CoLES: Contrastive Learning for Event Sequences with Self-Supervision
PROCEEDINGS OF THE 2022 INTERNATIONAL CONFERENCE ON MANAGEMENT OF DATA (SIGMOD '22)(2022)
摘要
We address the problem of self-supervised learning on discrete event sequences generated by real-world users. Self-supervised learning incorporates complex information from the raw data in low-dimensional fixed-length vector representations that could be easily applied in various downstream machine learning tasks. In this paper, we propose a new method "CoLES", which adapts contrastive learning, previously used for audio and computer vision domains, to the discrete event sequences domain in a self-supervised setting. We deployed CoLES embeddings based on sequences of transactions at the large European financial services company. Usage of CoLES embeddings significantly improves the performance of the pre-existing models on downstream tasks and produces significant financial gains, measured in hundreds of millions of dollars yearly. We also evaluated CoLES on several public event sequences datasets and showed that CoLES representations consistently outperform other methods on different downstream tasks.
更多查看译文
关键词
representation learning, metric learning, contrastive learning, self-supervised learning, event sequences, data management
AI 理解论文
溯源树
样例
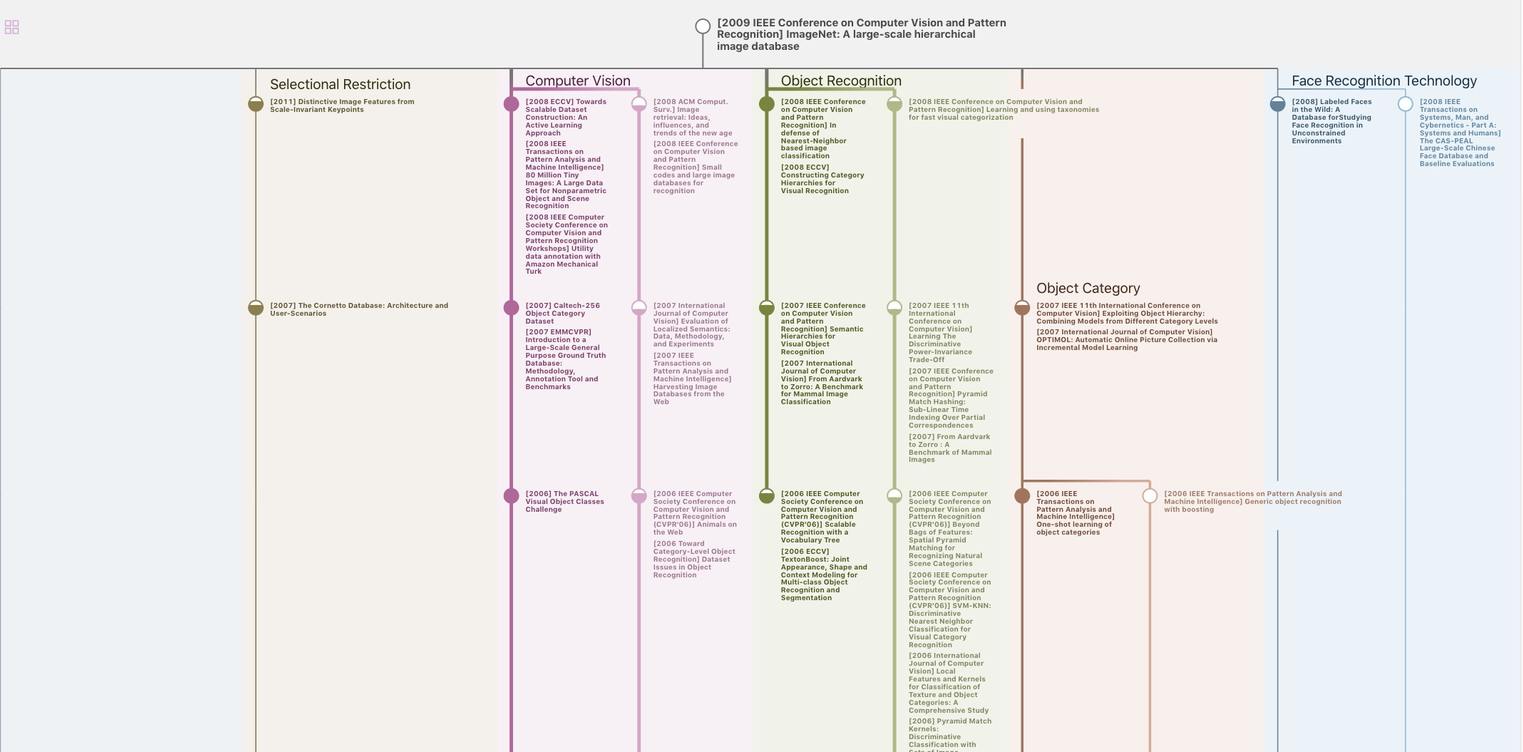
生成溯源树,研究论文发展脉络
Chat Paper
正在生成论文摘要