Multi-stream attentive generative adversarial network for dynamic scene deblurring.
Neurocomputing(2020)
摘要
Image deblurring methods based on deep learning have been proved to be effective for dynamic scene deblurring. Nevertheless, the most existing methods usually fail to deal with severe and complex dynamic scene blurs. In this paper, we propose a novel multi-stream attentive generative adversarial network (MSA-GAN) for solving the problems. Firstly, we design a novel attentive guidance module for generating an attention map which can guide to pay more attention on the severe blur regions or objects and their edge or texture structures in the feature extraction and deblurring process. Secondly, we propose the multi-stream and multi-scale feature extraction strategy and design an attentive and multi-scale feature extraction module, in which we design a multi-scale residual block for extracting the multi-scale features and capturing more information of complex blurs. Thirdly, we propose a multi-scale feature fusion strategy and the strategy is implemented in the multi-stream and multi-scale feature fusion module through the designed gate structure, which can adaptively learn and fuse multi-scale features from different streams. Finally, The extensive experiments are performed on the GoPro dynamic scene dataset and real image data, and the experimental results both quantitatively and qualitatively have demonstrated that the proposed method outperform the recent state-of-the-art methods.
更多查看译文
关键词
Attentive guidance module,Multi-stream,Attention map,Multi-scale fusion,Gate structure,Dynamic scene deblurring
AI 理解论文
溯源树
样例
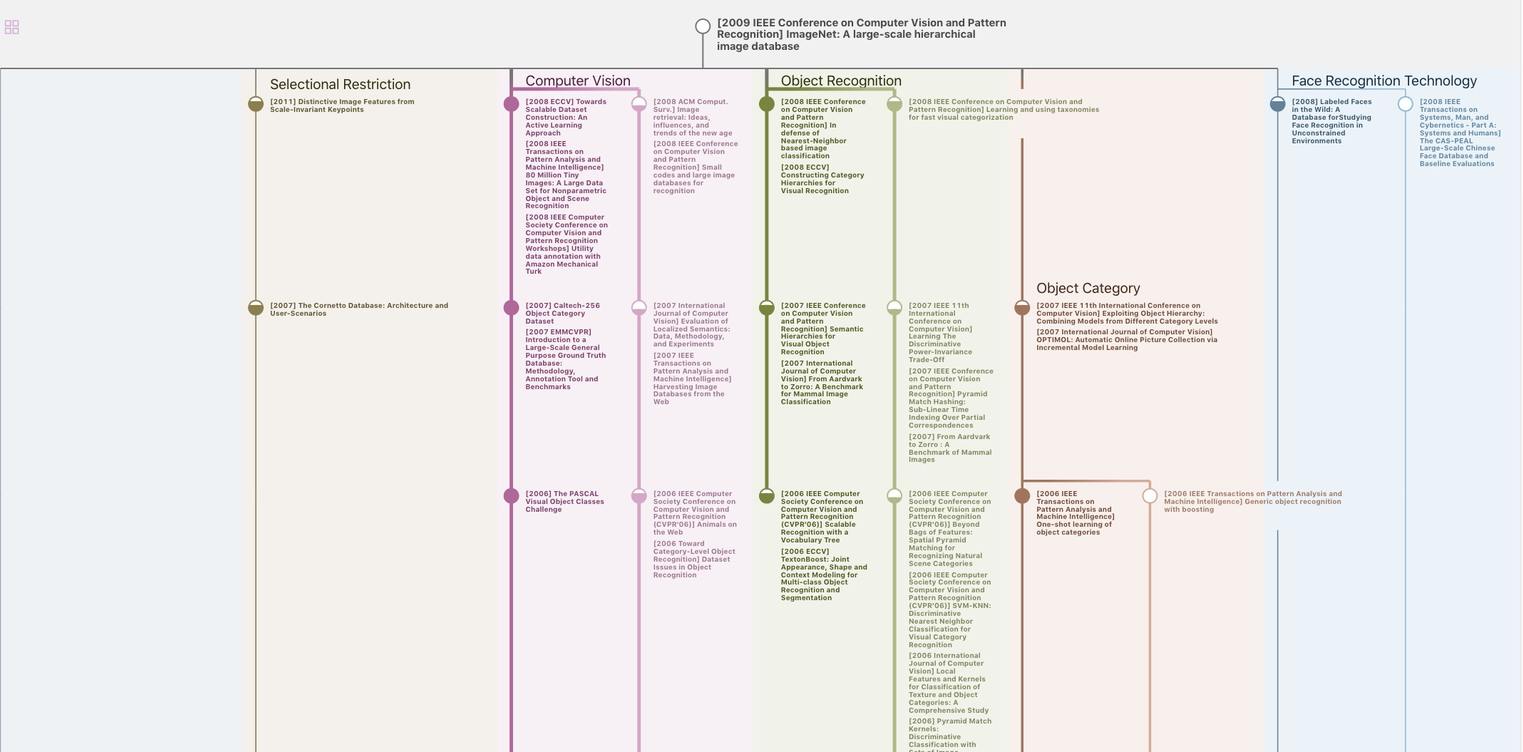
生成溯源树,研究论文发展脉络
Chat Paper
正在生成论文摘要