Distributed adaptive Newton methods with global superlinear convergence
AUTOMATICA(2022)
摘要
This paper considers the distributed optimization problem where each node of a peer-to-peer network minimizes a finite sum of objective functions by communicating with its neighboring nodes. In sharp contrast to the existing literature where the fastest distributed algorithms converge either with a global linear or a local superlinear rate, we propose a distributed adaptive Newton (DAN) algorithm with a global quadratic convergence rate. Our key idea lies in the design of a finite-time set-consensus method with Polyak's adaptive stepsize. Moreover, we introduce a low-rank matrix approximation (LA) technique to compress the innovation of Hessian matrix so that each node only needs to transmit message of dimension O(p) (where p is the dimension of decision vectors) per iteration, which is essentially the same as that of first-order methods. Nevertheless, the resulting DAN-LA converges to an optimal solution with a global superlinear rate. Numerical experiments on logistic regression problems are conducted to validate their advantages over existing methods. (C)& nbsp;2022 Elsevier Ltd. All rights reserved.
更多查看译文
关键词
Distributed optimization, Newton method, Low-rank approximation, Superlinear convergence
AI 理解论文
溯源树
样例
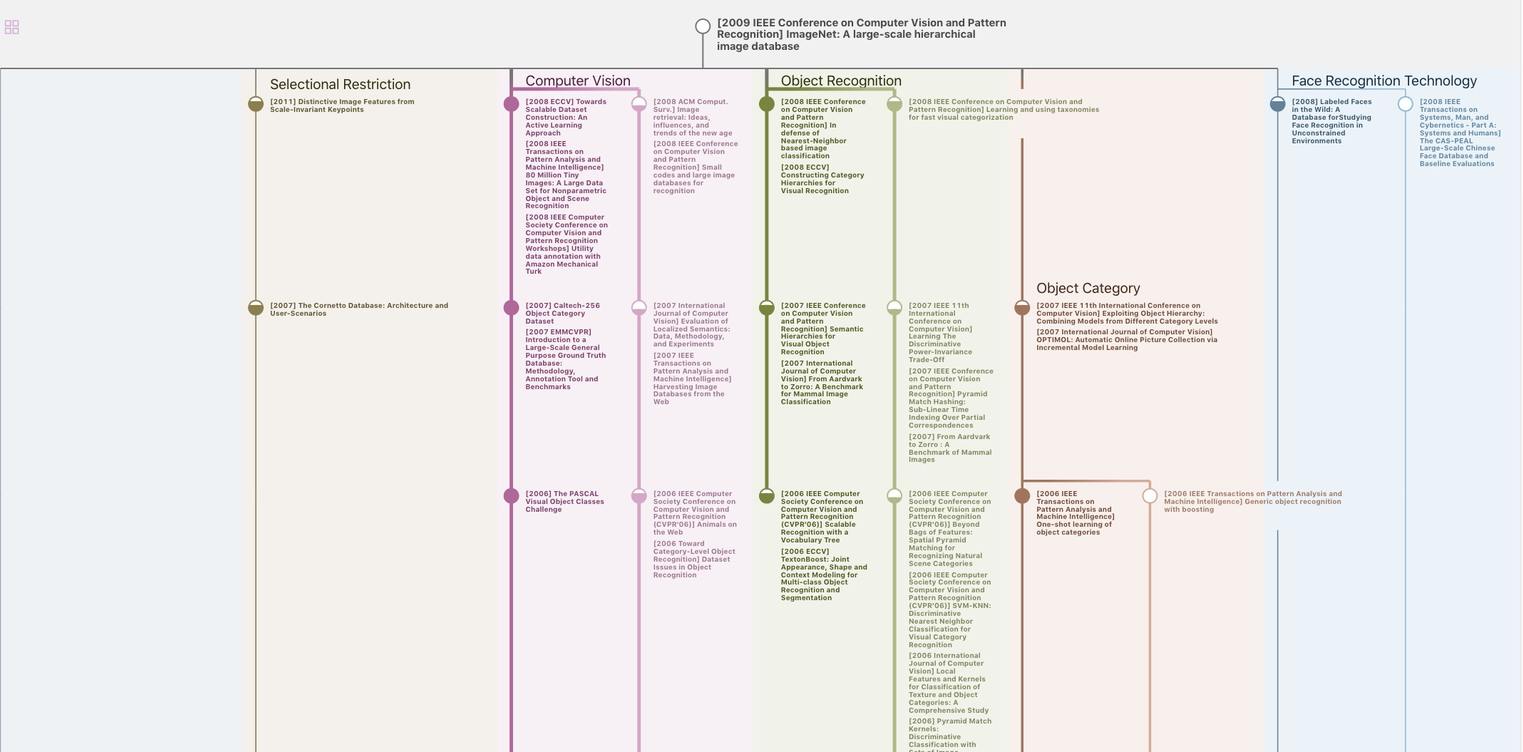
生成溯源树,研究论文发展脉络
Chat Paper
正在生成论文摘要