Interpreting Interpretations: Organizing Attribution Methods by Criteria
2020 IEEE/CVF Conference on Computer Vision and Pattern Recognition Workshops (CVPRW)(2020)
摘要
Motivated by distinct, though related, criteria, a growing number of attribution methods have been developed to interprete deep learning. While each relies on the interpretability of the concept of "importance" and our ability to visualize patterns, explanations produced by the methods often differ. In this work we expand the foundations of human-understandable concepts with which attributions can be interpreted beyond "importance" and its visualization; we incorporate the logical concepts of necessity and sufficiency, and the concept of proportionality. We define metrics to represent these concepts as quantitative aspects of an attribution. We evaluate our measures on a collection of methods explaining convolutional neural networks (CNN) for image classification. We conclude that some attribution methods are more appropriate for interpretation in terms of necessity while others are in terms of sufficiency, while no method is always the most appropriate in terms of both.
更多查看译文
关键词
image classification,CNN,convolutional neural networks,logical concepts,human-understandable concepts,interprete deep learning,organizing attribution methods
AI 理解论文
溯源树
样例
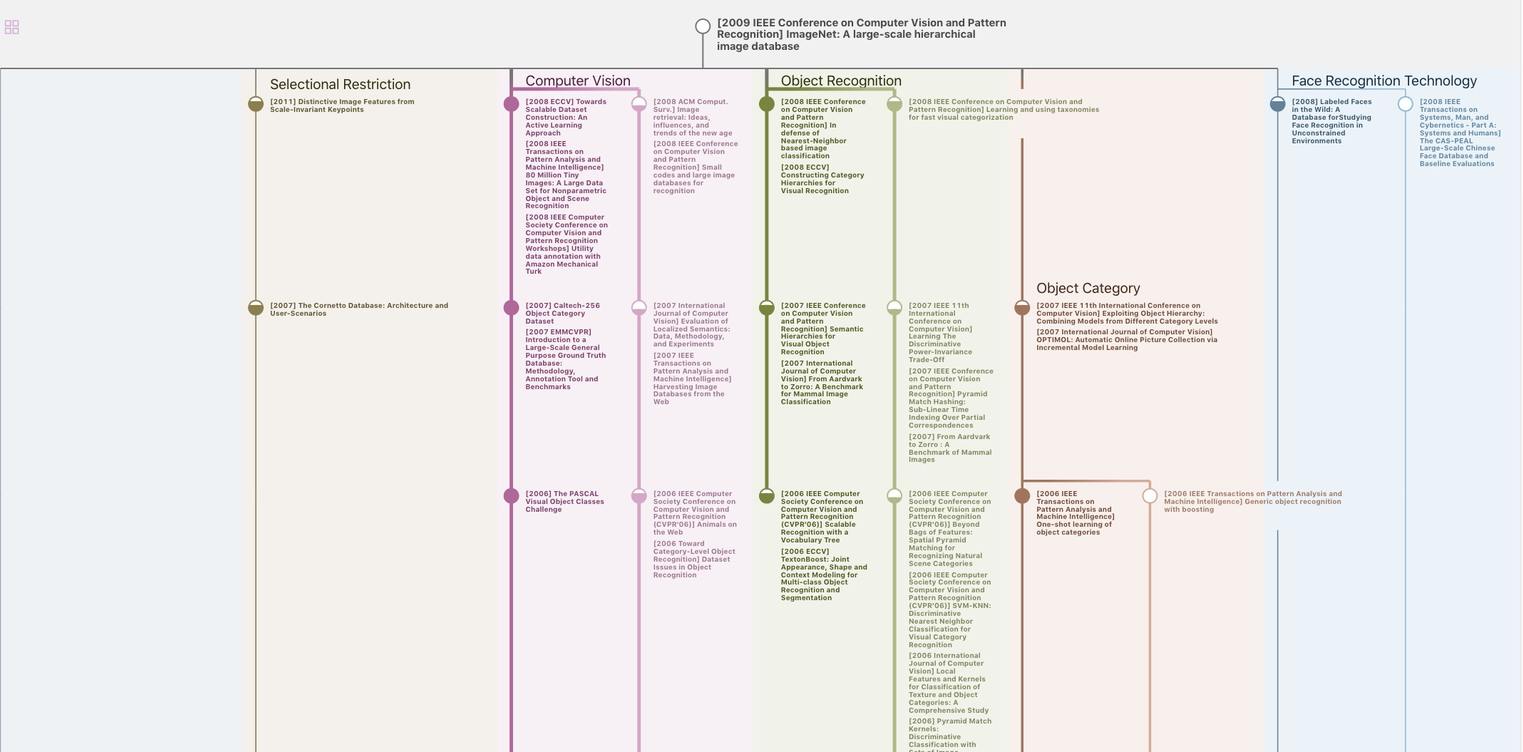
生成溯源树,研究论文发展脉络
Chat Paper
正在生成论文摘要