Live Semantic 3D Perception for Immersive Augmented Reality.
IEEE transactions on visualization and computer graphics(2020)
摘要
Semantic understanding of 3D environments is critical for both the unmanned system and the human involved virtual/augmented reality (VR/AR) immersive experience. Spatially-sparse convolution, taking advantage of the intrinsic sparsity of 3D point cloud data, makes high resolution 3D convolutional neural networks tractable with state-of-the-art results on 3D semantic segmentation problems. However, the exhaustive computations limits the practical usage of semantic 3D perception for VR/AR applications in portable devices. In this paper, we identify that the efficiency bottleneck lies in the unorganized memory access of the sparse convolution steps, i.e., the points are stored independently based on a predefined dictionary, which is inefficient due to the limited memory bandwidth of parallel computing devices (GPU). With the insight that points are continuous as 2D surfaces in 3D space, a chunk-based sparse convolution scheme is proposed to reuse the neighboring points within each spatially organized chunk. An efficient multi-layer adaptive fusion module is further proposed for employing the spatial consistency cue of 3D data to further reduce the computational burden. Quantitative experiments on public datasets demonstrate that our approach works 11× faster than previous approaches with competitive accuracy. By implementing both semantic and geometric 3D reconstruction simultaneously on a portable tablet device, we demo a foundation platform for immersive AR applications.
更多查看译文
关键词
Three-dimensional displays,Convolution,Semantics,Two dimensional displays,Image segmentation,Graphics processing units,Solid modeling,Dense 3D Reconstruction,3D Semantic Segmentation,3D Convolutional Network,Virtual Reality,Augmented Reality
AI 理解论文
溯源树
样例
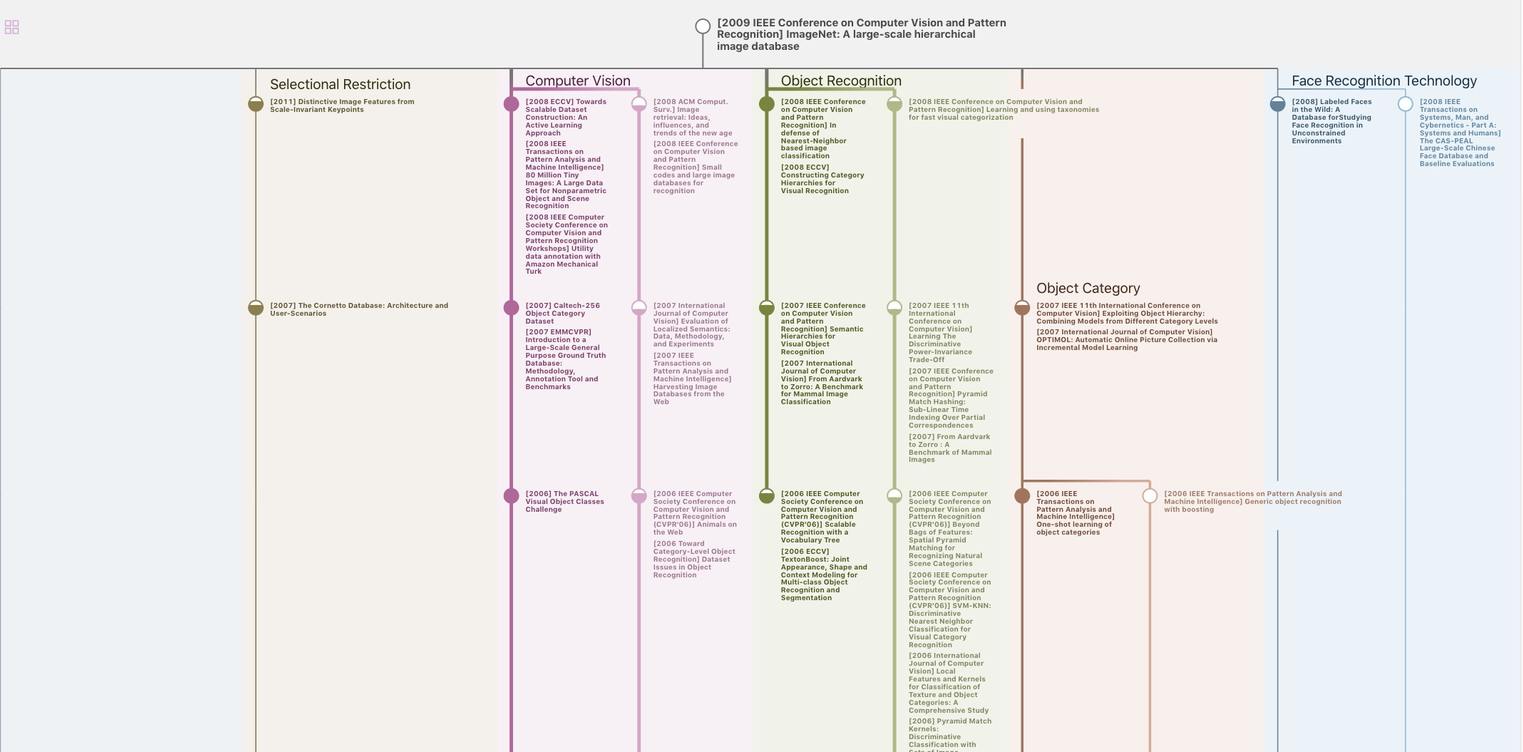
生成溯源树,研究论文发展脉络
Chat Paper
正在生成论文摘要