Robust Quantization: One Model to Rule Them All
NIPS 2020(2020)
摘要
Neural network quantization methods often involve simulating the quantization process during training. This makes the trained model highly dependent on the precise way quantization is performed. Since low-precision accelerators differ in their quantization policies and their supported mix of data-types, a model trained for one accelerator may not be suitable for another. To address this issue, we propose KURE, a method that provides intrinsic robustness to the model against a broad range of quantization implementations. We show that KURE yields a generic model that may be deployed on numerous inference accelerators without a significant loss in accuracy.
更多查看译文
关键词
robust quantization,model
AI 理解论文
溯源树
样例
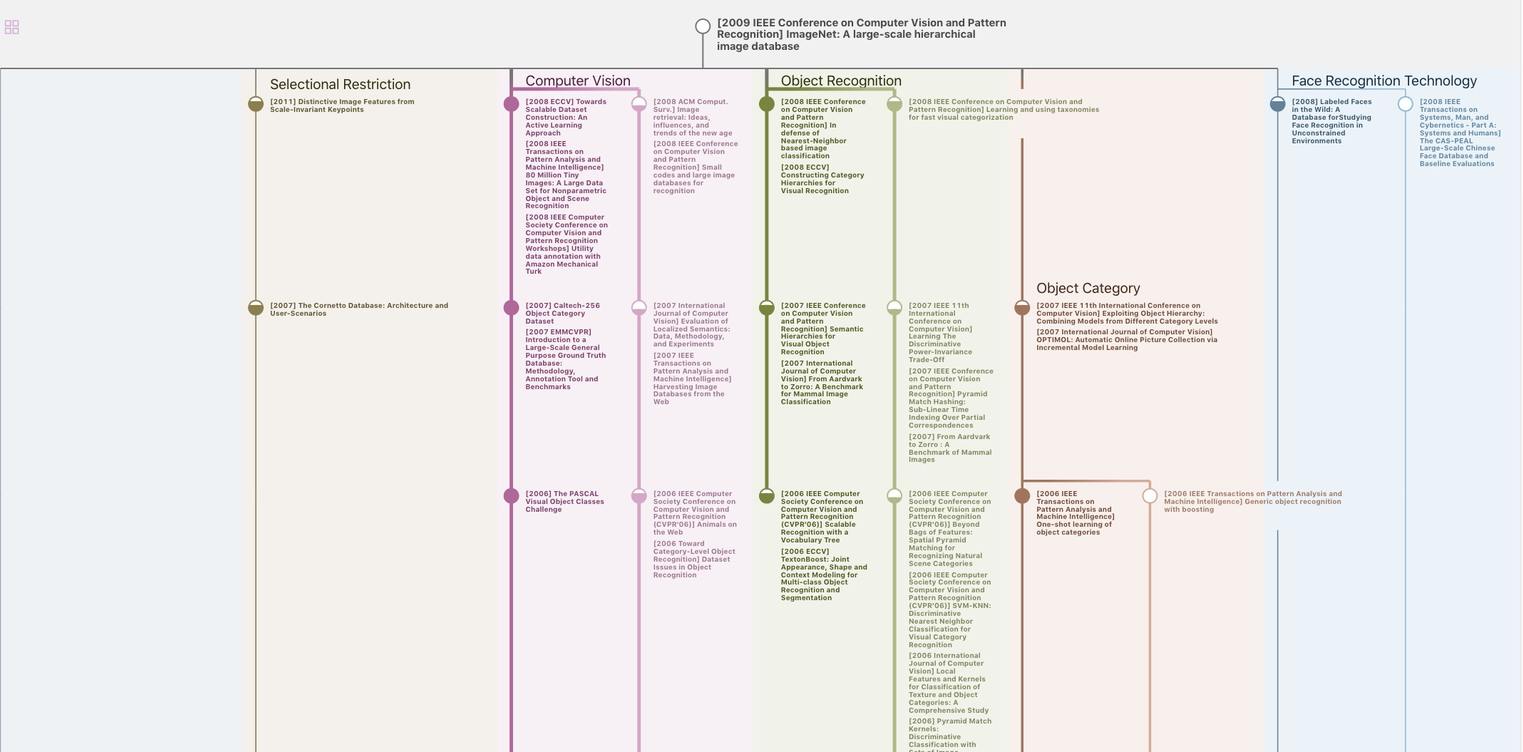
生成溯源树,研究论文发展脉络
Chat Paper
正在生成论文摘要