Improved Approximate Degree Bounds for K-Distinctness.
Electronic Colloquium on Computational Complexity (ECCC)(2020)
Abstract
An open problem that is widely regarded as one of the most important in quantum query complexity is to resolve the quantum query complexity of the k-distinctness function on inputs of size N. While the case of k=2 (also called Element Distinctness) is well-understood, there is a polynomial gap between the known upper and lower bounds for all constants k>2. Specifically, the best known upper bound is O(N^{(3/4)-1/(2^{k+2}-4)}) (Belovs, FOCS 2012), while the best known lower bound for k >= 2 is Omega(N^{2/3} + N^{(3/4)-1/(2k)}) (Aaronson and Shi, J.~ACM 2004; Bun, Kothari, and Thaler, STOC 2018). For any constant k >= 4, we improve the lower bound to Omega(N^{(3/4)-1/(4k)}). This yields, for example, the first proof that 4-distinctness is strictly harder than Element Distinctness. Our lower bound applies more generally to approximate degree. As a secondary result, we give a simple construction of an approximating polynomial of degree O(N^{3/4}) that applies whenever k <= polylog(N).
MoreTranslated text
Key words
Approximation Algorithms,Quantum Error Correction
PDF
View via Publisher
AI Read Science
AI Summary
AI Summary is the key point extracted automatically understanding the full text of the paper, including the background, methods, results, conclusions, icons and other key content, so that you can get the outline of the paper at a glance.
Example
Background
Key content
Introduction
Methods
Results
Related work
Fund
Key content
- Pretraining has recently greatly promoted the development of natural language processing (NLP)
- We show that M6 outperforms the baselines in multimodal downstream tasks, and the large M6 with 10 parameters can reach a better performance
- We propose a method called M6 that is able to process information of multiple modalities and perform both single-modal and cross-modal understanding and generation
- The model is scaled to large model with 10 billion parameters with sophisticated deployment, and the 10 -parameter M6-large is the largest pretrained model in Chinese
- Experimental results show that our proposed M6 outperforms the baseline in a number of downstream tasks concerning both single modality and multiple modalities We will continue the pretraining of extremely large models by increasing data to explore the limit of its performance
Try using models to generate summary,it takes about 60s
Must-Reading Tree
Example
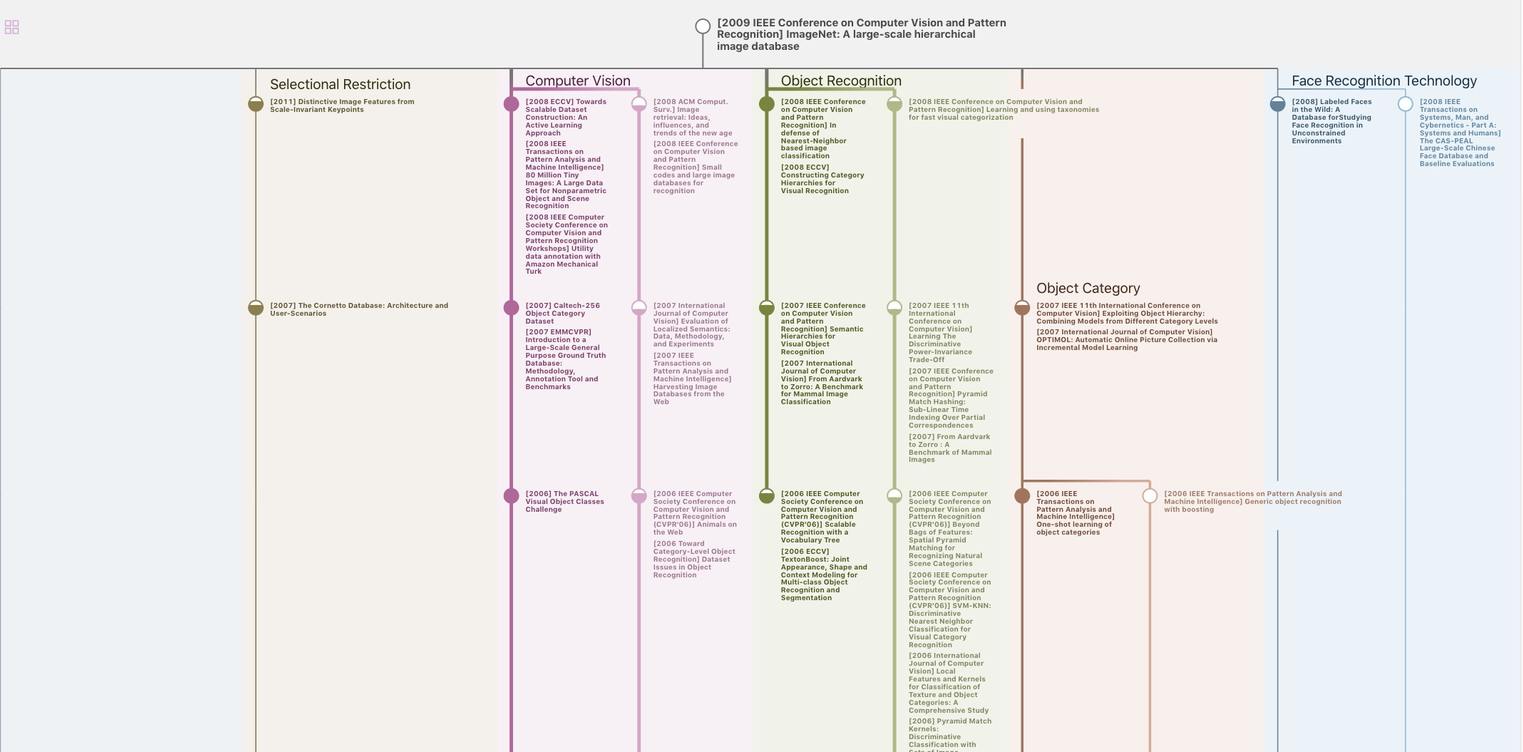
Generate MRT to find the research sequence of this paper
Related Papers
2003
被引用131 | 浏览
Data Disclaimer
The page data are from open Internet sources, cooperative publishers and automatic analysis results through AI technology. We do not make any commitments and guarantees for the validity, accuracy, correctness, reliability, completeness and timeliness of the page data. If you have any questions, please contact us by email: report@aminer.cn
Chat Paper
去 AI 文献库 对话