Learning Bijective Feature Maps for Linear ICA.
AISTATS(2021)
摘要
Separating high-dimensional data like images into independent latent factors remains an open research problem. Here we develop a method that jointly learns a linear independent component analysis (ICA) model with non-linear bijective feature maps. By combining these two methods, ICA can learn interpretable latent structure for images. For non-square ICA, where we assume the number of sources is less than the dimensionality of data, we achieve better unsupervised latent factor discovery than flow-based models and linear ICA. This performance scales to large image datasets such as CelebA
更多查看译文
关键词
bijective feature maps,linear ica,learning
AI 理解论文
溯源树
样例
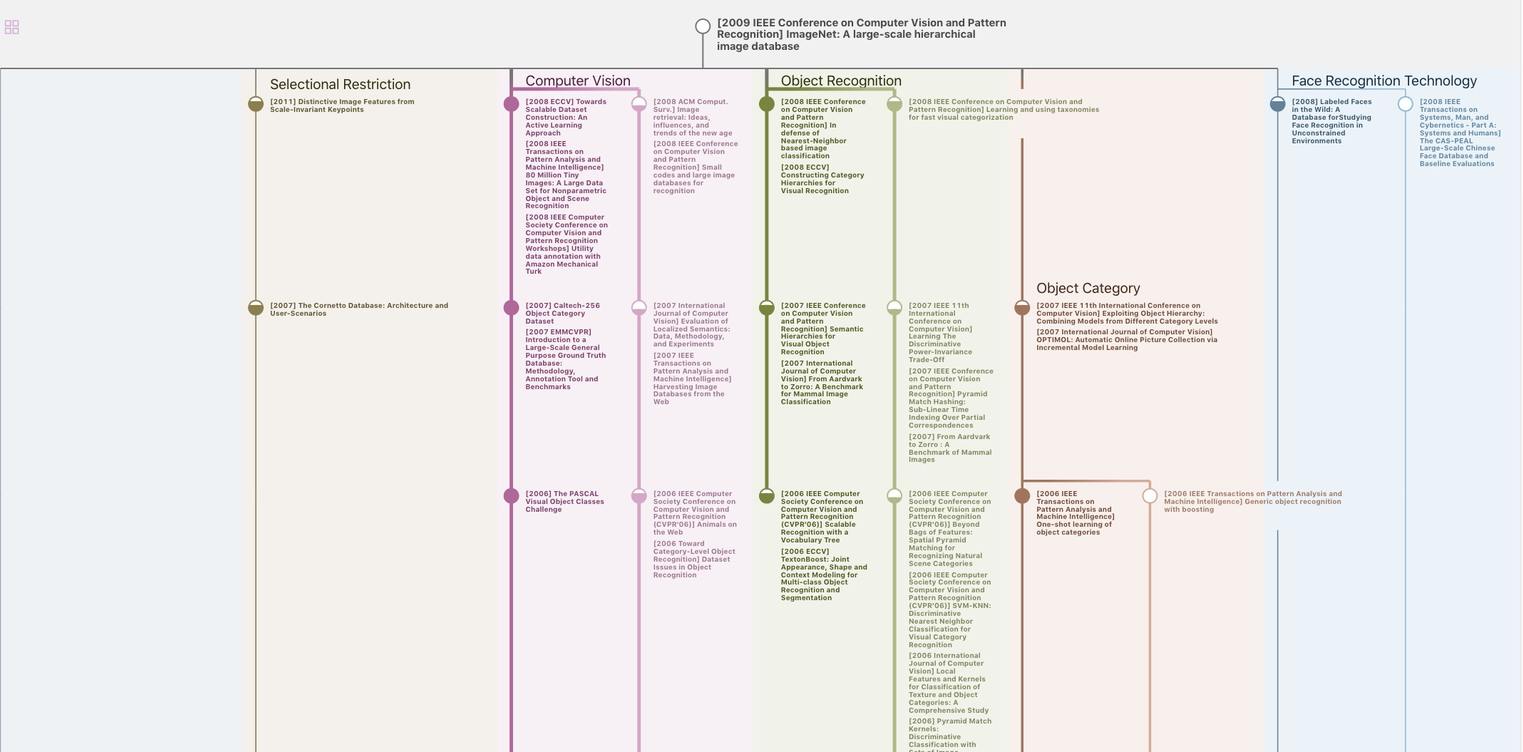
生成溯源树,研究论文发展脉络
Chat Paper
正在生成论文摘要