A Wasserstein Minimum Velocity Approach to Learning Unnormalized Models
INTERNATIONAL CONFERENCE ON ARTIFICIAL INTELLIGENCE AND STATISTICS, VOL 108(2020)
摘要
Score matching provides an effective approach to learning flexible unnormalized models, but its scalability is limited by the need to evaluate a second-order derivative. In this paper, we present a scalable approximation to a general family of learning objectives including score matching, by observing a new connection between these objectives and Wasserstein gradient flows. We present applications with promise in learning neural density estimators on manifolds, and training implicit variational and Wasserstein auto-encoders with a manifold-valued prior.
更多查看译文
关键词
wasserstein minimum velocity approach,models,learning
AI 理解论文
溯源树
样例
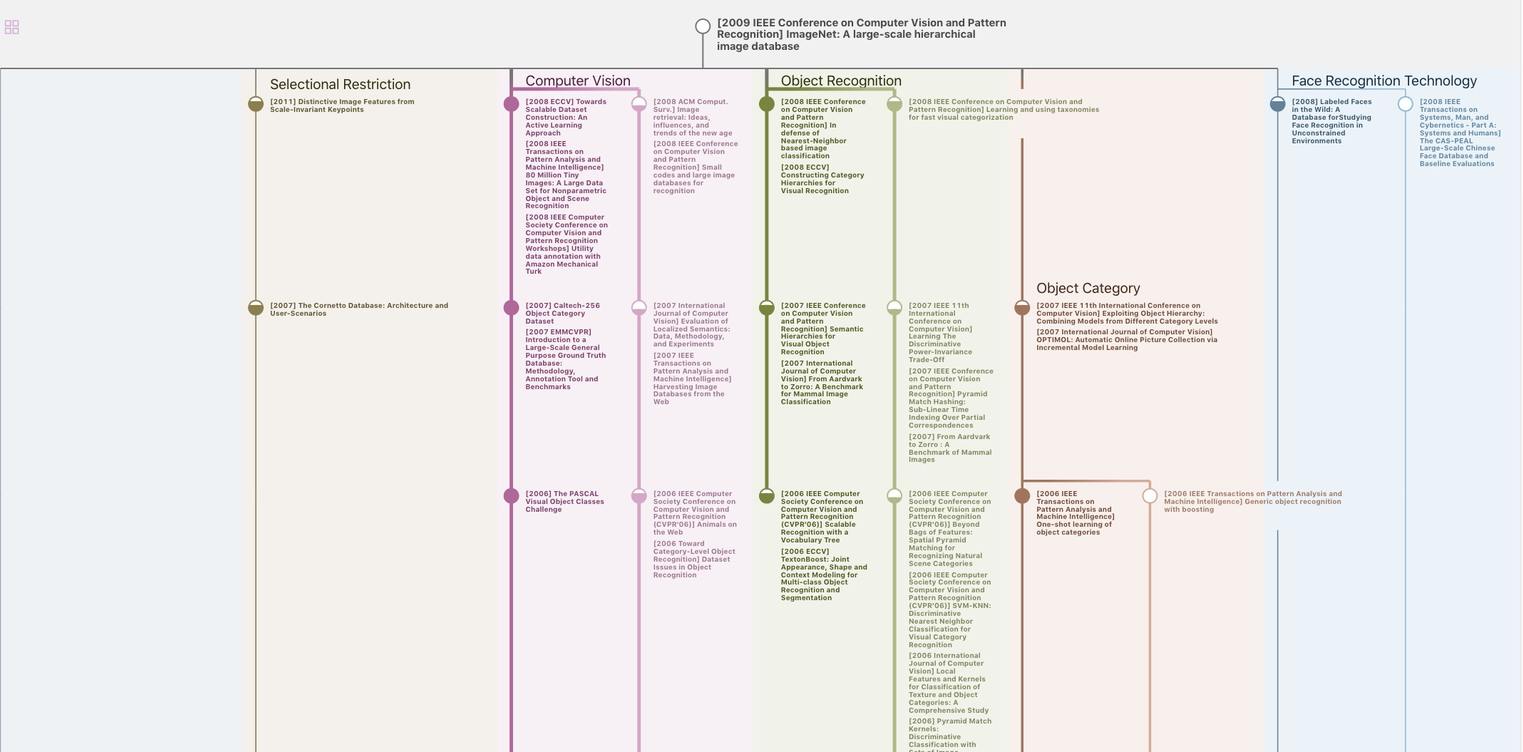
生成溯源树,研究论文发展脉络
Chat Paper
正在生成论文摘要