as well as global information (e.g., clustering coefficient, assortativity, etc.) if desired. This allows one to efficiently generate random networks with similar properties as an observed network, and the models can be used for several downstream tasks such as link prediction. Our methods are scalable to sparse graphs consisting of millions of nodes. Empirical evaluation demonstrates competitiveness in terms of both speed and accuracy with state-of-the-art methods-which are typically based on embedding the graph into some low-dimensional space- for link prediction, showcasing the potential of a more direct and interpretable probablistic model for this task.
更多查看译文
关键词
maximum entropy models,exponential random graphs,network modeling,link prediction
AI 理解论文
溯源树
样例
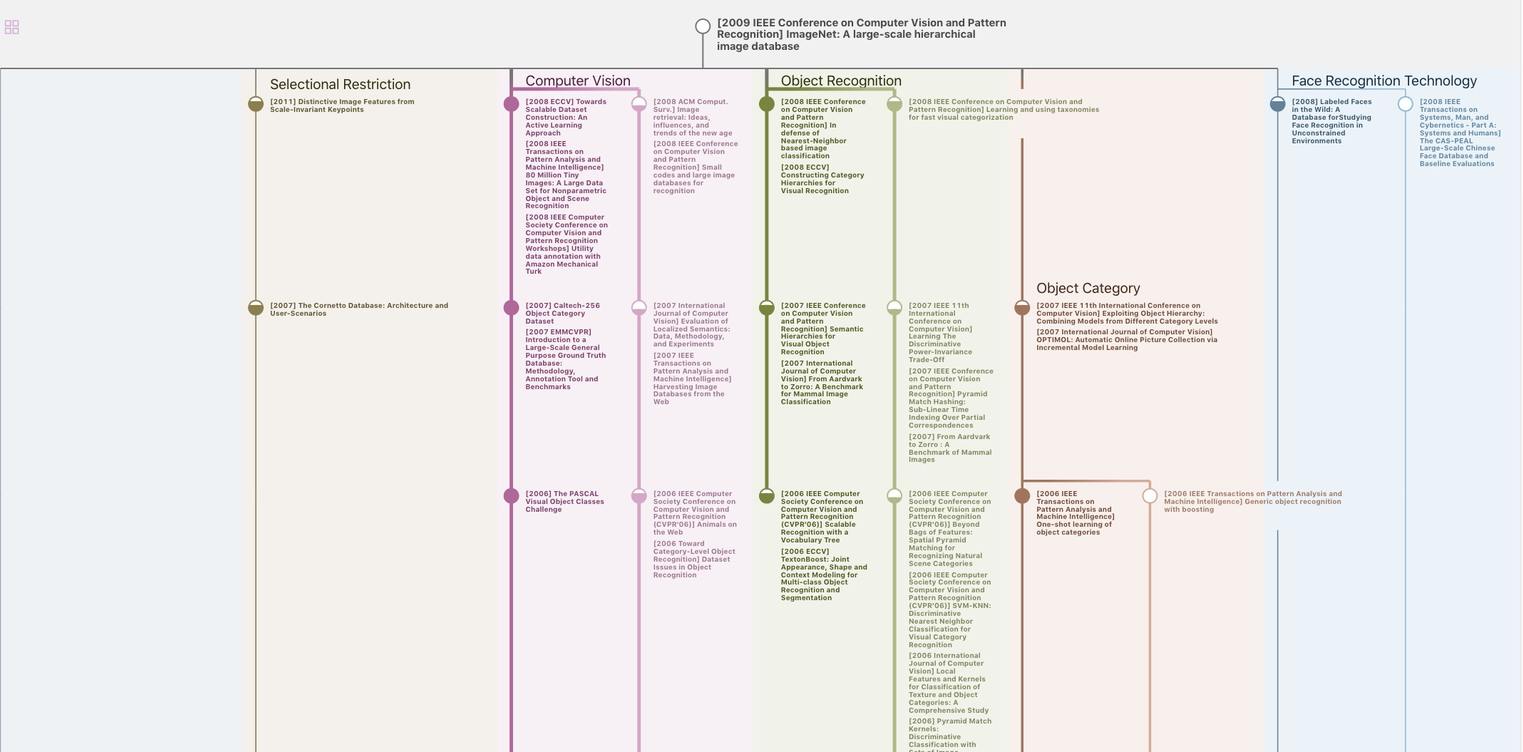
生成溯源树,研究论文发展脉络
Chat Paper
正在生成论文摘要