Learning Gaussian Graphical Models via Multiplicative Weights
INTERNATIONAL CONFERENCE ON ARTIFICIAL INTELLIGENCE AND STATISTICS, VOL 108(2020)
摘要
Graphical model selection in Markov random fields is a fundamental problem in statistics and machine learning. Two particularly prominent models, the Ising model and Gaussian model, have largely developed in parallel using different (though often related) techniques, and several practical algorithms with rigorous sample complexity bounds have been established for each. In this paper, we adapt a recently proposed algorithm of Klivans and Meka (FOCS, 2017), based on the method of multiplicative weight updates, from the Ising model to the Gaussian model, via non-trivial modifications to both the algorithm and its analysis. The algorithm enjoys a sample complexity bound that is qualitatively similar to others in the literature, has a low runtime $O(mp^2)$ in the case of $m$ samples and $p$ nodes, and can trivially be implemented in an online manner.
更多查看译文
关键词
gaussian graphical models,weights,learning
AI 理解论文
溯源树
样例
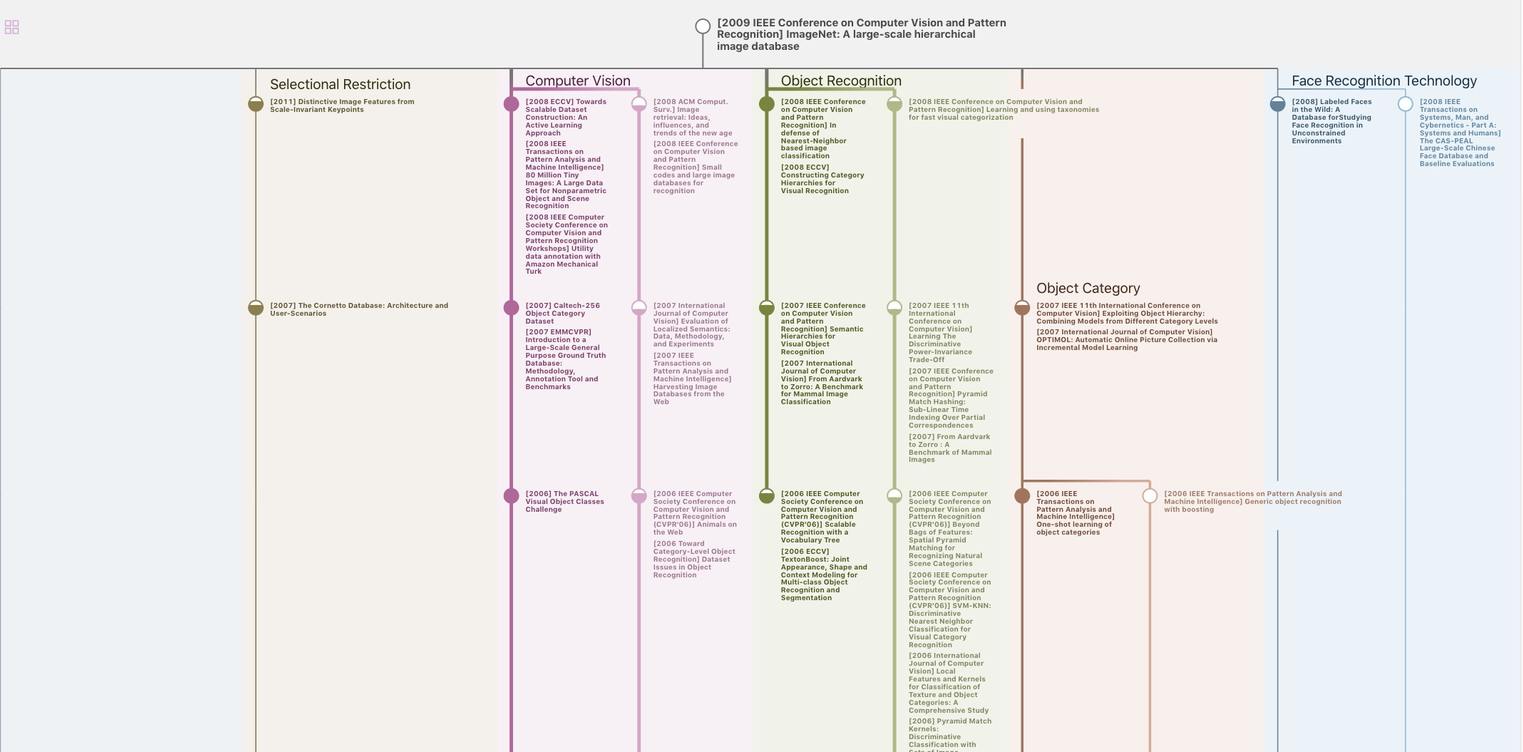
生成溯源树,研究论文发展脉络
Chat Paper
正在生成论文摘要