Improving aspect-based sentiment analysis via aligning aspect embedding.
Neurocomputing(2020)
摘要
Aspect-Based Sentiment Analysis (ABSA) is a fine-grained sentiment analysis task, which aims to predict sentiment polarities of given aspects or target terms in text. ABSA contains two subtasks: Aspect-Category Sentiment Analysis (ACSA) and Aspect-Term Sentiment Analysis (ATSA). Aspect embeddings have been extensively used for representing aspect-categories on ACSA task. Based on our observations, existing aspect embeddings cannot properly represent the relation between aspect-categories and aspect-terms. To address this limitation, this paper presents a learning method which trains aspect embeddings according to the relation between aspect-categories and aspect-terms. According to the cosine measure metric we proposed in this paper, the limitation is successfully alleviated in the aspect embeddings which are trained by our method. The trained aspect embeddings can be used as initialization in existing models to solve ACSA task. We conduct experiments on SemEval datasets for ACSA task, and the results indicate that our pre-trained aspect embeddings are capable of improving the performance of sentiment analysis.
更多查看译文
关键词
Aspect embedding,Sentiment analysis,Representation learning
AI 理解论文
溯源树
样例
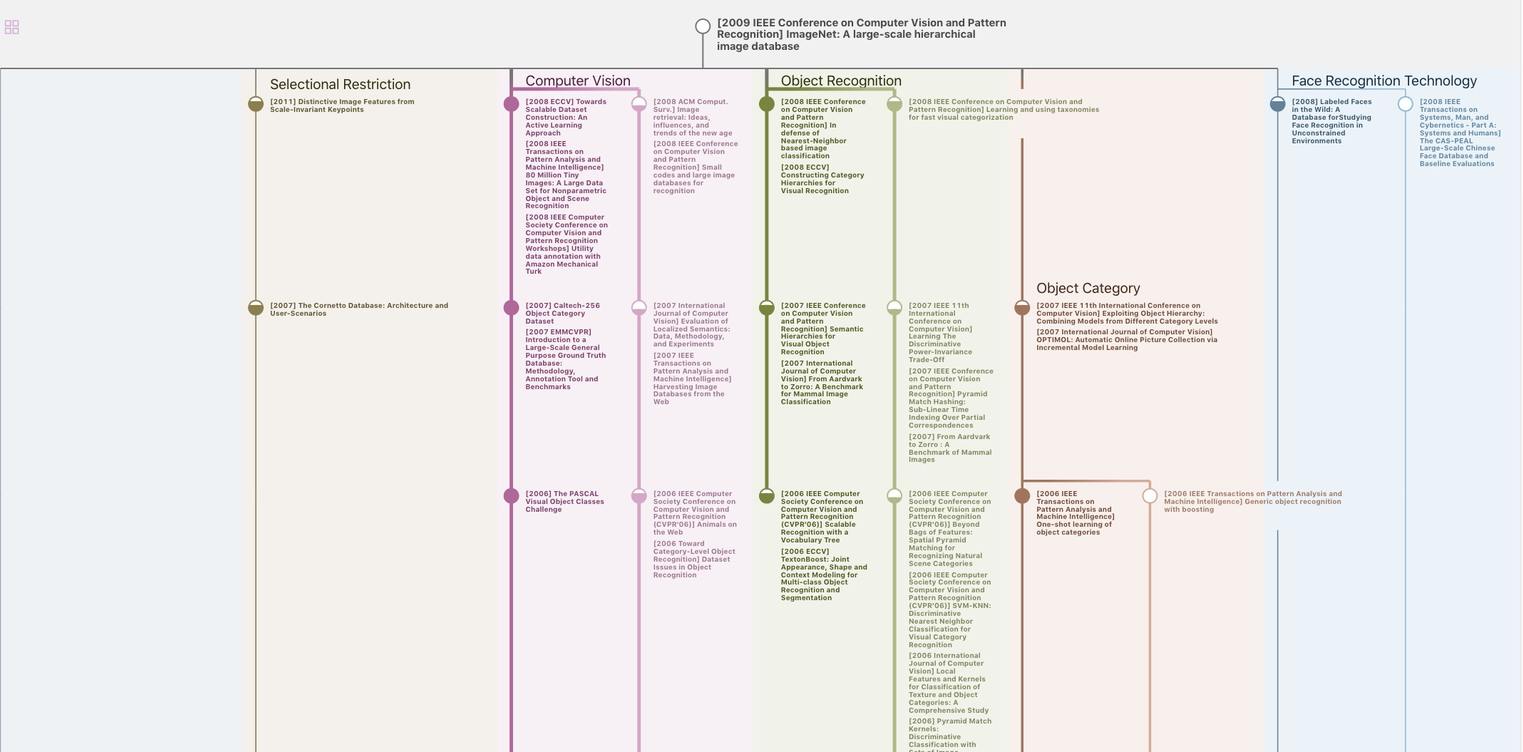
生成溯源树,研究论文发展脉络
Chat Paper
正在生成论文摘要