A Cybersecurity Framework For Classifying Non Stationary Data Streams Exploiting Genetic Programming And Ensemble Learning
NUMERICAL COMPUTATIONS: THEORY AND ALGORITHMS, PT I(2020)
摘要
Intrusion detection systems have to cope with many challenging problems, such as unbalanced datasets, fast data streams and frequent changes in the nature of the attacks (concept drift). To this aim, here, a distributed genetic programming (GP) tool is used to generate the combiner function of an ensemble; this tool does not need a heavy additional training phase, once the classifiers composing the ensemble have been trained, and it can hence answer quickly to concept drifts, also in the case of fast-changing data streams. The above-described approach is integrated into a novel cybersecurity framework for classifying non stationary and unbalanced data streams. The framework provides mechanisms for detecting drifts and for replacing classifiers, which permits to build the ensemble in an incremental way. Tests conducted on real data have shown that the framework is effective in both detecting attacks and reacting quickly to concept drifts.
更多查看译文
关键词
Cybersecurity, Intrusion detection, Genetic programming
AI 理解论文
溯源树
样例
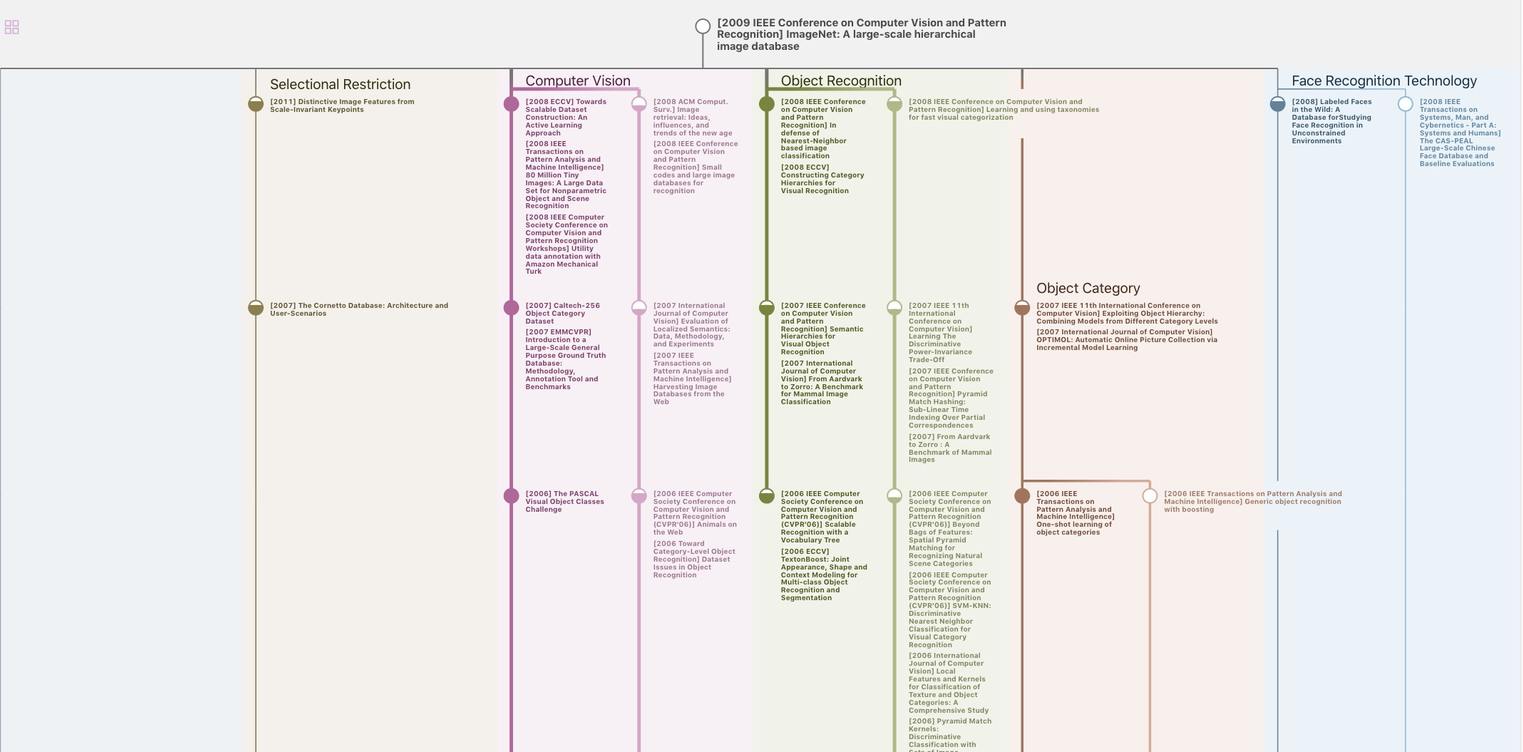
生成溯源树,研究论文发展脉络
Chat Paper
正在生成论文摘要