Multi-Step Model-Agnostic Meta-Learning: Convergence and Improved Algorithms
CoRR(2020)
Abstract
As a popular meta-learning approach, the model-agnostic meta-learning (MAML) algorithm has been widely used due to its simplicity and effectiveness. However, the convergence of the general multi-step MAML still remains unexplored. In this paper, we develop a new theoretical framework, under which we characterize the convergence rate and the computational complexity of multi-step MAML. Our results indicate that although the estimation bias and variance of the stochastic meta gradient involve exponential factors of $N$ (the number of the inner-stage gradient updates), MAML still attains the convergence with complexity increasing only linearly with $N$ with a properly chosen inner stepsize. We then take a further step to develop a more efficient Hessian-free MAML. We first show that the existing zeroth-order Hessian estimator contains a constant-level estimation error so that the MAML algorithm can perform unstably. To address this issue, we propose a novel Hessian estimator via a gradient-based Gaussian smoothing method, and show that it achieves a much smaller estimation bias and variance, and the resulting algorithm achieves the same performance guarantee as the original MAML under mild conditions. Our experiments validate our theory and demonstrate the effectiveness of the proposed Hessian estimator.
MoreTranslated text
Key words
algorithms,multi-step,model-agnostic,meta-learning
AI Read Science
Must-Reading Tree
Example
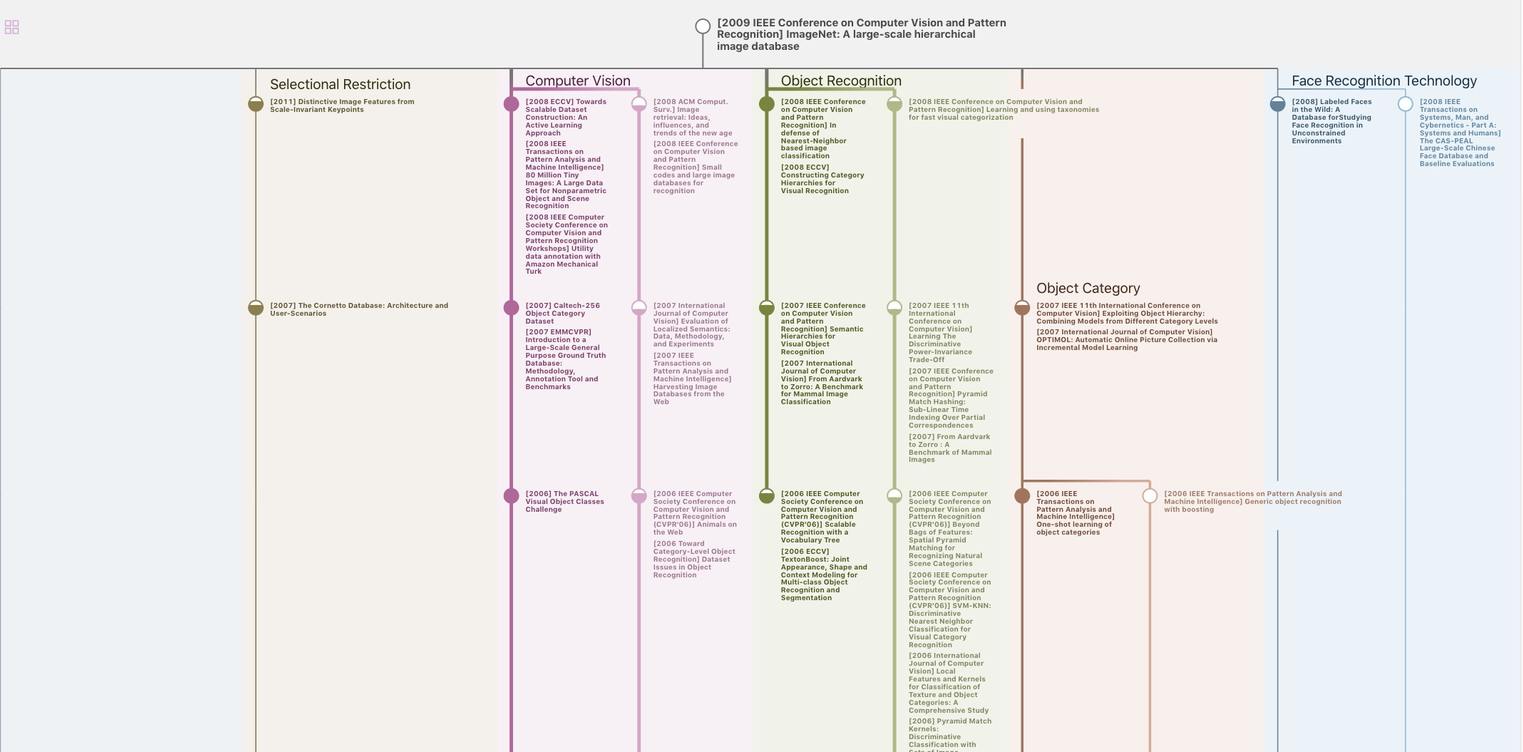
Generate MRT to find the research sequence of this paper
Chat Paper
Summary is being generated by the instructions you defined