Deep Neural Networks for the Correction of Mie Scattering in Fourier-Transformed Infrared Spectra of Biological Samples
arXiv (Cornell University)(2020)
摘要
Infrared spectra obtained from cell or tissue specimen have commonly been observed to involve a significant degree of (resonant) Mie scattering, which often overshadows biochemically relevant spectral information by a non-linear, non-additive spectral component in Fourier transformed infrared (FTIR) spectroscopic measurements. Correspondingly, many successful machine learning approaches for FTIR spectra have relied on preprocessing procedures that computationally remove the scattering components from an infrared spectrum. We propose an approach to approximate this complex preprocessing function using deep neural networks. As we demonstrate, the resulting model is not just several orders of magnitudes faster, which is important for real-time clinical applications, but also generalizes strongly across different tissue types. Furthermore, our proposed method overcomes the trade-off between computation time and the corrected spectrum being biased towards an artificial reference spectrum.
更多查看译文
关键词
Infrared Spectroscopy,Near-Infrared Spectroscopy,Hyperspectral Imaging,Spectral Analysis,NMR Spectroscopy
AI 理解论文
溯源树
样例
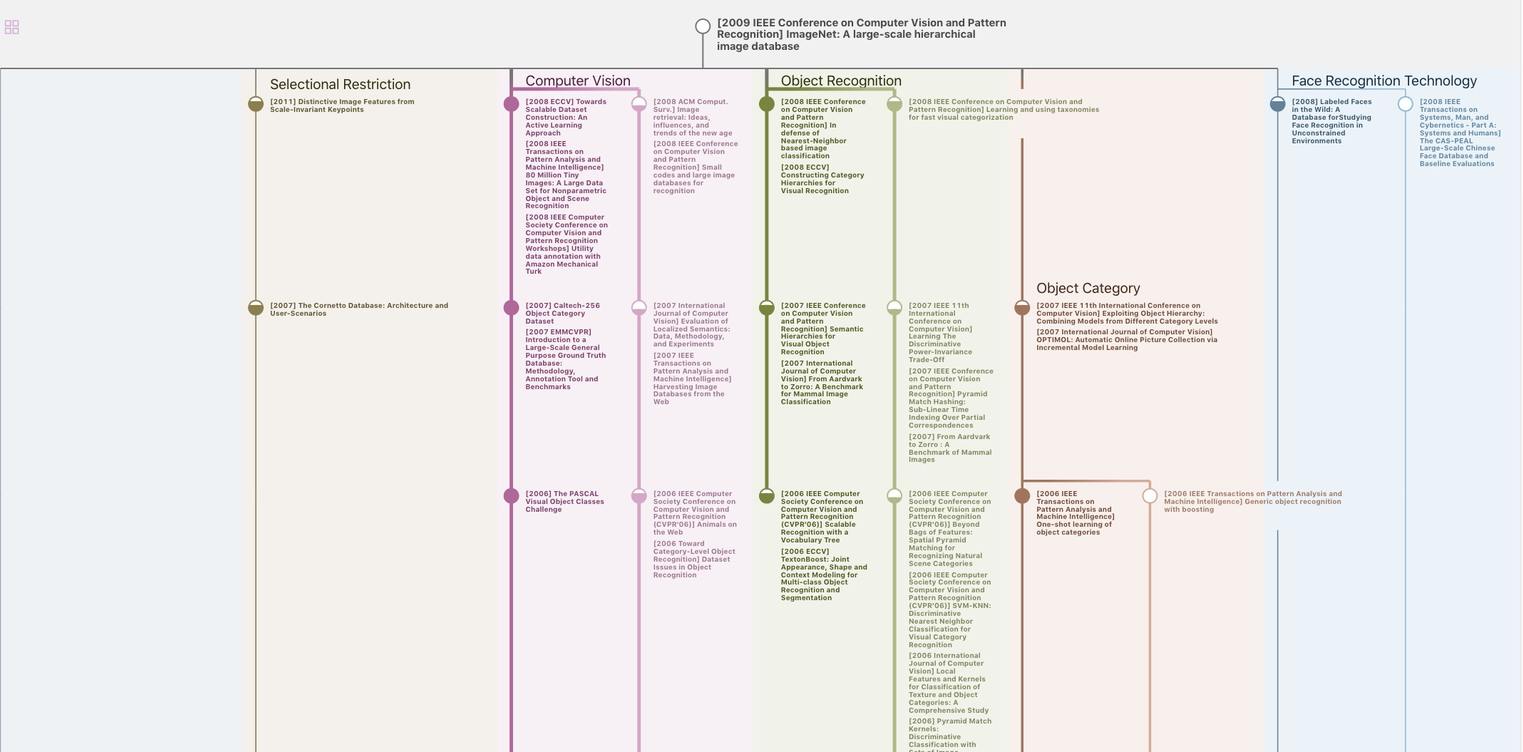
生成溯源树,研究论文发展脉络
Chat Paper
正在生成论文摘要